Advocates For AI In The Laggard Life Sciences

By Matthew Pillar, Editor, Bioprocess Online
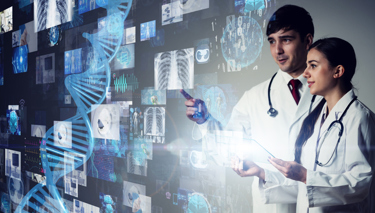
A team at Xavier Health is taking an unbiased lead on the adoption of artificial intelligence in the life sciences. They’ll present progress on their daunting task this month.
The Xavier AI Summit 2020 will set the stage for Xavier’s AI in Operations team (www.xavierhealth.org/aio-team) to present on its progress developing a roadmap and a maturity continuum for life science companies seeking to leverage AI in their operations. The summit takes place August 24, 26, 28, and 31 (Virtual Main Summit Sessions) and Sept. 2-3 (Virtual Summit Workshop).
The team began to take shape in August of 2019 as that year’s Xavier AI Summit drew to a close. “We had conducted some interviews on AI implementation progress and challenges previous to the event,” relays team member Cynthia Ipach (Compliance Insight). “Lack of a roadmap was a recurring theme.” Once a company decides to invest in AI, she says, “Multiple departments tend to jump on board with projects, but no one is taking all business requests and creating an AI strategy for company as a whole.”
Today’s AI In Operations Team is an “organized, cross-industry discussion group of FDA officials and industry professionals working to increase the predictive assurance of product quality across all operations (i.e., manufacturing, quality, supply chain, etc.) through the power of AI.”
Ipach and fellow team member Kip Wolf (Tunnell Consulting) recognized that a lack of coordination and strategy could hamstring the value of AI investments by life sciences organizations, many of which aren’t well-heeled with corporate-level IT oversight resources. Together, they lead an AI In Operations (AIO) Team subcommittee dedicated to building an AI implementation roadmap that’s purpose-built for life sciences organizations.
That's no easy task. More a tech-enabled concept than a tech in its own right, AI doesn’t readily belong to any one department or discipline in the biotech org chart. There’s no singular, absolute, universal ‘starting point’ for its adoption. Rather, its use cases—and the return or value those use cases present—are as variable as the March winds. Hence the often project-specific initial interest in the concept.
How on earth do you map such a thing? “From the outset, our approach was intentionally nonbinary and nonlinear,” explains Wolf. “We interrogated multiple process groups that have adopted AI, their algorithms, the accuracy of their data, and the bias in data that affect their outcomes,” he says. “We then assign values to multiple measures of maturity on a Likert scale of one to five.” Wolf admits the approach isn’t perfect and isn’t finished, but he’s confident it delivers a baseline the industry can use today.
The “we” in the team has been meeting twice monthly since last fall and includes Lacey Harbour (Lima Corporate), Toni Manzano (Bigfinite), and Sundar Selvatharasu (Sierra Labs). The “life science industry pros with an interest in AI” that the committee aims to serve aren’t limited to any one sector. While the dialog began in the context of medical devices, Ipach and Wolf say they’re adamant about ensuring the fruits of the committee’s labor are applicable to providers, sponsors, manufacturers, and anywhere in the lifecycle, from discovery to commercialization. “Whether you consider yourself a biotech, a pharma, a cell and gene company, a vaccine developer, a med device manufacturer, it doesn’t matter. There are use cases for AI in your organization,” says Ipach.
AI Use Cases: Wherever Data Can Be Interrogated
There are so many potential applications for artificial intelligence in biotech, in fact, it’s hard to put your finger on a “killer app” that stands above the rest. “Our Xavier AI Development Team in interested in exploring any process that requires the interrogation of data,” says Wolf. They’re using algorithms to uncover root cause in a number of quality control applications, such as deviation analytics to analyze root cause of manufacturing defects and variations in batch results, for instance. This particular application is especially important as manufacturing process automation catches on, because it speeds up the analysis of full batch data that, in continuous and automated environments, is only getting fuller.
Programmatic analysis of capacity planning for labs is another likely application Wolf points to. “Live connections to lab equipment to monitor use and resource scheduling is an AI-based concept that would drive computational analysis for lab capacity in real time for forecasting purposes,” he says. That’s a powerful efficiency enabler for any capacity-constrained business, from a biopharma to a CDMO and beyond.
Internal and external (agency, partner, third party) inspections and audits are also more efficient using AI, says Wolf. “Automating the computational analysis of data scraped from the Web can reduce audit and inspection costs, and promote more frequent assessment of compliance.”
Slow Adoption, Despite Benefits Untold
Despite these and countless other applications for computational analysis in life science industries, Ipach and Wolf—both well-established consultants in the space—report laggard adoption. “Among the groups we’ve surveyed that are highly organized and have a handle on AI, we see usage happening in multiple different ways across many disciplines in the business,” says Ipach. “Then there are the beginners who are determining how to clean their data, how to choose and configure their algorithms, and whether to buy or build. We don’t see a whole lot in between.”
Wolf concurs. “Life sciences are slow to adopt new technology,” he says, in the exact tone you’d expect to hear someone saying something that’s been said and heard for years. “In 1995, I’d talk about QMS and TQM and ISO 9000 and 9001, and I would be told by life sciences clients that those don’t apply here. But they did, and it took 20 years for them to become ubiquitous. Now, as then, we find ourselves decades behind the curve.”
Wolf points to what he terms small pockets of innovation where that trend is being bucked. The likes of Pfizer, Astra Zeneca, Merck and GSK, he says, are bearing out use cases. “In companies like these, we’re seeing three or four data scientists killing it on small projects, proving out their business cases by saving big money where AI is applied, and putting that saved money into the addition of talented people.”
A Labor Of Learning
When Wolf and Ipach take the stage this month to present their work at the Xavier AI Summit, it will mark the beginning of the next chapter on the AI In Operations team’s journey. “Next year, we’ll look at our maturity model and further define how to progress from one level to the next, complete with specs and risk analysis,” says Ipach.
It’s hard and unprecedented work, with many moving parts and many stakeholders, and Wolf, Ipach, and team are doing it all for free. “It’s an exciting project to be a part of, because it’s necessary work that’s beneficial and available, open source, to the entire industry,” says Wolf . “Where other groups might come at this from a commercial perspective and thus, refuse to talk to one another, we’re approaching it from a nonproprietary, academic perspective with altruistic intentions.”
Learn more at Xavier’s AI Summit, August 24, 26, 28, and 31 (Virtual Main Summit Sessions) and Sept. 2-3 (Virtual Summit Workshop).