Using AI To Predict Multispecific Formulation Patterns
By Vadim Klyushnichenko, Ph.D., Calibr-Skaggs Institute for Innovative Medicines
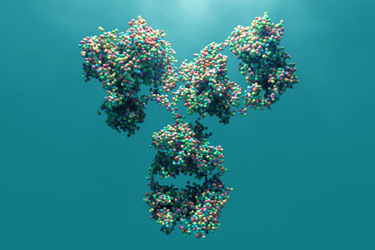
Bispecific and trispecific antibodies are engineered to bind two or more distinct antigens or epitopes, enabling novel therapeutic mechanisms such as T cell redirection, dual immune checkpoint blockade, and multispecific viral neutralization. Their promise has driven rapid clinical growth: as of March 2025, over 600 bispecific antibodies are in clinical trials, with 17 FDA-approved products and a projected market exceeding $50 billion by 2030.1,2 Additionally, more than 50 trispecific antibodies are in clinical development, with first approvals anticipated by 2028.3 The U.S. and China dominate bispecific and trispecific antibody research.
Despite their potential, multispecific antibodies introduce substantial formulation challenges beyond those of conventional mAbs. Structural asymmetry, engineered linkers, and non-native interfaces increase molecular heterogeneity, aggregation propensity, and solution viscosity. These characteristics often render platform mAb formulations insufficient, requiring customized approaches. Moreover, multispecifics frequently necessitate orthogonal analytical methods to characterize higher-order interactions and complex degradation pathways, increasing analytical and regulatory complexity.
This article explores the key formulation challenges unique to multispecific antibodies, reviews emerging AI-enabled formulation strategies, and provides practical insights into the implementation of commercial AI platforms for predictive formulation development, particularly within small to midsize biopharmaceutical companies. Finally, the importance of robust experimental validation to confirm AI-derived formulation predictions and ensure regulatory readiness is emphasized.
A Complex Therapeutic Class
However, their structural and functional complexity poses unique challenges throughout development. Unlike conventional mAbs, bispecific and trispecific antibodies often:
- contain asymmetric chains, additional binding domains, or engineered linkers,
- exhibit greater molecular heterogeneity from mispaired chains or partial assembly,
- show increased surface hydrophobicity or charge heterogeneity, and
- require novel analytical methods to characterize multiple species.
From a formulation perspective, these features translate into higher risks of aggregation, viscosity, and colloidal instability.4 Traditional monoclonal antibody formulation “platforms” — such as histidine or citrate buffers at pH 5-6 with polysorbate — often fail to achieve sufficient stability for bispecific constructs.
From a formulation perspective, these features translate into higher risks of aggregation, viscosity, and colloidal instability. Traditional monoclonal antibody formulation platforms, such as histidine or citrate buffers at pH 5-6 with polysorbate, sometimes fail to achieve sufficient stability for bispecific constructs.
Moreover, increased molecular weight and asymmetry often drive solution viscosity above 30-50 cP at 100 mg/mL, complicating both fill/finish and subcutaneous administration.5
Additionally, the lack of established developability rules for novel formats forces developers into larger, more expensive formulation screens, with no guarantee of success.
Table 1. Key Formulation Challenges in Bi- and Trispecific Antibodies vs. Conventional mAbs
Challenge | Conventional mAbs | Bi- & Trispecific Abs |
Structural heterogeneity | Low | High |
Aggregation propensity | Moderate | High |
Viscosity at high concentration | 5-20 cP | Can be 2X-3X higher |
Analytical complexity | Standard assays | Multi-domain assays |
Colloidal stability | Well-characterized | Poorly predicted |
Stability screening burden | Moderate | High |
Data adapted from recent literature on bispecific antibody developability challenges.
