Next Steps For AI, Machine Learning In Biopharma

By Matthew Pillar, Editor, Bioprocess Online
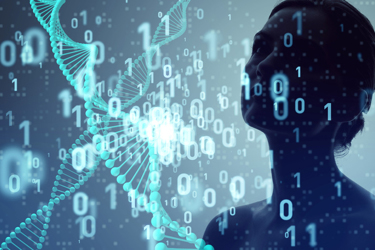
A common thread is emerging among new biopharma companies; they’re hiring as many data scientists as they are wet lab scientists and their data server budgets are growing faster than that of their protein purification processes. Here’s what that means to you and your company.
Bill Gates isn’t the only tech industry insider turned life sciences “outsider” currently throwing his weight around the biosciences. There’s a new breed of biopharma leader on the rise, an ilk identified not by their lab coats but by their pocket protectors, and not by their hair nets but by their propeller heads. The market worth of data scientists and digital transformation specialists is rapidly appreciating as biopharma firms seek to explore how harnessing data and appropriately processing it will help them work faster and smarter, from drug discovery to clinical trials and beyond. The growing number of tech and data science professionals in the field are increasingly being tasked with the exploration and implementation of artificial intelligence (AI) applications specific to the business of biopharma – and many of those tech and data-oriented professionals are riding that skillset straight to the top. Here’s why AI matters so much in biopharma right now, and why it’s central to the success of your business –and even your career.
What’s AI?
Merriam-Webster defines Artificial Intelligence as “a branch of computer science dealing with the simulation of intelligent behavior in computers,” and “the capability of a machine to imitate intelligent human behavior.” Similarly, Oxford Languages defines it as “the theory and development of computer systems able to perform tasks that normally require human intelligence, such as visual perception, speech recognition, decision-making, and translation between languages.”
Those capabilities typically take shape as an algorithm—a set of rules to be followed when processing inputs—written into a computer software application.
Physical footprint aside, these definitions miss the important point that with today’s inexpensive supercomputing capacity and an ever-expanding dataset, AI facilitates human-style intelligence on a scale and speed incomprehensible to human beings.
In biopharma, drug discovery is the current killer application for AI, though it’s being called in to action to improve myriad tasks and processes. MarketsandMarkets, which pegs the AI market in biopharma alone at $3.88 billion by 2025 on 52.9 percent CAGR, says precision medicine, medical imaging, and diagnostics/research round out the top four applications for the technology in the biopharma space.
Why Does AI Matter To Biopharma?
Speed and efficiency are arguably the most important differentiating characteristics of winning biopharma companies. In an industry that accepts varying degrees of failure as a given, those who fail the fastest and the cheapest are often among the front runners. The ability of artificial intelligence algorithms to apply the sophisticated nuance of your people-driven discovery, development, or clinical operations at 10X or 100X the scale ensures you discover failures fast and at minimal expense. Fast failure is a concept not celebrated enough.
Of course, in discovery there’s even more value in the speed of success. Agile, data-driven emerging biopharmas are disrupting drug discovery protocols by processing giant data sets in the search for “hits” worthy of further exploration. How much data are we talking? The world’s library of genomics data grew from a terabyte in 2000 to more than 20 petabytes today on its way to an estimated 40 exabytes by 2025. For reference, the data backup company Backblaze says at an average data exchange rate of 20 Megabytes per hour, an office of 100 people would have to browse the Internet nonstop for 57,077 years to reach ONE exabyte of data.
Multiple sources have pegged the average patient’s medical records at 80 megabytes per year. I won’t attempt to extrapolate a figure for the amount of national or global EMR data generated annually. The point is that as these data sets grow, so does their potential to paint an increasingly detailed portrait of the patient population’s genetic composition, treatment history, and treatment efficacy. That, in turn, serves to build the value of tools we can use to leverage that data to do things like:
- Discover new therapeutic candidates
- Understand market trends, demands, and unmet medical needs to direct pipeline decisions
- Align specific treatments with specific patients based on genomic data
- Explore and better understand patient populations for clinical trial recruitment
- Ultimately, more efficiently achieve better patient outcomes.
We can’t possibly extract the value of all this data manually.
What’s Difficult About Leveraging AI In Biopharma?
Clearly, the data is there for the taking, and its volumes are growing exponentially. The current challenge lies in developing purpose-specific tools to process it all. Chances are, there’s not an off-the-shelf app available for the data experiments you want to conduct. You’re not going to buy a plug & play drug discovery download from Oracle and wake up to a hit list of potential matches to send to the wet lab tomorrow morning.
Yet, it’s algorithmic software that’s responsible for the intelligence in AI. Those algorithms, written by a human, execute the host of intricate data computations that mimic human problem solving. Importantly, the software logs its findings and applies what it’s stored to continually refine the algorithm as it contemplates new data, with minimal intervention. That’s what we refer to as machine learning.
At this point, most data science minded biopharmas that are leveraging artificial intelligence and machine learning are growing their own applications using either open-source AI software development tools like Google’s TensorFlow, Amazon’s SageMaker Neo (AWS), and Microsoft’s Cognitive Toolkit, or they’re developing on proprietary tools from companies like IBM and Oracle.
What Should You Do Now?
Start with the right people. Agile, AI-enabled biopharma companies like Fountain Therapeutics and NeuBase are processing big data on applications designed and built by in-house programmers and data scientists, whose numbers run parallel to the biologists they both employ. Says NeuBase chief Dr. Dietrich Stephan, “There’s a compute component to actually identifying a mutant sequence you want to target, and then designing these drugs … Once we have a series of drugs that we've screened with activity data and cytotoxicity data, we apply machine learning to understand the attributes of those drugs and allow us to engineer out the off-target effects to give it the performance we want.”
At Fountain, CEO John Dimos says a combination of cloud-based AWS processing power and on-premises servers are screening drugs 24/7 at a rate of 50,000 compounds per month, which has huge potential implications on the efficiencies of early-stage drug development. “We’re gearing up for the readout of animal studies at the end of this year, and we’re really looking forward to being able to prioritize some of those hits and selecting clinical candidates to move forward in 2021,” he says.
To get to these levels of output, both leaders placed an early emphasis on hiring data scientists and programmers with keen interest in drug development and proceeded to thoroughly integrate those employees with those in the wet lab. No need, however, for you science types to worry that the algorithms those programmers write will code you right out of a career. While a recent study from McKinsey warned that automation will eliminate some 800 million jobs by 2030, biology isn’t one of them. Biologists and related scientists, the report says, face only a 15.6 percent risk of being replaced by automation.
AI and machine learning have cemented their places in the biopharma industry. Bioinformatics and computational biology are now degree programs at several schools, and even before that became the case, there was evidence of the value of information science in biopharma leadership. During the 15 years he worked at Roche before becoming CEO, Severin Schwan worked in informatics. Spark Therapeutics Founder & CEO Jeffrey D. Marrazzo studied systems science & engineering, a close cousin to Information Systems, at UPenn. As biopharma and high tech continue to intersect, the industry becomes more influenced and underwritten by Silicon Valley, which has produced tech leaders-turned-biopharma CEOs like Aldo Carrascoso (high-throughput glycoproteomics, InterVenn), Marc Stapley (population genomics, Helix), Eric Lefkofsky (precision medicine, Tempus), and many, many more. As these leaders move forward with visionary applications for AI and machine learning, the entire industry and the patients it serves are poised to make great progress.