Computational Biology Speeds The Path To Clinical Studies

By Matthew Pillar, Editor, Bioprocess Online
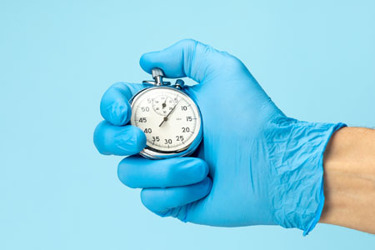
I had the pleasure of meeting Matthias Alder and Eric Richman back in August when I was doing some research on the advance of artificial intelligence and computational biology in biopharma. At the time, Richman was CEO and Alder was COO at Gain Therapeutics, a company leveraging AI for drug discovery in its SEE-Tx target identification platform and building on that discovery by developing a preclinical pipeline of therapeutic candidates in Parkinson’s and lysosomal storage disorders including Gaucher Disease, GM1 Gangliosidosis, Krabbe disease, and other difficult-to-treat disorders characterized by protein misfolding.
Since then, Alder has assumed the CEO role at Gain, where Richman remains as senior adviser and board member. More recently, I caught up with Alder for insight into the company’s pragmatic application of its computational discovery platform as it readies its Parkinson’s program for clinical trials in 2023.
From Computational, Predictive Modeling To The Clinic
“We're not fixing the gene itself, but we’re acting at the very beginning of the disease cascade by stabilizing an enzyme that is expressed by a mutated gene,” explains Alder. “Because the gene is mutated, the enzyme is misfolded. We address that misfolding and restore the function of the enzyme.”
The therapeutic compounds the company is developing are small molecule, but the foundational technology it’s using in drug discovery has broad applicability. Alder says it’s capable of reducing a decade-plus drug discovery process to three months by replicating access and targeting of the 90 percent of proteins that are currently considered undruggable. While he’s bullish on the technology, he acknowledges that artificial intelligence plays a supporting, not leading role in the platform. “We take a structural, physical approach, as opposed to a purely AI approach, which would require a lot of preexisting data,” he says. “We can look at these proteins that have never before been targeted, where there’s very little known about binding sites, and investigate their response to new therapies.”
Still, Alder isn’t naïve enough to envision an in-silico-to-clinical leap for newly discovered therapeutics, even if the “artificial” intelligence points to a winner.
I referenced George Yancopoulos, scientific founder and chief scientific officer at Regeneron, who, speaking recently on a panel at the Galien Forum, said he disputes the "I" in AI, because "there is no intelligence there." Yancopoulos offered a soundbite-worthy analogy when he posited that there’s no existing AI system in the world that could figure out that peanut butter and chocolate taste good together.
Alder didn’t disagree. “We’re very attuned to the fact that on the computational side, the predictions are just that. They are good ideas, predictions of what might happen, but you have to test those predictions in real life experiments.” That, he says, happens through experimental methods assays to determine whether the platform’s prediction turns out to be true in real life. For that purpose, Gain turns to a lab operation that’s integrated with its discovery platform team. Computational biologists and lab scientists sit side-by-side in the company’s Barcelona facilities, a juxtaposition that enables a feedback loop. “Virtual hits are synthesized and taken into physical experiments, where the properties of the molecules we’re developing are analyzed, optimized, and run back through the screening process,” he explains. “It’s an iterative cycle, looking at analogs of that molecule to improve it and optimize its drug-like properties. There's a lot of medicinal chemistry work that needs to be done. What pops out of the platform is not the molecule that then we can simply take into testing.”
Instead, the computational platform enables an understanding of the shape and size of the binding pockets on target proteins and the specific molecular interactions that need to occur between the therapeutic structure and the protein’s surface. Those “binding hotspots,” as Alder calls them, help developers understand what the molecule needs to look like and how it can be optimized—and importantly, what physical structures simply won’t work. “Understanding these physical properties is absolutely key to rational drug design, and we’re demonstrating that value in our Parkinson's program,” he says.
More Machine Modeling Than Machine Learning
In response to Yancopoulos’ derisive take on artificial intelligence, Alder points out an important distinction of Gain’s platform. “We're not relying on a lot of preexisting structural information and data in an attempt to teach the computer to recognize similar things in different places on different proteins,” he says. “We're physically scrutinizing the surface of the protein to identify the binding sites where there was no preexisting data whatsoever.” In this and other AI approaches in general, he says, the human factor is required to assess and understand what the machine model is demonstrating. Generating 3-D structures of 1-D sequences of proteins taken from a database moves the ball downfield. It takes human assessment and understanding of those models and their predictions—and the therapeutic molecule’s attributes and reaction to the protein and its predicted binding sites—to get the ball into the pre-clinical red zone. “Particularly with novel, allosteric binding sites that are not previously known or tested, you need that human experience,” he says.
Still, as Gain leans closer to clinical studies, Alder says the company will explore applications for computational applications in its trial efforts. The company is currently evaluating IT-based clinical trial tools that he believes could expedite and simplify clinical studies, specifically in the context of patient selection. “Clinical studies often fail not because the product doesn't work, but because it’s being tested in the wrong patient set. Using data sources to help select the trial population could be very valuable,” he says. The Parkinson’s patient subset that Gain seeks are those with mutations to the GBA1 gene, so identifying biomarkers is central to the effort. The company is working on a grant from the Michael J. Fox Foundation to identify biomarkers that can be used as surrogate endpoints in clinical studies.
The company’s computational platform, says Alder, also represents an opportunity for application beyond its own pipeline. “We’re building up the business development side of the business in an effort to partner with pharma companies to fully exploit the platform, which is therapeutic agnostic,” he says. “As a small biotech, we can barely scratch the surface of its capabilities.”
Learn more about Gain Therapeutics at www.gaintherapeutics.com.