Industry Examples
Industry examples of approved and clinical-stage bispecific and trispecific antibodies underscore the multifaceted formulation challenges unique to these complex molecules. As shown in Table 2, key hurdles include increased aggregation propensity, elevated viscosity at therapeutic concentrations, reduced conformational and colloidal stability, and greater structural heterogeneity due to engineered linkers, asymmetric chains, or non-native interfaces.
Unlike conventional mAbs, these multispecific formats often fall outside platform formulation solutions, necessitating tailored strategies to ensure stability, manufacturability, and delivery feasibility. Developers have addressed these challenges through diverse approaches, including excipient screening for aggregation suppression, pH and ionic strength optimization to mitigate viscosity, protein engineering to reduce hydrophobic surface area, and incorporation of enzyme co-formulation (e.g., hyaluronidase) to enable subcutaneous administration. Collectively, these examples highlight the need for expanded formulation toolkits and data-driven design methods to overcome the intrinsic complexity of next-generation antibody therapeutics.
Table 2. Formulation challenges and strategies across approved and clinical-stage bispecific and trispecific antibodies. Approved products are highlighted in green 🟩; clinical-phase candidates are yellow 🟨.
Antibody | Targets | Indication | Sponsor | Approval Year / Phase | Formulation Challenge | Formulation Strategy |
---|---|---|---|---|---|---|
Blinatumomab | CD19 × CD3 | B-ALL (relapsed/refractory) | Amgen | 2014 (FDA) | Short half-life; instability of small BiTE, lacking Fc | Trehalose, polysorbate 80; pH optimization; cold-chain continuous infusion |
Emicizumab | FIXa × FX | Hemophilia A prophylaxis | Roche/Chugai | 2017 (FDA) | Stability of engineered Fc; disulfide scrambling | Controlled redox; optimized buffer; mild surfactants |
Mosunetuzumab | CD20 × CD3 | B-cell lymphoma | Genentech/Roche | 2022 (EMA) | Mispaired chains; aggregation at high conc. for SC delivery | Chromatographic purification; excipient screening; PEGylation explored |
Teclistamab | BCMA × CD3 | Multiple myeloma | Janssen (J&J) | 2022 (FDA) | Aggregation; immunogenicity; impurity sensitivity | Anti-aggregation excipients; impurity control; high-res SEC for aggregate monitoring |
Amivantamab | EGFR × MET | NSCLC (EGFR exon20ins mutations) | Janssen (J&J) | 2021 (FDA) | High viscosity at therapeutic conc. | Arginine, histidine; pH optimization; hyaluronidase co-formulation |
DuoBody platform** | Various (platform tech) | Oncology, immunology indications | Genmab | Multiple phases | Fab-arm exchange equilibrium; homodimer reformation | Buffer stabilization of Fab-Fc; screening excipients; stabilizing linkers |
SAR442257 | CD38 × CD3 × CD28 | Hematologic malignancies | Sanofi | Phase 1 | Elevated aggregation; viscosity; analytical complexity | Multi-attribute methods; predictive modeling; polyols, amino acids |
REGN1979 (Odronextamab) | CD20 × CD3 | B-cell NHL | Regeneron | Phase 2 | Aggregation; SC delivery challenges at high conc. | Protein engineering to reduce hydrophobic patches; viscosity-reducing excipients |
Tebotelimab | PD-1 × LAG-3 | Solid tumors | MacroGenics | Phase 2 | Stability of tandem checkpoint-binding arms; high viscosity | Buffer tuning; amino acid excipients; linker optimization |
Zenocutuzumab | HER2 × HER3 | NRG1 fusion-positive cancers | Merus | Phase 2 | Aggregation from heterodimer interface; conformational instability | Domain stabilization via engineering; excipient screening for interface stabilization |
Epcoritamab | CD20 × CD3 | B-cell NHL | Genmab/AbbVie | 2023 (EMA) | Aggregation; viscosity for SC administration | High-throughput excipient screening; co-formulation with hyaluronidase |
Glofitamab | CD20 × CD3 | Diffuse large B-cell lymphoma | Genentech/Roche | 2023 (FDA) | Aggregation control; immunogenicity risk | Glycosylation optimization; polysorbate stabilization; low-pH buffer |
*Formulation strategies reflect published literature and conference disclosures; specific proprietary methods may not be publicly reported, some inferred from product characteristics
**Platform technologies (e.g., DuoBody) represent multiple molecules rather than a single product
A variety of platform technologies underlie the design and manufacturability of the bispecific and trispecific antibodies listed in Table 2. Each platform addresses specific molecular assembly challenges, particularly heavy and light chain pairing and heterodimer formation. The BiTE platform (Amgen), used for blinatumomab, employs a minimal scFv–scFv linker format lacking an Fc region, enabling T cell engagement but requiring continuous infusion due to rapid clearance.
