AION Labs' Challenge To AI Drug Development Innovators

By Matthew Pillar, Editor, Bioprocess Online
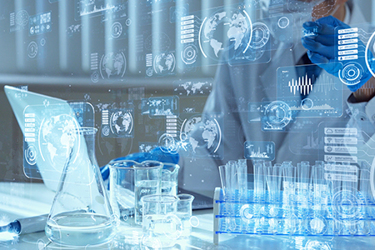
Mati Gill’s exposure to the power of pharma collaboration can’t be overstated. During his 11 years as COO of the Global Legal Group, and later, head of government affairs, corporate & international markets at Teva Pharmaceuticals, he was exposed to virtually every aspect of the business. When Teva made a concerted effort to build its corporate innovation strategy to strengthen its development platforms and pipeline, he was an undisputed pick to lead the exercise in support of Teva R&D for the Israeli pharma titan. As he helped make inroads with Israeli’s academic and emerging life sciences ecosystems, a promising opportunity began to reveal itself and became the focus of his work: The roles of computational biology and artificial intelligence (AI) in drug discovery and development.
Gill found the concept nascent among the next generation of innovators but hampered by the pharmaceutical industry at large.
“The people who understand machine learning and AI typically come from the high-tech industry. They aren’t experts in pharma, and the pharma industry aren’t experts in AI,” says Gill. Therein lies problem number one; the solvable, though effort-intensive, challenge of interdisciplinary and interindustry tech/science collaboration that leads to common understanding of each player’s problems and solutions.
"The research questions we choose are challenges that, if addressed, we believe could be the nexus for a startup company that can develop the computational, AI-based technology to either discover new drugs or develop platform technologies that enable drug development."
Problem number two gets a bit stickier, and trickier, to solve: overcoming skepticism and secrecy.
When a life sciences-focused tech company, or a tech-focused life sciences company, for that matter, approaches big pharma to pitch what it considers a great AI-driven solution to a specific problem, big pharma has historically bristled. Validation happens in the clinic, after all, and comes with a hefty price tag. It’s sometimes challenging for decision makers to accept a computational platform’s direction when they don’t fully understand how a conclusion w.as reached. And, pharma holds the data, which is typically not made available to external technology developers.
Mighty Levers Move Mighty Mountains
Despite those challenges, the promise of AI-enabled drug discovery and development cannot be contained. That’s perhaps nowhere as palpable as it is in Israel, a country whose economic standing is rooted firmly in its high-tech, and more specifically, AI-driven contributions to industries around the globe. As the collaboration Gill worked with then Teva colleagues to fuel began to grow its own legs, its mission began to outgrow Teva’s own R&D initiative. What began as a consortium grew into a complex alliance with several global pharmaceutical players—AstraZeneca, Merck, Pfizer, The Israel Biotech Fund, BioMed X Institute, and of course, Teva Pharmaceuticals—as well as the high-tech king of kings, Amazon Web Services (AWS). In the collaborative spirit, a venture creation model began to take shape, crafted by representatives of all these alliance members. AION Labs was born of it, and Gill was appointed its CEO.
Solving The Challenge By Issuing A Challenge
Here’s how the new venture is working to nurture the development of AI in drug discovery and development. Each of the six AION Labs alliance members have an appointed, ranking representative on the organization’s board of directors, and a separate representative on its investment committee. The representatives from the pharma companies — AstraZeneca, Merck, Pfizer, and Teva — work with their respective R&D groups to identify and bring forth the top research challenges they face. From that list of challenges, the board selects one at a time—four-to-six per year over an initial five-year horizon—to address in a global, crowdsourced call for applications from individuals who believe they can affect the challenge with an AI- or machine learning-focused approach. “The research questions we choose are challenges that, if addressed, we believe could be the nexus for a startup company that can develop the computational, AI-based technology to either discover new drugs or develop platform technologies that enable drug development,” says Gill.
Fifteen finalists are enrolled in a five-day bootcamp, where their ideas are supported, tested, and matched with two to three complementary finalists, who then pitch their ideas to the investment committee in what Gill describes as akin to a biopharma “Shark Tank.” “Winning teams of two to three individuals come together, often without any prior knowledge of one another, to form a research partnership that receives a multi-year funding package to develop their solutions in what we call a venture creation track,” explains Gill. That package includes up to $2 million of runway funding over four years, wet and computational lab and office space at AION Labs, guidance from R&D mentors in the team’s specific discipline, a dedicated solution architect from AWS to assist with technology and cloud development, and venture formation/management/R&D consultation and mentoring from AION Labs and BioMed X. The projects are funded by a mix of the participating pharmaceutical companies, The Israel Biotech Fund, the Israel Innovation Authority and Digital Israel.
