Inside FDA's AI-Based Established Conditions Pilot

By Matthew Pillar, Editor, Bioprocess Online
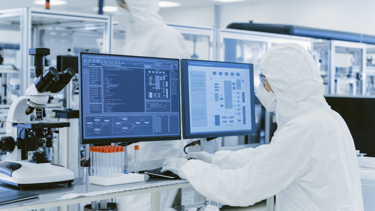
In lieu of a physical meeting, the FDA/Xavier PharmaLink 2020 conference opted to go virtual, as did most conferences during the busy spring show season. PharmaLink did not go off without a hitch, despite Xavier’s incredible and honorable 11th-hour work switching conference rooms to chat rooms and coordinating session slots with speakers dotted around the globe. Such is the case when you’re trying to connect thousands, live, via one very long, very thin wire.
One victim of an IP communications tech glitch was CDER Operations Research Analyst Geok Yan Loo, whose session on predictive analytics in quality operations suffered some significant audio disruption at go-time back in March. True to its good form and to its credit, Xavier and Loo resurrected that presentation for a gaggle of event registrants on April 1st. This time, the presentation titled CDER’s Approach to Innovation and Use of Predictive Analytics was well-presented and well-received.
For her part, Loo works on the front lines of CDER’s Division Of Quality Intelligence, a section of the Office of Pharmaceutical Quality (OPQ)/ Office of Quality Surveillance. Her presentation detailed results of a pilot program conducted by the OPQ that applied predictive analytics and machine learning algorithms to historical (FY17-FY19) Field Alert Report (FAR) data from 343 participating facilities producing therapies at the ANDA and NDA stages.
The goal of the pilot was to determine whether the proactive identification of these data signals could assess pharmaceutical quality systems (PQS) effectiveness.
By way of a quick primer, predictive analytics can be loosely defined as the use of data, statistical, and machine learning techniques to identify the likelihood of future outcomes based on historical data. The concept has been widely adopted by other industries, notably retail and financial services, and it manifests itself in those applications in our everyday lives. When you visit a retailer’s web page and see ads for items relevant to something you recently purchased or searched for on Google, or when you receive a fraud alert from your credit card company after purchasing an expensive item while on a business trip far from home, you’re experiencing the outcome of a computer algorithm that very quickly assesses your historical data inputs, notices a trend or an anomaly, and triggers the ad or alert you see.
It’s a four-part action driven by artificial intelligence to collect, explore, and model data before visualizing the results, and it’s a concept that’s building steam in pharma.
Results Of The FDA Pilot
In her presentation, Loo described how the FDA EC (Established Conditions from ICH Q12) Pilot Program leveraged AI to build a framework for management of post-approval CMC changes in a more predictable manner to reduce the regulatory submission burden for the Agency and for applicants regarding proposed changes to Established Conditions, which are legally binding, and changes to which require a submission to the regulatory agency—high-risk changes require prior approval, while low-risk changes require a submission within 30 days of the action.
The pilot program further sought to identify the measures of a mature quality system among participating organizations. As submissions were received, they were classified by lifecycle stage. Loo pointed out that in this exercise, submission of FARs—under normal circumstances, an exercise best avoided altogether—were actually a good and important thing to the efficacy of the study and the long-term optimization of PQS measurement. Those reports—mandatory within 3 days of receiving information on a possible or actual product quality issue—provided the data lifeblood for the study.
Loo’s presentation went deep on the algorithmic science and controls used. The slides describing those controls can be accessed here. The outcome of the pilot revealed trends in root causes and CAPAs (corrective and preventive actions) taken to establish best practices, including a summary of the best performance models for PQMS metrics, replete with median investigation time and percent of root causes identified. From there, FDA is able to drill down into the data for deeper analysis of facility performance. Not all of the findings were intuitive. The pilot program found median investigation time averages of 30-35 days in FYs 2017 through 2019. Where a short median investigation time often indicated promptness, for instance, long median investigation times didn’t always suggest a laggard response, but rather, a desirably thorough investigation of a complex problem. Root cause identification in those same years varied between averages of 75 percent and 78 percent. Those findings were more intuitive—the more root causes identified, for instance, the more effective the organization’s standard operating procedures were deemed. Repeat deviation metrics, which varied between 29 percent and 33 percent during the studied years, assessed the organization’s ability to mitigate. Finally, CAPA retraining (the number of CAPA that only listed “retraining” as the corrective action), which bounced between 24 percent and 67 percent, is an important indicator of the possibly poor investigations that are not likely to avoid future failure.
The pilot program illustrated the potential power of machine learning as a tool to improve PQS effectiveness and benchmark our industry’s aggregate performance, and as is always the case in AI-driven data play, more data will only improve modelling as the model is trained. Acknowledging this as the case, Loo suggests that the pilot program is likely to be broadened. CDER is continuing to gather feedback, and its next steps are being determined in concert with other FDA offices.
In the meantime, the pilot program illustrates just how powerful and competitively advantageous it could be for pharma organizations to bring machine learning in house to conduct their own internal quality and risk reduction assessments. What’s the first step toward that? I’d suggest sharing Loo’s slide deck with your resident data scientist.