This Digital Twin Approach Aims To Optimize ATMP Production
By Rene-Pascal Fischer, scientist and software architect, Fraunhofer IESE, and Anna Dünkel, Ph.D., cell and gene therapy development, Fraunhofer IZI
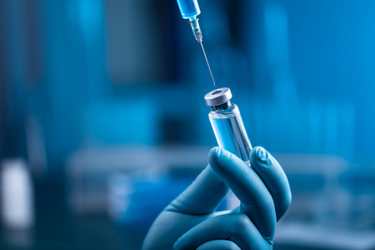
The most compelling factor driving the development of allogeneic advanced therapy medicinal products (ATMPs) is the potential to provide a sufficient quantity of cell products to a large number of patients from a single source. The implementation of robust protocols for cell expansion guarantees the production of consistent and reproducible doses for therapeutic applications, thereby ensuring the quality and efficacy of the final product.
Scalable manufacturing processes for allogeneic ATMPs impact production costs through, for example, efficient cell expansions, making them more cost-effective compared to patient-specific treatments like autologous CAR T cells.1 Currently, manufacturing therapeutic cells includes labor-intensive automated, semi-automated, and manual processes. The objective is to achieve efficient increases in cell yields through the use of cost-effective expansion protocols, while addressing various challenges, including the availability and quality of starting material, robust cultivation protocols, and the transferability from small- to large- scale, as well as from research to GMP manufacturing.
Consistency in product quality remains a focal point, as standardized cell expansion is essential to maintain uniform product quality across different batches.
The expansion of primary cells often requires compromises in optimal cultivation conditions or cell yields to meet GMP compliance. Utilizing cultivation data collected during expansion processes can improve outcomes by incorporating predictive quality models into the manufacturing process. This approach is being explored through the use of digital twins in the Fraunhofer Lighthouse Project RNAuto, which aims to digitally document various processes and product data as well as quality assurance information, among them an allogeneic cell expansion process. Digital twins can transform this data into a digital product passport, ensuring consistent product quality and facilitating compliance with Pharma 4.0 standards. By integrating predictive process monitoring, digital twins can optimize cultivation conditions and yield, ensuring that high standards of GMP compliance are maintained.
RNAuto Initiative Rooted In Open-source Digital Twins Platform BaSyx
The current landscape of automated production control in cell manufacturing is heavily influenced by the development and integration of digital twins.2 These advanced models are crucial for predictive and automated management of the manufacturing process. Within the scope of the RNAuto project, digital twins play a key role in automating documentation and standardizing technologies to ensure consistent quality and efficiency. This initiative builds on established Industry 4.0 standards, particularly the asset administration shell (AAS), which emerged from the German Industry 4.0 platform as a European standard.
The Industrial Digital Twin Association (IDTA) is at the forefront of standardizing submodels for various applications, including asset control. The importance of these efforts is underscored by the 2021 Impulse Report3, which highlights the need for developing value-creation architectures as a critical research area for successful Industry 4.0 implementation. This includes the networking of companies, standardized data exchange, and the provision of digital services. Key areas of focus are efficiency improvements, data analysis capabilities, and fostering innovation, interoperability, cost reduction, customer satisfaction, and adaptability.
In production planning, decision support systems that utilize mathematical models and simulations have been in use for years.4 Similarly, mathematical and artificial intelligence (AI)-based models have been developed for reactions in bioreactors and are already part of automation systems. Since 1997, AI has been proposed to enhance control accuracy and as a noninvasive technology.5,6
Since 2016, the open-source middleware Eclipse BaSyx for digital twins is pushing forward the standards of the Industry 4.0 platform. With over eight years of experience, Eclipse BaSyx provides a technical foundation for asset control, utilizing existing standards such as BPMN. The bidirectional integration with assets through Eclipse BaSyx DataBridge allows for data transformation and mapping between native asset communication protocols and the AAS.
The ongoing research project aims to unify these diverse approaches by aligning data documentation and predictive analytics, paving the way for a more interconnected and efficient manufacturing landscape.
RNAuto Seeks To Increase Productivity Through Predictive Intervention
The Fraunhofer Lighthouse Project RNAuto is aimed at transforming the production of mRNA-based vaccines and allogeneic cell therapeutics through automation and digitalization. Utilizing cutting-edge research, the project seeks to address the pressing need for efficient, scalable, and cost-effective manufacturing processes of mRNA-based drugs. For allogeneic cell therapy, natural killer (NK) cells are used for mRNA modification and upscaling procedures.