A Unique Emphasis On A Pre-Competitive Environment
When Gill was appointed to lead the new AION Labs initiative, he then immediately set his sight on recruiting the chief technology officer for the lab as his partner to oversee the science and technology aspects of the ventures the Lab would support. He found that CTO in Dr. Yair Benita, whose Utrecht University Ph.D. in bioinformatics and Harvard Medical School/Mass Gen post doc in computational biology laid the foundation for experience well-suited for his new role. Dr. Benita was previously computational biologist and principal scientist at Merck, head of computational research and discovery at Compugen, and head of science operations at CytoReason.
“I’m a licensed pharmacist, but I quickly learned that lab work wasn't for me, and I drifted towards computer science,” says Dr. Benita. “I ended up combining the two at a very early point in my career, as I saw an opportunity for the process of drug development to be much more data-driven. Early on, it was trial and error all the time, it was very expensive, and very laborious. As an industry we’ve gotten better, but we’re still very, very far from where we should be,” he says.
Getting to where we should be, says Dr. Benita, requires changing the status quo. “Academia is the R&D engine of pharma in general,” he says. “Pharma reads papers and tries to develop drugs for diseases using mechanisms we understand, but most of that academic work is done on animal models and cells.” That’s problematic, he says, in that most of those development efforts fail when attempts are made to translate them to humans. Those failures don’t surprise him. “We’re trying to solve problems in models that aren’t real. The human disease is unique,” he says.
At Compugen, Dr. Benita led a team that leveraged computational biology to discover a new immune checkpoint in oncology. That effort made its way to the clinic, proved the conceptual promise of data-driven discovery, and fueled his penchant to move forward on the computational biology path.
That’s when he moved to CytoReason, which owns one of the world’s largest libraries of human molecular data and a platform to make that data accessible and useful to clinical and pre-clinical drug development programs. He worked with multiple big pharma organizations that contracted the firm’s library to inform their drug development efforts. But there was still a problem. “We made a great impact, but I realized that it's very challenging to implement the results that come out of data analytics in pharma. They see the results, but they're not sure what to do with them. They want to go with their own gut instincts. After four years at CytoReason, I reached the conclusion that the customer /provider relationship is not great. I prefer to engage as a partner,” he says. The opportunity here at AION Labs facilitates that partner approach. We’re working with multiple pharma companies in a pre-competitive space as partners, not customers, where the goal is to develop new technologies, as opposed to helping specific pharma companies solve minute problems in their respective pipelines. Our pharma partners recognize that there’s a big problem they want to solve, they’re willing to fund it, and they’re willing to share their data.”
Gill and Dr. Benita, for the record, don’t hold the laggard pace of digital adoption against the pharmaceutical industry. “Pharma is one of the last industries to undergo a digital revolution, and it's not by chance,” says Gill. “We're dealing with the most important and sensitive elements of life—our health and our bodies. It makes sense that we're a bit more conservative in the way we approach disruption of the way we do things, particularly when those disruptors have yet to be fully developed, proven, and scaled up.” That’s why they believe the AION Labs approach—inciting innovation that results in new discovery and development technology being built from the ground up and with support from big pharma—as opposed to being sold to big pharma—is the best path forward.
“We’re putting together teams of the best people available to solve challenges, and giving them everything they need,” says Gill. “They don't need to worry about the investors or the money or the guidance or the data. They can just focus on solving the problem.”
Challenge #1 Accepted: Computational Prediction Of The Antibody Sequence
AION Labs launched its first challenge on October 19, 2021: Computational Prediction of the Antibody Sequence. “The pharma companies provide the protein, and the challenge is to predict the antibody that will bind it,” explains Dr. Benita. “Currently, antibodies are screened. You must send millions of antibodies through assays. It's a very difficult process that takes two to three years,” he says.
An in-silico prediction of the antibody sequence, says Dr. Benita, is a perfect problem for AI. “Pharma has a lot of screening data on antibodies. They have the data sets to learn from. They have the capabilities to test. It's really tough to do, so it's a big challenge, but it also has a big potential impact.”
The call for applications for this challenge will remain open for 8 to 10 weeks. Details can be found at www.aionlabs.com.