Seeq Corporation
1301 2nd Avenue Suite 2850
Seattle, WA 98101 US
Seattle, WA 98101 US
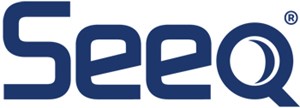
Company Overview
Seeq® enables process manufacturing organizations to rapidly investigate and share insights from data in historians, IIoT platforms, and database web services as well as contextual data in manufacturing and business systems.
This website uses cookies to ensure you get the best experience on our website. Learn more