What To Expect From Artificial Intelligence In Pharma — And How To Get There
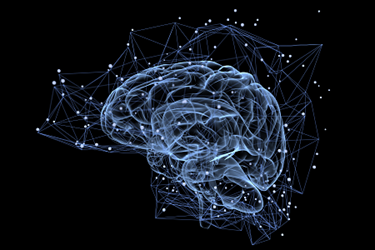
Artificial intelligence (AI) has truly moved from concept to reality in the pharmaceutical industry. Many companies are already using AI to pour through mountains of scientific data in an effort to speed and improve the drug discovery process. And the technology is starting to find new applications in areas as diverse as regulatory/compliance, clinical trials, manufacturing, and supply chain.
For an update on AI and its adoption in the industry, Pharmaceutical Online turned to three speakers from Xavier Health’s upcoming AI Summit:
- Anthony Barnes, chief science officer and co-founder, Bionometrix
- Matthew Schmucki, senior engineer, AstraZeneca
- Marla Phillips, director, Xavier Health, Xavier University
Below, these experts answer a series of questions regarding specific AI case studies, implementation best practices, regulatory considerations, and the technology’s future prospects in the industry.
Please provide a quick introduction to artificial intelligence (AI). What is it exactly, and how can it help businesses become more efficient?
Anthony Barnes, Bionometrix: I try to boil it down to a simple definition of intelligence. My working definition is that intelligence is the use of past experiences or data to predict the future in a way that benefits you. The nature of these predictions can range from reaching for a door handle while carrying a load of towels in your arms, to trying to predict the behavior of the North Korean military. Human intelligence is built upon analogic thought, while more artificial intelligence is based upon datasets of multiple experiences.
There are actually layers of intelligence, from predicting an individual concept of event to the idea of reasoning how a set of individual predictions lead to an “understanding” of a situation. The big kahuna of intelligence is a knowledge graph that links entire environments together such that one actually has a mastery of a given situation and can predict outcomes driven by any change. This mastery of understanding is called expertise.
In what ways is AI currently being used in the bio/pharma industry?
Barnes: A lot of the present use of artificial intelligence is found in drug discovery and drug development, as one tries to predict the efficacy and safety (and a set of other variables) of a given molecular form in a given biological system.
Other AI tools are used to predict the appropriateness of a given patient for a clinical trial, by using any number of criteria and different datasets from medical records to physical examination to radiology work-up.
There are many fine-tuned applications evolving that apply AI across the pharmaceutical business model. For instance, precision medicine and predictive medicine are applications where treatment protocols are predicted for a given patient, rather than for a group of patients. Most new medical diagnostics and radiology applications are read using some form of machine learning, often involving complex mathematical concepts and forms of deep learning.
As for the business aspects of pharmaceuticals, the industry is susceptible to many different applications, from treasury management to customer service using bots to proper adverse event detection and reporting directly to the FDA.
Cross-company quality assurance is an interesting application, as companies are used to using specifications to release products. Historically, these have been fairly clumsy devices, where bands of acceptability are agreed to with regulatory agencies. New structures are emerging to understand better how small variations actually predict market performance in different subpopulations.
Can you share any specific examples of successful AI use at pharma companies?
Matthew Schmucki: BERG, a pharmaceutical startup, has created an AI program that takes in biological data as cells transform from healthy to cancerous. The software utilizes information from the 2003 Human Genome Project in addition to the over 14 trillion data points in a single cell tissue. This research allowed BERG to develop a new cancer drug that could potentially reverse this process from healthy to cancerous.
Insilico Medicine, a bioinformatics company, has used AI to predict the therapeutic area of new drugs before the drug is tested. The program takes in a large amount of data from human cells exposed to current drugs and can compare the results to current developmental drugs. Often, the program identifies secondary therapeutic areas that researchers did not initially consider.
What are the key factors consider when evaluating AI for a specific function/process?
Schmucki: When considering AI, identify a problem statement that describes the key inputs and expected outputs. Look at what data is available for the AI to use. There needs to be large datasets with clean data for AI to be effective. If the data is not clean, you may spend more time straightening the data than using it.
It is also important to consider the end-user. Is it a group of users or a single user? Is it an experience programmer or someone who will need some type of graphical user interface (GUI) to interact with the solution? What will the output look like? These are all items that should be clearly documented in some type of charter to stay on task and avoid scope creep.
Who needs to be involved in AI-related discussions and decision making?
Schmucki: Support from leadership is necessary to implement any type of large project, so starting at the top is always a good place. The end user needs to be involved as well. You also need the actual data scientist or programmer who will be developing the solution. In pharma specifically, there may need to be an understanding from quality or validation depending on the intended use of the solution.
What are the initial steps in implementing an AI solution?