Roche and Genentech have widely employed knobs-into-holes (KiH) Fc engineering and CrossMab technology in antibodies like mosunetuzumab and glofitamab, promoting correct heavy chain heterodimerization and reducing light chain mispairing through domain crossover. Genmab’s DuoBody platform, utilized for epcoritamab, uses a post-expression Fab-arm exchange method to create bispecific IgG1 molecules with native-like architecture. Merus’ zenocutuzumab leverages a common light chain strategy, simplifying pairing by using a single light chain compatible with both antigen-binding arms. Each platform introduces unique structural features influencing colloidal stability, aggregation, and formulation behavior, necessitating customized formulation strategies beyond standard monoclonal antibody solutions. These diverse engineering platforms are illustrated in Figure 1, highlighting how structural design influences manufacturability, stability, and formulation strategies.
Figure 1. Representative bispecific antibody platform architectures: BiTE, knobs-into-holes, DuoBody, CrossMab, and common light chain. Structural differences influence manufacturability, stability, and formulation considerations.
AI-Enabled Strategies For Formulation Development
The complexity of bispecific and trispecific antibodies presents substantial challenges to formulation scientists, including heightened risks of aggregation, elevated viscosity, and structural heterogeneity. Traditional empirical screening approaches are often inefficient and insufficient for such molecules. In this context, artificial intelligence (AI) offers transformative opportunities to enhance prediction, streamline formulation screening, and enable data-driven design.
Recent advances in AI, including machine learning (ML), deep learning, and natural language processing (NLP), are enabling more predictive and systematic approaches to biologic formulation. Below, we describe key AI-enabled strategies applicable to multispecific antibody formulation, supported by recent research and industry examples.
Sequence-Based Developability Prediction
Advancements in AI have significantly enhanced the early-stage assessment of antibody developability by analyzing amino acid sequences to predict potential liabilities such as aggregation, poor solubility, and immunogenicity. ML models trained on extensive antibody sequence data sets can evaluate physicochemical properties, including hydrophobicity, charge distribution, and isoelectric points, to assess developability risks early in the design process.
For instance, a study by Lai et al. (2022) demonstrated the use of ML algorithms to predict aggregation rates and viscosity of therapeutic antibodies at high concentrations.6 By analyzing features such as spatial charge distribution and solvent-accessible hydrophobic areas, the models achieved high predictive performance, facilitating early identification of candidates with favorable developability profiles.
Additionally, GenScript has developed AI-driven platforms that evaluate antibody sequences for developability, enabling early identification of problematic regions before expression or formulation screening. Their approach focuses on predicting aggregation and solubility, immunogenicity risk assessment, and thermostability profiling directly from antibody sequences.
These AI-enabled strategies allow for the early identification of potential developability issues, guiding sequence optimization and reducing the risk of late-stage failures in antibody development.
Structure-Based In Silico Stability And Interaction Modeling
Advancements in structural prediction tools, notably AlphaFold2, combined with ML-enhanced molecular dynamics (MD) simulations, have significantly improved our ability to visualize and assess antibody architectures. These models can evaluate aggregation-prone interfaces, exposed hydrophobic patches, and domain flexibility, providing insights into potential stability issues. Such structural analyses inform computational workflows to predict excipient interactions and buffer effects, reducing the need for extensive empirical screening.
