Where's The Case For Generative AI In Biopharmaceutical Manufacturing?

By Matthew Pillar, Editor, Bioprocess Online
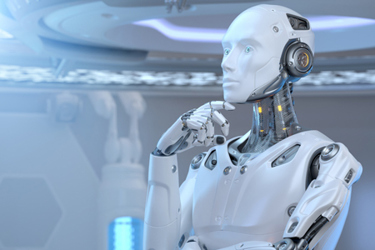
This July, we’ll drop an episode of the Business of Biotech podcast featuring a man who leads a company that’s credited with taking the first AI-designed antibody into the clinic and putting it in humans. His name is Aron Knickerbocker, his company is Aulos Biosciences, and the molecule, dubbed AU-007, is a computationally designed, IL-2 human monoclonal antibody. That story will be told on episode 210, for those taking notes. The candidate will remain in the spotlight through its clinical journey not only because it works differently than the myriad IL-2 therapeutics currently in development, but because if successful, it has the potential to, at least temporarily, silence the skeptics of AI’s expanding role in drug discovery and design.
Interestingly, those critics are largely those closest to biopharma R&D, and those closest to the adoption of the very technologies necessary to enable AI in molecular design. Anecdotally, it takes nothing more than a few minutes scrolling a search for “AI” in the biotech subreddit to pick up on this.
A new Axendia Market Research Report: The State Of Generative AI In Life Sciences, The Good, The Bad, And The Ugly, puts numbers to the tale. Among pharmaceutical DX (digital transformation) pros, 67% answered “not ready” when asked how ready the life sciences industry is to effectively leverage generative AI in terms of infrastructure, expertise, and regulatory considerations. Among R&D professionals, 43% said the same. Nearly half of all 200 survey respondents predicted that it would be more than 4 years before generative AI became mainstream in their functional area.
The early use cases for AI in the biopharmaceutical industry—at least, the early public use cases—have largely come from R&D, and more specifically, target identification and molecular design. I have friends in the cottage industry of AI-enabled drug discovery and design companies that have sprung up around AlphaFold, the Human Genome Project, and other sources of largely public datasets on which their platforms are built. The real questions for Bioprocess Online readers are, beyond discovery and design, where are the use cases in biologics manufacturing, supply chain management, QMS, and operations, and what’s holding us back?
AI In Biopharma Manufacturing
A whopping 79% of respondents to Axendia’s survey said they believe generative AI has the potential to “revolutionize” drug manufacturing, in terms of efficiency and quality. But the percentage of those respondents claiming to be current users of generative AI (5%) paints a picture that’s more evolutionary than it is revolutionary. The low-hanging application fruit, according to industry, is far-and-away process modeling and simulation, where more than a quarter of respondents say generative AI has the potential to be most beneficial. That also happens to be an area in which most drug manufacturers are flush with a resource that’s requisite to AI: data. Process optimization (19%), drug synthesis and formulation (12%), and real-time quality monitoring (10%) round out the top four most-cited opportunities.
Data access aside, there’s another reason modeling and simulation is seen as the first, best opportunity for generative AI to make an impact in pharma. It’s the discipline least encumbered by the external forces that create pause when a change to process, formulation, or monitoring is considered. Regulatory influence is primary among those forces. That goes without saying, but the numbers reinforce the truth: 69% of respondents to the Axendia survey cited regulatory compliance concerns as the top challenge or barrier to implementing generative AI in drug manufacturing. Data security and workforce knowledge—or lack thereof—come in tied for a distant second in the challenge/barrier category at 36%. Seems there’s a big chasm between the C-suite and the workforce in terms of that knowledge, though. Asked “how familiar are you with the concept of generative AI,” 82% of C-suite execs answered, “very familiar.” That confidence waned as the question moved down the ranks, to 62% of VPs/general managers and 31% of individual contributors. Just 23% of department heads and directors said the same. Gotta love those big corporate egos.
