Using AI And ML To Gain Insight Into Optimal Glycoforms

By Tyler Menichiello, contributing editor
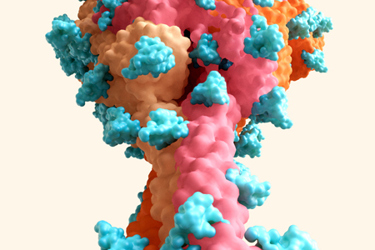
At this year’s BioProcess International (BPI) U.S. West conference, I attended a keynote presentation titled “Advances In Machine Learning For Cell Line Development To Predict Better Clones, Reduce Timelines, And Experiments” by Nathan Lewis, Ph.D., professor and Georgia Research Alliance (GRA) Eminent Scholar at the University of Georgia. Lewis talked about the research his team is doing to investigate how post-translational modifications like glycosylation can affect the safety and efficacy of biopharmaceutical products.
It’s important to characterize glycosylation for cell line development (CLD), according to Lewis. Yet, a deep understanding of glycosylation and how different glycoforms affect product safety and efficacy is limited. That’s why Lewis and his team used machine learning (ML) and hybrid modeling to analyze these characteristics and identify optimal glycosylation patterns. Through AI analysis and predictive modeling, his team found that protein sequence and structure play a role in determining glycosylation.
I had the chance to connect with Dr. Lewis after his talk. The following is his response to my question about his research (edited slightly for clarity).
What would you like to tell our audience about your research and your talk today? How can they apply this to their own product development?
Oftentimes, when we're thinking about making biologics, we're thinking about the easy stuff to measure, like the sequences of the genes and the proteins. We often don't consider enough the opportunity in the post-translational modifications. Glycans, for example, sometimes can significantly change the function, safety, and efficacy of a drug. This has been more of a challenge in the past — more of a liability, in biologics.
Our work that I presented today was about how we can turn it from a liability into an asset. We can actually go in and either use engineered cells to control the glycosylation from the outside in — trimming them down to the structures that we want, which we can identify using AI and other methods — or more recently, we’ve figured out how to engineer the glycans from the inside out by controlling the glycans that can be placed on a protein by engineering the protein itself. Now, we’re at a point where there are a lot of opportunities in drug design and manufacturing to get better, more potent drugs.
Oftentimes in CLD, we're trying to select for clones that have the best glycosylation, or we’re potentially trying to boost various attributes of the glycans. The latest work on how the protein structure influences the glycan structure gives insights into how much effort we should put into trying to optimize glycosylation. Sometimes, it's going to be already constrained by the protein structure itself.