For instance, Pak et al. (2023) investigated the capability of AlphaFold2 to predict the impact of single mutations on protein stability and function.8 Their findings suggest that while AlphaFold2 excels in structure prediction, its utility in predicting stability changes due to mutations is limited, highlighting the need for integrated approaches combining structural predictions with other computational methods.
Additionally, Lai et al. (2022) developed ML models incorporating features from MD simulations to predict antibody aggregation rates and viscosity.9 By analyzing spatial charge distributions and solvent-accessible hydrophobic areas, their models achieved high predictive performance, facilitating early identification of candidates with favorable developability profiles.
These AI-driven structural analyses enable early identification of potential developability issues, guiding sequence optimization and formulation strategies, and reducing the risk of late-stage failures in antibody development.
Data-Driven Formulation Design
The integration of AI and ML into biopharmaceutical formulation design has revolutionized the prediction and optimization of antibody stability and viscosity. By analyzing extensive data sets, these models can identify correlations between formulation components — such as buffer types, pH levels, ionic strength, and excipient combinations — and the resulting biophysical properties of antibodies.
For instance, Narayanan et al. (2021) demonstrated the application of a Bayesian optimization algorithm to accelerate the design of biopharmaceutical formulations.10 Their approach significantly reduced the number of experiments required to identify optimal formulations, enhancing efficiency in the development process.
In addition to academic advancements, commercial software platforms are facilitating the integration of AI workflows into formulation pipelines. BIOVIA Pipeline Pilot offers a comprehensive suite of machine learning and data modeling tools, enabling scientists to design, test, and deploy predictive models without extensive programming knowledge. This platform supports tasks ranging from data ingestion and cleaning to model building and deployment, streamlining the formulation development process.
Similarly, Benchling provides a cloud-based R&D platform that combines electronic lab notebooks, molecular biology tools, and data analytics. Its Benchling Bioprocess module allows for the structured collection and analysis of process data, feeding into machine learning models to generate predictive insights. This integration aids researchers in making informed decisions during formulation development.
Integration With High-Throughput Analytical Data
High-throughput (HT) analytical platforms are pivotal in biopharmaceutical formulation development, enabling rapid assessment of protein stability and aggregation across diverse conditions. Techniques such as dynamic light scattering (DLS), size-exclusion chromatography (SEC), differential scanning calorimetry (DSC), and viscosity measurements generate comprehensive data sets essential for formulation optimization.12-14 These methods can be used not only for the initial protein characterization but also for online monitoring of manufacturing as a part of process analytical technologies (PAT).15
The integration of AI and ML with these HT analytical methods facilitates:
- Anomaly detection: AI algorithms can identify outliers and inconsistencies in large data sets, ensuring data quality and reliability.
- Trend analysis: ML models can uncover subtle patterns and correlations between formulation variables and stability outcomes, aiding in the optimization of formulation conditions.
- Multi-parameter visualization: Advanced analytics enable the visualization of complex stability landscapes, assisting researchers in understanding the interplay between various formulation factors.
High-throughput DLS and DSC generate large data sets that could benefit from AI/ML integration for improved pattern recognition and predictive modeling. While direct applications of AI to such data in formulation contexts are still emerging, foundational HT analytical platforms provide the necessary data infrastructure.16,17
These integrative approaches can not only expedite the formulation development timeline but also improve the precision of stability predictions, ultimately leading to more robust and effective biopharmaceutical products.
Natural Language Processing For Knowledge Mining
Natural language processing (NLP) techniques are increasingly utilized to extract relevant insights from vast biomedical literature, patents, and regulatory documents. These methods aid in identifying buffer systems, excipient choices, stress stability findings, and formulation strategies pertinent to antibody development.
