Considerations For AI Use In Biomanufacturing

By Tyler Menichiello, Chief Editor, Bioprocess Online
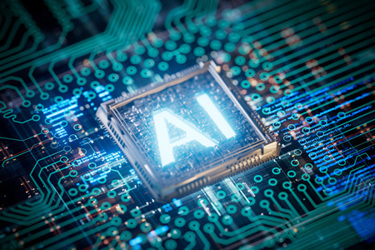
Artificial intelligence (AI) and machine learning (ML) are the buzzwords of the decade, and they’re often accompanied by a myriad of lofty promises — but what do they really mean for biopharma? How can these technologies be adopted to improve biomanufacturing processes, testing, and quality? And what should companies consider when adopting these technologies?
These are the questions that guided this month’s Bioprocess Online Live event, which featured expert panelists Deenadayalan Bakthavatsalam, Ph.D., CSO at rBIO, Tinglong Dai, Ph.D., Bernard T. Ferrari Professor at the Johns Hopkins Carey Business School and co-chair of the Johns Hopkins Workgroup on AI and Healthcare, and Tugce Martagan, Ph.D., associate professor of industrial engineering and innovation sciences at Eindhoven University of Technology.
During the hour-long event, our panelists discussed different use cases for AI and shared their thoughts on the adoption of these technologies.
AI/ML: The New Tools In Biomanufacturing
To start the conversation, I asked our panelists to define AI/ML and explain the ways these tools are being used in biopharma. “Artificial intelligence, to me, is the simulation of human intelligence by using machines,” says Dai. He likens the way these machines learn and improve over time to human babies, saying they’re analogous.
“They observe adults and imitate, so that’s learning,” he says. “They’re learning to see things better, hear things better, say things better — that’s perception. And then they become better and better decision makers. At a certain point, they start to make their own decisions and become autonomous agents, so that’s cognition. I think that’s the essence of AI.”
In the context of biomanufacturing, Bakthavatsalam considers AI as “a simple analytical tool to predict, enhance, and facilitate quality outcomes of a given process.”
AI technology can be both predictive and prescriptive, Martagan says. It can be used to build prediction models, as well as optimization models to help improve quality control and automation in biomanufacturing. She touches on the variety of applications for AI in biomanufacturing, both at the process level and at the facility level.
One example Martagan refers to, at the process level, is fermentation. When used in fermenting, AI can help define the golden profile (the ideal profile for the whole process), and if the process deviates from this golden profile, it can tell you how to bring it back, she explains. At the factory level, she says, AI can help with planning, scheduling, and inventory control. “Cost efficiency, robustness, automation — they are all possible with AI technologies.”
Industry wide, there’s already been some amount of integration into equipment monitoring (e.g., for predictive maintenance). And, as anyone who reads headlines knows, AI has already penetrated the drug discovery space. It’s being used in some R&D sites, especially at the chemistry level, Bakthavatsalam tells us, to help researchers learn and better understand the complex behavior of biological systems.
Keeping Humans In The Loop
The wider adoption and use of AI in biomanufacturing is inevitable, according to Bakthavatsalam, but there are still some areas where human intervention will remain essential. Creative problem solving and ethical decision making are two examples he points to. Sure, AI can analyze data and make recommendations, but when decisions warrant ethical considerations (e.g., decisions tied to patient safety, regulatory compliance, and environmental impacts), “human intervention is pretty crucial,” he says. It’s important to remember that AI is merely a tool, and when it comes to designing instruments or generating original ideas, there’s no substitute for human creativity.
Highly skilled labor is always going to be part of the biomanufacturing industry, Bakthavatsalam says. While AI can be used in the predictive maintenance of biomanufacturing equipment, the actual repair and maintenance of equipment will still require manual intervention.
The industry is already highly automated, but a lot of manual effort, attention, and care is needed to ensure quality, compliance, and safety, Dai says. “I think that part — that attention, that care, that human oversight, that detective skill, that ability to connect dots — that expertise is not going to be replaced by AI anytime soon,” he explains. In fact, he thinks the need for expertise will likely increase. As AI innervates the industry, more SMEs will be needed to ensure its accuracy and correct its mistakes.
