Why It's Computational Biology's Time To Shine

By Matthew Pillar, Editor, Bioprocess Online
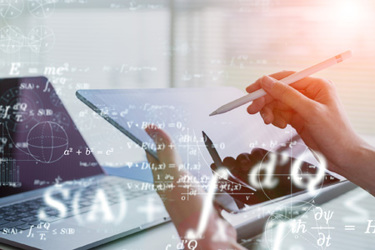
If you’re super familiar with the cost and time required to take a biologic drug from discovery all the way to market, go ahead and skip to the next paragraph. You don’t need the context I’m about to provide as I build a case for why the computational biology space is about to blow up. You already know that, according to the trade group Pharmaceutical Research and Manufacturers of America (PhRMA), it takes an average of 10 years to take a biologic from discovery to market. And you know that among 10 recent cancer biologic approvals, according to the Journal of the American Medical Association, the average cost of development rang in at $1 billion. You also know just how risky it is to dedicate that kind of time and money to biologic therapy development. The overall probability of clinical success, again per PhRMA, is estimated at less than 12%.
Imagine for a minute that you’re not a biopharmaceutical professional, but instead you’re a professional widget maker. You’ve worked for big widget makers most of your career, you know the widget business like the back of your hand, and you’ve got the itch to launch a widget company of your own. You put together a business plan, hit the venture capital circuit, and the conversation you engage in three to four times per week for the next six months goes something like this:
You: “I’m going to need a billion dollars, give or take a few million, over ten years.”
VC: “Wow. That’s a lot of money. At what point over those ten years do you anticipate revenue generation?”
You: “Year 11.”
VC: “Year 11? Why not year two? Why not year three?”
You: “It will take a few years to build the machinery required to build the widget. It will take a few more years to design the widget so it doesn’t break, or worse, break its user. Then it will take a few more years to rebuild the machinery required to produce the widgets at market scale. The first widget won’t be sold until year 11.”
VC: “I see. How many widgets will you sell in year 11?”
You: “Maybe none. Maybe a few million. Maybe more. And I should note there’s an 88% chance that the widget we’re designing will completely fail in year five or six of testing. Then we might have to start over again.”
In the most bullish of bull markets, this conversation terminates at the exit door. When the bears are beating biotech capital markets into submission like they’ve been for the past 12 months, it’s a nonstarter.
The number of clinical-stage biopharma companies that have scaled back their workforces, relinquished assets they hadn’t planned on divesting, or worse, shuttered operations this year alone puts a fine point on the problem. Sustaining operations for a decade of development without revenue generation is a ridiculous concept. For this to look more like a fundable business to mainstream investors, drug discovery must be a speedier exercise with a higher degree of confidence in the drug’s therapeutic outcome from the outset. Drug development must happen faster, and with more certainty around quality and safety from the first run. Bioprocess operations must happen more efficiently, consuming fewer expensive raw materials and spending less time on the long continuum between cell culture and distribution of finished drug product.
Why AI Hasn’t Taken Over Biotech—Yet
Creating therapeutic biologic development efficiency is no longer a matter of competitive advantage, it’s a matter of survival. That’s why I think we’re about to see a whole lot of activity on the computational biology front. We’ve been talking about it for years, but for many of those years there were very real aversions to its adoption:
- Pretty much all technology is half-baked at best when it first hits the market. Few people are interested in paying big money for the first model year of anything (see Microsoft).
- Cash runways were long and flush. Cheap money doesn’t motivate anyone to solve a problem before the cash runs out.
- Most of the biopharma decision makers who were around when computational biology (computer-aided drug discovery and development in particular) made its first-gen debut were brought up in wet labs. Their work was predicated on research conducted by a generation of wet lab scientists before them. There was little-to-no trust in in-silico anything.
- The stakes are high. Really high. Even as the “old” whitecoats grow more comfortable with AI in their personal lives, they also see it fail all the time. If your AI-driven Google search for a good pizza in Cambridge, MA returns results for pizza shops in Cambridge Springs, PA, you get a little frustrated and remain hungry. AI errors in biopharma discovery and development, on the other hand, could cost millions of dollars and risk patient safety. (Worth noting that there are few good pizzas to be found in Cambridge Springs, PA.)
Those aversions are crumbling, and for good reason. AI-enabled DNA, RNA, protein or peptide sequencing technologies are making discovery more efficient, and efficiency in identifying winners—and weeding out losers—is prerequisite in a tough market with such a high failure rate. Drug discovery and development innovators are catching on. A 2021 GlobalData survey revealed that 70% of industry respondents anticipate drug development will be the area most impacted by the implementation of smart technologies.
Beyond computer-enabled sequencing for discovery, in-silico technologies are making development and clinical trials more efficient. They’re helping researchers predict clinical outcomes, inform clinical trial designs, support evidence of effectiveness, identify clinical trial participants, and predict product safety. I suspect that as markets slowly rebound, these will become high-priority investment opportunities for emerging biopharmas.
Barriers To AI Adoption In Biopharma
While the technology is powerful and promising, it’s not easy to implement for several reasons. Computational biologists are a rare and precious resource. Several biopharma leaders I’ve spoken with that are using compute power in discovery and testing have lauded the unique ability of their IT-oriented people to work closely with their wet lab-oriented scientists. But that’s the exception.
Software helps, and there is a growing market of life sciences-specific software providers developing tools for the analysis of large volumes of complex—and exponentially growing—data. But because the data is so large and complex, customization of those tools is almost a certainty. That comes at a cost.
Regulatory pathways are also challenging. The FDA must assess the credibility of a company’s computational modeling and simulation approach, and from what I gather, that paradigm is still being sussed out. CBER’s take on AI/Machine Learning leans toward monitoring biologic products post-approval (i.e. the Biologics Effectiveness and Safety (BEST) System). Its work supporting in-silico testing leans a bit more toward an ad-hoc approach.
These are all barriers that can and will be scaled, and the pace of the climb will accelerate as markets recover. We’ll be covering the companies investing in computational biology as it happens. If you’re one of them, get in touch. I’d love to learn more.