AI In Pharmaceutical Supply Chain Management
Still rattled by pandemic-era supply chain catastrophe, a third of respondents to the Axendia Market Research Report: The State Of Generative AI In Life Sciences, The Good, The Bad, And The Ugly indicated they’re looking to AI for a leg up on avoiding supply shortfalls in the future. Demand forecasting, predictive analytics, and inventory management and optimization applications are the top applications they expect generative AI to help. It’s been more than a decade since I last covered retail technology. Long before the turn of that decade, I was covering how some of the very brands that dispense pharmaceuticals (i.e. Walgreens, Rite Aid, CVS, Walmart, etc.) were leveraging machine learning-based tools from major ERP vendors like SAP and SAS to manage their suppliers and inventories. Pharma might be late for the game, but there are old lessons to be learned here. But, yet again, optimism outweighs adoption. While 56% of respondents say they’re confident or very confident that generative AI can enhance the efficiency and resilience of supply chains, 77% of them said they’re not currently using AI-driven technologies or analytical tools to manage supply chain resilience.
What’s to blame for that lack of effort? Supply chain disparity, for starters. In retail, the retailer rules. Walmart has the selling power to force all of its suppliers to do it their way or hit the highway to Amazon. That power makes data aggregation, and ensuing analysis, realtively easy. Beyond those select few atop the biopharma pyramid, no such power exists in the life sciences. During the pandemic, when everything from pipette tips to cell culture media to APIs were in short supply, the fragility of our multi-system, bend-at-the-capacity-of-suppliers supply chains was on full display. It didn’t help the case for building quality demand forecasting data when backorders were being placed via paper and telephone. Ironically, those dynamic circumstances also contribute to the lack of adoption of AI-driven supply chain management tools. Forty-two percent of survey takers expressed concern that generative AI models would struggle to adapt to unforeseen circumstances or novel scenarios. That’s the irony – the recent display of why we need it is a leading contributor to why we don’t have it.
AI In Biopharma QMS
Post-market surveillance and QMS rallied high levels of support for generative AI solutions in the Axendia report, with 77% indicating that performance reporting and metrics improvement and efficiencies hold the most potential. Yet, again, current adoption of the technology for that application is a lowly 12%
This is another case of an obvious application for the technology in a data-rich discipline, but it’s the quality and governance of that data that gives would-be adopters pause. A staggering 88% of survey respondents said they think generative AI could introduce potential quality risks. That’s a big barrier to clear in a stubbornly conservative industry – that obstinance motivated by said industry’s highly regulated reality – but it’s not unwarranted. Biased data builds biased algorithms, biased algorithms yield biased results, biased results produce biased regulators, and biased regulators net bad outcomes for biopharmas.
AI In Lab Operations
Of the disciplines mentioned here, data analysis in lab operations has garnered the most current adoption of generative AI technology. Still, just two in ten survey respondents said they’re using it, despite the 70 percent who indicated they believe the technology holds the potential to revolutionize lab processes in terms of efficiency and quality. Data analysis and interpretation leads the charge, with 94% indicating those exercises would benefit the most from generative AI, followed distantly by workflow and process optimization at 54%.
Why do 94% of respondents to the Axendia survey believe in the potential of AI to improve laboratory understanding, while just 21% are using it to do as much? The problem isn’t the program, it’s the people. Nearly half said their workforce isn’t ready for it, and 35 percent said competing priorities stand in the way of adoption. Those are people problems, and one of those problems might well be aforementioned: there’s a breakdown between corporate perception of AI’s power and lab-level acceptance of it. That lack of acceptance is ripe for exploration. Is it fear that generative AI might minimize workforce value, or worse, displace certain facets of the workforce altogether? Survey takers say no – just 9% of them are “very concerned” about the effect of AI on their own jobs over the next 2-3 years (21% “somewhat concerned”), while 69% aren’t concerned at all.
Maybe there’s something deeper going on here. Maybe the dichotomy between C-level acceptance and operations-level skepticism is rooted in an inconvenient reality that doesn’t fit the tech-bio narrative. Or maybe the skunkworks generative AI operations known to the C-suite aren’t so transparent to the floor-level biotech redditers who downplay the technology’s power. More research needs to be done. I’ll see what ChatGPT and Perplexity have to say about it. In the meantime, there’s Aulos and AU-007, which just might become the poster child for AI in biopharma.
What do you think? Email me, or connect with me on LinkedIn to weigh in on where AI belongs, or doesn't, in biopharma.
Interested in seeing Axendia's research firsthand? Life science industry professionals, regulators, and those in academia can receive the report free of charge upon registration here.