According to Dai, this illustrates a paradox surrounding AI: The people who can benefit the most from it are the people who do not need it (i.e., SMEs). These experts can use AI reliably and safely because they’re qualified to recognize and correct any blatant mistakes it makes, whereas novice users may put too much faith in the technology’s decision making. As more facilities adopt AI, a loss of human expertise is “almost inevitable,” he says cautiously. Therefore, the industry must be careful to preserve this expertise to maintain a measure of human oversight in the future.
Training AI Models
AI models are only as good as their training. However, just like human intelligence, the way these models learn and make decisions can be somewhat of a mystery, even to the developers. This black box problem presents some challenges when it comes to using AI in biomanufacturing, Bakthavatsalam says, because transparency is paramount in this regulated industry.
One way to mitigate the black box problem is through a hybrid model approach, Martagan says, which combines symbolic AI (rule based) with non-symbolic AI (ML and deep learning). The symbolic AI helps explain the logic behind certain decisions made by the non-symbolic AI, making the model more understandable (transparent) and explainable to regulators.
However, Dai sees the industry’s challenge as less about avoiding these black box models and more about making sure the models work in the real world. The best way to ensure their performance is by training models on good, quality data (data that reflects real-world applications and decision-making environments). If you train models on data that isn’t realistic or has features not found in real-world environments, then you risk ending up with a good model that performs well in training but very poorly when applied in reality.
Training AI is like helping a child study for a math test, Dai says. If you tell the child the answers to every question, they will do really well in practice, but when it comes time to actually take the exam, they will probably fail because they won’t have any answers in front of them. The training environment (studying with the answers provided) did not match the real-world environment (taking the test). Similarly, unrealistic or incomplete training data and a misguided approach to training can yield artificially high-performing models that will not do well in the real world.
Data Security And Silos
When it comes to adopting AI in biopharma, data security is a legitimate concern. “You always want to protect your data, because that’s part of your resources,” Dai says. However, it’s important to weigh the risk of data security against the value these technologies provide, he says. He points to consumer technologies like iPhones and Alexas, which collect more user data than people can even comprehend. And though consumers don’t exactly know what Apple or Amazon does with all this data, they happily surrender it for the practical utility of these devices.
While every biopharma company can benefit from adopting AI, there is no one-size-fits-all model. Bakthavatsalam refers to this long-tail problem in AI: Every company’s needs and use cases vary, which will result in individual silos of applications. Even within biomanufacturing, there are multiple verticals that operate independently, he says (e.g., regulatory aspects and vendor supply chains). This presents interoperability challenges, which he compares to the early days of electronic health records. Moving forward, he says, some aspects of these silos can be shared (without sharing IP) to benefit the industry more broadly.
Addressing Resistance
It’s clear that AI isn’t going anywhere, and if companies don’t learn how to utilize these tools, they may fall behind in the long run. However, it would be foolish to assume industry-wide adoption and use will happen seamlessly or overnight. Regulatory bodies are going to play a key role, says Bakthavatsalam.
“I think even before moving into advanced AI models, we need to step back a little bit and also understand how to collect good quality data to build these models,” Martagan says. We need to invest in helping people understand the potential of AI, she argues, and build good data structures, data models, and standardization techniques to enable these AI-driven initiatives.
On the topic of adoption, Bakthavatsalam stressed the importance of addressing workforce resistance. “The most important factor of adoption of AI is providing education, training, and support to the workforce,” he says. He recommends demonstrating the value of this technology with pilots and case studies. It’s important for workers to understand that AI is not replacing them — rather, it is supporting their efficiency and reducing their workload. If the industry fails to address these concerns and educate workers how to use these technologies, “manufacturing will not survive,” he says.
Much more was covered during this event than I can include in this summary, so I encourage you to watch the full recording, which is offered on Bioprocess Online for free thanks to the event sponsor, Atum.