For instance, NLP tools have been developed to automatically identify antibody and antigen entities from scientific articles, facilitating the extraction of valuable information for formulation scientists.18
Additionally, AI-based text mining applications leverage NLP to recognize entities and extract relationships within biomedical texts, enhancing the efficiency of knowledge discovery in drug development.19
These approaches are particularly valuable for novel bispecific antibody formats with limited historical data, enabling scientists to infer knowledge from analogous constructs and augment empirical screening with evidence-based recommendations.
Table 3. Summary of AI-Enabled Strategies for Multispecific Antibody Formulation
AI Strategy | Key Applications |
Sequence-Based Prediction | Aggregation propensity; chemical instability; developability scoring |
Structure-Based Modeling | Interface stability; aggregation hotspots; excipient interaction prediction |
Data-Driven Formulation Design | Buffer/excipient recommendation; predictive DoE; narrowing screening space |
Integration with HT Analytics | Automated data analysis; anomaly detection; trend visualization |
NLP-Based Knowledge Mining | Literature/patent mining; precedent extraction; regulatory guidance |
Collectively, these AI-enabled strategies transition formulation development from empirical trial-and-error approaches toward predictive, knowledge-augmented designs, offering significant advantages in the development timeline and robustness of bispecific and trispecific antibodies.
Practical AI Platforms For Formulation Development At Bio/Pharmaceutical Institutions
AI offers powerful opportunities to accelerate and improve formulation development for biopharmaceuticals. While large pharmaceutical companies may invest in building proprietary AI platforms, supported by significant computational resources, in-house data science teams, and long-term R&D budgets, such custom development can be prohibitively expensive for small and midsize companies. These smaller organizations often rely on contract development and manufacturing organizations (CDMOs) for formulation services, which, while effective, can also be costly and inflexible.
For companies aiming to build internal expertise, especially those developing their own bispecific or trispecific antibody platforms, adopting commercially available AI-enabled formulation tools provides a feasible, scalable solution.
The use of AI platforms for formulation development offers several benefits. These tools enable companies to reduce reliance on traditional trial-and-error experimentation by applying predictive models trained on historical or proprietary data. AI can help identify optimal buffer systems, excipients, and process parameters, reduce the number of formulation conditions requiring wet-lab testing, and enhance understanding of complex molecular interactions. By integrating data-driven approaches earlier in development, companies can accelerate timelines, improve robustness, and lower experimental costs.
Several commercial and open-source AI platforms are now available to support formulation development, offering accessible solutions for small and midsize companies aiming to reduce experimental burden and accelerate development of complex biologics. These platforms vary in scope, from data integration and predictive modeling tools to in-silico prototyping environments, enabling organizations to select solutions aligned with their resources and formulation challenges.
Table 4. AI Platforms Supporting Formulation Development for Biopharmaceuticals
Platform | Key Capabilities |
BIOVIA AI-Powered Formulation |
Predictive formulation modeling, data integration, machine learning workflows for biologics and small molecules. |
Persist.AI | AI-powered optimization of drug formulations, small data set learning, autonomous formulation pipelines. |
ChemIntelligence | AI-driven formulation development, prediction of material interactions, formulation optimization consulting. |
VeriSIM Life – BioiSIM | In silico platform for formulation prediction, translational modeling, virtual prototyping to reduce in vivo testing. |
FormulationAI (ComputPharm) | Open-source AI for pharmaceutical formulation, predictive modeling tools accessible to academic and industry users. |
Dotmatics AI Native Multimodal Platform | Data management, AI-based multimodal data analysis, workflow automation, integration with ELN and R&D systems. |
These commercially available AI platforms provide small to midsize biopharmaceutical companies with accessible entry points into predictive, data-driven formulation development. They eliminate the need to build complex AI infrastructure from scratch while offering flexibility to customize workflows and integrate experimental data. For companies aiming to develop multispecific antibody platforms or other complex biologics, adopting such AI tools can bridge the gap between empirical screening and predictive design, ultimately improving development efficiency, reducing costs, and enhancing product quality.