Barnes: Implementing an AI solution normally means some form of predictive analytics project where there is either diffuse “big data” or expensive “good data” that has been culled from the company’s experience. As with all issues, knowing what question you want to answer is the most important issue to be solved, and this is not easy, especially in highly functional organizations. Once these two aspects (data available and the question one wants insight into), then the process of turning this into some form of useful association, correlation, or predictive model is the burden. Also, finding some way to build an “evergreen model” that allows adaptation with changes in the model, the environment, or both is a challenge. The biggest issue is knowing when a predictive model stops predicting as expected and figuring out why. It is kind of like when the “check engine” light comes on in your car. At best it is a fault in the sensor; at worst it is a cracked engine block.
Where do regulatory bodies — particularly FDA — stand regarding AI use?
Marla Phillips, Xavier University: The FDA has been leading initiatives with the pharmaceutical, biological, and medical device industries to try to (1) increase the ownership of product quality to the industry through the Center for Devices and Radiological Health (CDRH) “Case for Quality”, (2) identify proactive signals of risk to product quality through the Center for Drug Evaluation and Research (CDER) “Call for Metrics”, (3) reduce the risk of drug shortages, and (4) utilize more holistic information during product approval that would allow FDA to grant a “pre-certification” review process that is similar to the Transportation Security Administration (TSA) pre-check. Artificial intelligence can increase the dataset of relevant internal and external information to support these and other goals of the FDA. As a result, several FDA officials are noting the power of AI but, as with industry, are exploring the possibilities, obstacles, and areas that are still not quite understood. FDA’s Bakul Patel, for example, is exploring the power of artificial intelligence in the newly formed Digital Health Unit that reports directly to the CDRH center director, Jeff Shuren. Through the Xavier Artificial Intelligence Initiative, FDA and industry members will form a team to explore how AI can be used to continuously assess product quality, which could enable industry to move from the proactive measures afforded by metrics to the predictive capabilities of artificial intelligence.
Additionally, industry is already using the power of artificial intelligence to identify possible early signals of clinical trial failure, as well as in a diagnostic capacity. The outcome of this work is then used for R&D, followed by product development through typical industry methodologies, so this does not appear to affect the purview of FDA.
What’s next for AI in the pharma industry?
Schmucki: As AI matures, it could change our healthcare’s diagnostic system. It may become easier to take a cell sample from a patient and run it through an automated list of treatments with the highest success rates. The AI would be able to review medical journals as they are published around the world and always be aware of the latest studies. For a smaller practice with only one or two doctors, this could completely change the diagnosis and treatments. This small practice now has instant access to and analysis of the world’s medical data and treatment options.
Phillips: There remains a tremendous amount of potential to use AI to detect therapeutic targets such that researchers can develop pathways to treat and cure currently untreated and terminal diseases. This is very exciting for the future health of each one of us! We are seeing AI make its way into supply chain operations, and through expansion beyond “Big Data”, helping to optimize manufacturing operations. I personally believe AI can help FDA pull together vast information on each company to successfully establish maturity models such that more robust risk comparisons can be made in an effort to most appropriately deploy FDA investigator resources. I also believe the power of AI can be used to significantly augment release decisions and internal/external audits. By continuously scanning documents and data, AI can give a real-time reflection of risk to product quality, and can put that information in the hands of the people making decisions of whether to release product to the market or not.
We will be exploring these future possibilities and more through the Xavier Artificial Intelligence Initiative, where we have engaged FDA officials, pharmaceutical professionals, medical device professionals, service providers and AI experts. I believe we are poised to truly make a difference.
Barnes: Since the individual assets are so valuable (e.g., Humira), and since regulation is so heavy in the industry, having a light-footed, predictive analytics effort is hard. Everything from marketing messaging to drug component release is carefully managed, and changes need several levels of management response because no one wants to muck up the golden goose. In many ways, pharma needs automated, close-in prediction as a way to hone the control of systems more than it needs AI to allow rapid market pivots with respect to market messaging. Sometimes pricing can be managed very adaptively, but again, rapid response to market characteristics can lead to troubling recrimination. There is a reason why pharma is normally a top-down management situation, as the decisions are normally big decisions, often with no way to reverse course. In most drug companies, the system is built to deliver decision support information up the ladder to help senior managers make decisions and apply resources.
Another interesting area to consider is the use of deep learning to predict in-market observations of a drug’s performance in an expanded clinical population, where comorbidities and predispositions and subtle biological and environmental issues can be detected with enough power to truly understand what is happening at the total market level. As deep learning can often detect subtle conditional information that often does not have a label until studied, there is a whole level of population perturbed information that can lead to unique understandings of how a class of drug or a dosing pattern leads to differential population effects that might be lasting across certain subsets. We really do not understand the subtle effects and interactions found in drug populations, and there is very little incentive for companies to document these, though there are big reasons for the population in general to intelligently understand these effects. This difference in public interest versus private interest needs to be broached carefully, as we sometimes give up very good drugs because we do not yet have sensitive mechanisms to keep the baby while throwing out the bathwater. Big Data and AI might allow intelligent use of drugs that are probably not useful when simply released for all comers.