AI's Potential To Aid Multispecific Analytical Characterization
By Vadim Klyushnichenko, Ph.D., Calibr-Skaggs Institute for Innovative Medicines
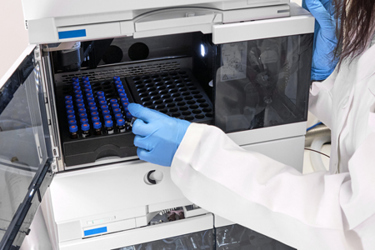
While AI tools can predict formulation parameters, their recommendations must be experimentally validated through robust analytical characterization. The following section outlines key analytical methods essential for assessing stability, aggregation, and manufacturability of multispecific antibodies.
The structural complexity and molecular heterogeneity of bispecific and trispecific antibodies demand a broad and sophisticated analytical toolkit to ensure formulation stability, manufacturability, and regulatory compliance. In addition to traditional methods used for mAbs, multispecifics often require specialized assays to evaluate higher-order interactions, colloidal behavior, and unique degradation pathways.
Part 1 of this discussion described how artificial intelligence (AI) helps predict bispecific and trispecific formulation patterns in the current state of art. Here, we’ll explore how it helps in the analytical characterization of these complex therapeutics.
Robust and orthogonal analytical techniques are essential to characterize critical quality attributes, support regulatory submissions, and experimentally validate AI-predicted formulation strategies. Below is an overview of key analytical methods applied in the formulation development of multispecific antibodies.
Aggregation and size characterization
Multispecific antibodies are prone to aggregation from domain mispairing, incomplete assembly, and hydrophobic interfaces. Multiple orthogonal techniques are recommended:
- Size-exclusion chromatography (SEC): quantifies soluble aggregates and monitors monomer purity. SEC is widely used for aggregation analysis of pharmaceutical proteins.
- Multi-angle light scattering (MALS): provides absolute molar mass of monomer and aggregates. MALS detection has been coupled with ion exchange chromatography (IEX), demonstrating its value in combination with non-size-based fractionation methods.
- Dynamic light scattering (DLS): enables rapid screening of colloidal stability across formulation conditions. DLS is a powerful tool for monitoring and characterizing nanoparticle aggregation dynamics.
- Analytical ultracentrifugation (AUC): characterizes aggregate species without chromatography-induced artifacts. AUC is routinely used as an assay orthogonal to SEC for aggregate quantitation.
- Accelerated stress testing: exposure to thermal, oxidative, and UV/visible stressors to assess degradation pathways. Such studies reveal the prominent stability of liquid-formulated biotherapeutic asymmetric monovalent bispecific antibodies.
- Freeze-thaw studies: evaluate aggregation and stability following repeated freezing and thawing cycles. The composition of the formulation buffer and the number of freeze–thaw cycles can affect antibody stability.
Charge and hydrophobicity characterization
Engineered binding domains and fusion partners may introduce additional charge and hydrophobic heterogeneity:
- Ion exchange chromatography (IEC): separates charge variants for batch consistency monitoring. IEC is based on the reversible adsorption of charged protein molecules to the immobilized exchange groups.
- Imaged capillary isoelectric focusing (icIEF): high-resolution profiling of charge isoforms. icIEF-based methods have been developed and validated as multi-attribute methods for bispecific antibodies.
- Hydrophobic interaction chromatography (HIC): assesses relative hydrophobicity influencing aggregation risk. HIC is a versatile method for the purification and separation of biomolecules by using hydrophobicity.
Colloidal and self-interaction characterization
Multispecific antibodies may exhibit increased self- and cross-interactions, impacting viscosity and clearance:
- Self-interaction chromatography (SIC): measures protein self-interactions predictive of high-concentration viscosity. SIC has been evaluated as a screening method for physical characterization of protein–protein interactions.
- Cross-interaction chromatography (CIC): detects non-specific interactions with plasma proteins or matrix components. CIC can rapidly identify the aggregation stability of new antibody formulations.
- Affinity-capture self-interaction nanoparticle spectroscopy (AC-SINS): low-sample alternative to SIC for detecting weak self-association. AC-SINS is capable of identifying mAbs with different self-association propensities.
- Second virial coefficient (B22): quantifies net protein-protein interaction forces under formulation conditions. B22 is used as a predictor of protein aggregation.
Functional and non-specific binding assays
Screening for unintended binding is critical to mitigate immunogenicity or off-target effects:
- DNA binding assay: detects non-specific binding to negatively charged nucleic acids. Antibodies can bind to single-stranded DNA with affinities as high as KD = 1 µM.
- Baculovirus and insulin binding assays: identify potential cross-reactivity to viral contaminants or endogenous hormones. The baculovirus particle (BVP) binding assay has been utilized to evaluate polyreactivity of antibodies.
Thermal and structural stability
Evaluating conformational stability and unfolding transitions ensures formulation resilience:
- Differential scanning calorimetry (DSC): measures thermal unfolding transitions (Tm) of individual domains.
- Differential scanning fluorimetry (DSF): higher-throughput screening of thermal stability.
- Glass transition temperature (Tg’): relevant for lyophilized formulations.
- Fourier-transform infrared (FTIR) spectroscopy: monitors secondary structure integrity.
- Hydrogen-deuterium exchange mass spectrometry (HDX-MS): probes higher-order structure and domain flexibility.
- Liquid chromatography-mass spectrometry (LC-MS): confirms sequence integrity, molecular mass, and post-translational modifications.
Rheology and viscosity
High-concentration formulations often require viscosity optimization:
- Viscosity measurement: performed across formulation conditions to ensure manufacturability and suitability for subcutaneous administration.
Bridging AI Predictions With Experimental Validation
These analytical methods are integral to validating AI-predicted formulation parameters. AI-driven suggestions regarding excipient choices, pH ranges, or buffer systems can be experimentally confirmed using aggregation, stability, and viscosity assays. Additionally, high-quality data generated from these assays can iteratively train or refine AI models, improving future predictive accuracy.
By combining AI-assisted formulation design with orthogonal analytical validation, developers can achieve more robust, scalable, and regulatory-compliant formulations for bispecific and trispecific antibodies.
Conclusion
For small and midsize biopharmaceutical companies, commercially available AI-enabled platforms provide a practical solution to leverage predictive analytics without the need for proprietary infrastructure. By integrating AI tools for sequence-based developability assessment, structure-based modeling, data-driven formulation design, and natural language processing for literature mining, developers can narrow experimental designs, prioritize promising formulations, and accelerate development timelines.
About The Author:
Vadim Klyushnichenko, Ph.D., is vice president of pharmaceutical development and quality at Calibr–Skaggs Institute for Innovative Medicines, a division of The Scripps Research Institute. He has over 20 years of leadership experience in pharmaceutical development, manufacturing, quality systems, and regulatory compliance. His interests include exploring innovative technologies, including artificial intelligence, to enhance pharmaceutical development, manufacturing, and quality management.