Illustrative Case Studies Of AI Platform Implementation For Formulation Development
Case Study 1: Reducing Viscosity Challenges in a Bispecific Antibody Using a Commercial AI Formulation Platform
A midsize biotech developing a CD3xCD20 bispecific antibody for B-cell lymphoma encountered challenges achieving an injectable viscosity at 150 mg/mL for subcutaneous administration. Due to limited in-house formulation data (fewer than 20 experimental formulations), the team sought to augment their knowledge by leveraging published data.
They adopted a commercial AI-enabled formulation platform capable of mining and analyzing hundreds of peer-reviewed publications, patents, and public formulation databases related to monoclonal and multispecific antibody formulations. Using NLP and machine learning, the platform extracted relationships between formulation variables (pH, excipients, ionic strength) and viscosity outcomes across diverse antibody formats.
The AI model identified trends showing that trehalose and arginine at specific concentrations, combined with pH near 5.5, were associated with lower viscosity in structurally similar antibodies. Based on these insights, the team designed five formulation candidates instead of screening the originally planned 30 conditions.
Subsequent lab testing confirmed that two of these AI-prioritized formulations achieved target viscosity below 25 cP, meeting both manufacturability and delivery requirements, while saving eight weeks of experimental screening.
Key takeaway: An AI-driven platform leveraging external literature and patent mining enabled knowledge-augmented formulation design, reducing experimental scope without relying solely on limited internal data sets.
Case Study 2: Centralizing Data and Enabling Predictive Stability Modeling Using a Commercial Cloud-Based Formulation Platform
A small biopharmaceutical company developing a HER2xHER3xEGFR trispecific antibody faced difficulties consolidating formulation data generated across multiple CROs and internal labs. Data were dispersed across spreadsheets, PDFs, and unstructured reports, making it challenging to analyze formulation outcomes across antibody variants.
The company implemented a commercial cloud-based formulation data management platform with integrated analytics and compatibility for machine learning tools. This platform centralized experimental data from disparate sources, standardizing annotations for formulation variables (pH, buffer type, excipients) and analytical outcomes (aggregation, thermal stability, viscosity).
After consolidating 50 historical formulation data sets, the team applied a built-in machine learning module to model the relationship between formulation components and thermal stability (Tm). The model incorporated excipients beyond common polysorbates and sugars, including cyclodextrins, methionine, histidine, cysteine, EDTA, and ascorbic acid, exploring their potential impact on stability.
Visualization of model outputs identified that combinations including arginine, histidine, and low concentrations of β-cyclodextrin were associated with higher thermal stability for certain antibody variants, while formulations excluding methionine showed lower oxidation rates.
Based on these insights, the team prioritized six formulation candidates for experimental verification. Three candidates demonstrated a 2 degree to 3 degree C increase in Tm and improved oxidation resistance compared to the baseline formulation.
Key takeaway: A cloud-based AI-enabled platform allowed a small biotech to unify fragmented formulation data, apply predictive modeling, and identify underutilized excipients contributing to improved stability profiles.
These examples illustrate how commercially available AI platforms can be applied in practical, scalable ways for formulation development, especially at smaller companies with limited in-house AI resources. By leveraging existing data — either proprietary or external — AI tools can prioritize experimental efforts, reduce screening burden, and enhance formulation robustness for complex antibody therapeutics.
Barriers And Considerations For AI Adoption In Formulation Development
While AI-enabled platforms offer significant promise for improving formulation development of bispecific and trispecific antibodies, their implementation is not without challenges. Practical barriers include:
- Limited training data for novel antibody formats: Most available formulation data sets pertain to conventional monoclonal antibodies, with sparse data on multispecific constructs.
- Generalizability gaps across molecular architectures: AI models trained on existing antibodies may not fully capture the biophysical nuances of unique bispecific or trispecific formats.
- Validation and regulatory considerations: Formulation decisions informed by AI models will require robust experimental confirmation to meet regulatory expectations for data traceability and scientific justification.
- Integration with legacy informatics systems: Many small and midsize companies operate on disparate or outdated data systems, complicating seamless integration of AI platforms.
Addressing these barriers will require collaboration across formulation scientists, data scientists, and regulatory experts to ensure that AI tools are appropriately validated, interpretable, and effectively integrated into existing development workflows.
To mitigate these challenges, companies can adopt several strategies:
- Start by building internal data sets systematically through structured data collection, even from small-scale experiments, to incrementally train more relevant AI models.
- Engage with AI vendors that offer transfer learning or access to pretrained models adaptable to multispecific antibody data.
- Establish early collaboration between formulation teams and data scientists to ensure models incorporate domain-relevant features and constraints. Importantly, integrate AI workflows alongside, not in place of, empirical testing, using AI as a prioritization tool rather than sole decision maker.
- Proactively engage with regulatory agencies to discuss AI-assisted workflows and validation plans, fostering transparency and acceptance.
Conclusion
The formulation of bispecific and trispecific antibodies presents unique challenges stemming from their structural complexity, including increased aggregation propensity, high viscosity, and greater molecular heterogeneity compared to monoclonal antibodies. Traditional empirical formulation approaches often fall short for such molecules. AI offers a promising pathway to augment formulation development by enabling predictive modeling, data integration, and knowledge mining from literature and experimental data sets.
Nevertheless, AI-based formulation decisions must be supported by robust experimental validation using orthogonal analytical techniques to ensure product stability, manufacturability, and regulatory compliance. Techniques such as SEC-MALS, DLS, DSC, SIC, and charge variant analysis remain essential for confirming AI predictions and meeting regulatory expectations for data integrity and traceability.
To successfully integrate AI into formulation development, companies must navigate barriers related to data availability, model generalizability, validation requirements, and informatics integration. Addressing these challenges through cross-functional collaboration between formulation scientists, data scientists, and regulatory experts will be key to unlocking the full potential of AI in multispecific antibody formulation. Ultimately, the convergence of AI and experimental science offers a transformative opportunity to improve efficiency, reduce costs, and accelerate access to next-generation biologics.
References:
- Kuick Research. Global Bispecific Antibody Market Opportunity, Drug Dosage, Patent, Price, Sales and Clinical Trials Insight 2030, March 2025. https://www.kuickresearch.com/report-global-bispecific-antibody-market-size
- GlobeNewsWire. Bispecific Antibody Market Opportunity, Drug Dosage, Patent, Price, Sales & Clinical Trials Insight 2030: New Report Highlights 600+ Bispecific Antibodies in Clinical Trials Worldwide. https://www.globenewswire.com/news-release/2025/03/12/3041385/0/en/Bispecific-Antibody-Market-Opportunity-Drug-Dosage-Patent-Price-Sales-Clinical-Trials-Insight-2030-New-Report-Highlights-600-Bispecific-Antibodies-in-Clinical-Trials-Worldwide.html
- Kuick Research. Global Trispecific Antibodies Clinical Trials, Fast Track Status, Technology Platforms and Market Opportunity Outlook 2025. March 2025. https://www.kuickresearch.com/report-trispecific-antibodies-market
- Amash, A., Volkers, G., et al. (2024). Developability considerations for bispecific and multispecific antibodies. mAbs, 16(1), 2394229. https://pubmed.ncbi.nlm.nih.gov/39189686/
- Majumder, S., Wang, W., & Alphonse Ignatius, A. (2019). Impact of Buffers on Colloidal Property and Aggregation Propensities of a Bispecific Antibody. Journal of Pharmaceutical Sciences, 108(3), 1139–1147. https://pubmed.ncbi.nlm.nih.gov/30391414/
- Zhao, J., et al. (2018). Viscosity challenges in high-concentration bi-specific antibody formulations. European Journal of Pharmaceutics and Biopharmaceutics, 128, 98–106.
- Lai, P.-K., Gallegos, A., Mody, N., Sathish, H. A., & Trout, B. L. (2022). Machine learning prediction of antibody aggregation and viscosity for high concentration formulation development of protein therapeutics. mAbs, 14(1), 2026208. https://doi.org/10.1080/19420862.2022.2026208
- GenScript. (2025). AI-Powered Antibody Drug Development: Revolutionizing Developability Assessment Through Intelligent Innovation. Retrieved from https://www.genscript.com/learning-center/revolutionizing-developability-assessment-through-intelligent-innovation.html
- Pak, M. A., et al. (2023). Using AlphaFold to predict the impact of single mutations on protein stability and function. PLoS ONE, 18(3), e0282689. https://doi.org/10.1371/journal.pone.0282689PLOS+1PubMed+1
- Lai, P.-K., et al. (2022). Machine learning prediction of antibody aggregation and viscosity for high concentration formulation development of protein therapeutics. mAbs, 14(1), 2026208. https://doi.org/10.1080/19420862.2022.2026208
- Narayanan, H., et al. (2021). Design of Biopharmaceutical Formulations Accelerated by Machine Learning. Molecular Pharmaceutics, 18(10), 3843–3853. https://doi.org/10.1021/acs.molpharmaceut.1c00469
- Kwan, T.O.C., et al. (2022) Measuring Protein Aggregation and Stability Using High-Throughput Biophysical Approaches. Front. Mol. Biosci., Sec. Structural Biology (9) https://doi.org/10.3389/fmolb.2022.890862
- Some D., et al. High-Throughput Dynamic Light Scattering (HT–DLS) for Screening Biotherapeutic Formulations. Wyatt Technology Corp. Corporate whitepaper. https://www.wyatt.com/files/literature/white-papers/High-Throughput-Dynamic-Light-Scattering-for-Screening-Biotherapeutic-Formulations.pdf
- Dauer K., et al. (2021) Microwell Plate-Based Dynamic Light Scattering as a High-Throughput Characterization Tool in Biopharmaceutical Development, Pharmaceutics 13(2), 172; https://doi.org/10.3390/pharmaceutics13020172
- Yu Z., et al. (2013) Utilizing dynamic light scattering as a process analytical technology for protein folding and aggregation monitoring in vaccine manufacturing. J Pharm Sci. 102(12):4284-90 https://pubmed.ncbi.nlm.nih.gov/24122727/
- Liu et al., (2022). High-throughput dynamic light scattering for formulation screening of monoclonal antibodies, mAbs, 14(1): 1-7, e2022142 https://pmc.ncbi.nlm.nih.gov/articles/PMC9149252/
- Vladimir I. Razinkov, Gerd Kleemann. High-Throughput Formulation Development of Biopharmaceuticals: Practical Guide to Methods and Applications. Elsevier Woodhead Publishing, 1st Edition (2016).
- Dinh T.T., et al. (2022) Extract antibody and antigen names from biomedical literature. BMC Bioinformatics 23:524 https://doi.org/10.1186/s12859-022-04993-4
- He F., et al, (2023) Applications of cutting-edge artificial intelligence technologies in biomedical text mining. Med. Rev. 2023; 3(3): 200–204 https://doi.org/10.1515/mr-2023-0011
About The Author:
Vadim Klyushnichenko, Ph.D., is vice president of pharmaceutical development and quality at Calibr–Skaggs Institute for Innovative Medicines, a division of The Scripps Research Institute. He has over 20 years of leadership experience in pharmaceutical development, manufacturing, quality systems, and regulatory compliance. His interests include exploring innovative technologies, including artificial intelligence, to enhance pharmaceutical development, manufacturing, and quality management.