To enhance the manufacturing process of allogeneic NK cell therapeutics, the RNAuto project is pioneering the development of a dynamic digital-twin-controlled expansion technology. NK cells, because of their unique biological properties, are ideal for allogeneic cell therapeutics as they do not trigger rejection responses in foreign recipients like T cells do. The primary goal of a predictive intervention in the cultivation process is to increase cell yield and product quality while reducing manufacturing costs related to personnel and resources. This is especially crucial in minimizing cleanroom days and workforce requirements, significantly improving the economic feasibility of ATMP production processes. Intelligent, predictive process control systems accelerate decision-making, allowing for timely interventions in the cultivation process.
A standardized modeling approach to the manufacturing process, directly integrated with Eclipse BaSyx, supports the Pharma 4.0-advocated plug-and-produce concept. This facilitates the efficient and straightforward design and development of scalable, flexible production facilities composed of individual components. To achieve these goals, an initial step involves defining a formal model of cell growth for NK cells. This model describes the dependencies of product quality on the starting materials and production parameters, forming the basis of a digital control system. This control system is fundamental for the automated creation of a digital twin, called “Guard” in this project, that monitors the manufacturing process and intervenes as needed based on predictive models.
Cell expansion is a complex biological process. Therefore, the project scope for the predictive model is limited to a few significant parameters (e.g., glucose and lactate levels) to demonstrate the feasibility of the approach. Initially, GMP-compliant in-process samples for glucose consumption and lactate accumulation during NK cell expansion will be collected and used to derive the predictive model. Establishing a solid data foundation requires small-scale expansion processes where metabolites from cell culture samples are measured. Regular cell count and viability assessments are also essential for establishing a valid predictive model. Eventually, this model will be scaled up to large-scale expansion cultures in a dynamic expansion chamber, also developed within the project.
The digital twins for the cell expansion module, sensors, and process monitoring will be integrated as digital twins to enable process control and monitoring. Orchestration, using standardized process description, is described as a sequence of abstract tasks (e.g., mixing substances) with defined inputs and outputs (e.g., maximum flow rate). Abstract descriptions allow flexibility in choosing specific devices or sensors later, following the concepts of Industry 4.0 and Pharma 4.0. For execution, allowable parameters are manually defined, enabling the Guard to automate checks during production based on the predictive model.
When the process is executed, a digital twin instance is created from the abstract description, referencing the specific devices or sensors being used. The use of digital twins allows for automated matching between tasks and devices, ensuring that all required capabilities (e.g., flow rate range) are met by the executing devices. This instance provides all necessary information for NK cell production and defines the sequence of steps with the selected devices. The Guard not only monitors but also uses current process information to make future predictions, dynamically adjusting the task sequence if needed.
In summary, the RNAuto project aims to bring concepts of Industry 4.0 of individualized manufacturing to the pharmaceutical domain while combining it with a new monitoring and documenting approach to adapt to the needs of the domain itself.
Dated Regulations Raise A Potential Stumbling Block For RNAuto
While the potential benefits of implementing dynamic expansion technology for NK cell therapeutics are immense, there are significant obstacles that must be overcome. One of the primary challenges is the stringent regulatory environment governing cell therapy manufacturing. Current regulations, designed to ensure patient safety and product consistency, do not easily accommodate the predictive and automated interventions proposed by digital twin technology. The integration of real-time data analytics and automated decision-making into GMP-compliant processes raises potential concerns about validation, control, and oversight. Additionally, the complexity of creating accurate predictive models that can reliably intervene in the cultivation process without human oversight partially presents technical hurdles. Ensuring that the digital twins and their associated predictive models maintain accuracy and reliability across different batches and scales of production is one specific research topic as medicine will continue to get more personalized in the future.
Despite these obstacles, the promise of reduced production costs, increased efficiency, and improved product quality through intelligent process control systems makes overcoming these regulatory and technical barriers a worthwhile endeavor. Additionally, the complete automation is instrumental to allowing more patients to be treated with novel therapies with higher chances of success. Thus, engagement with regulatory bodies to develop guidelines and frameworks for the implementation of these novel technologies will be crucial in realizing their full potential.
Until such regulatory adaptations are made, the wide-scale adoption of these advancements will remain limited, delaying the significant benefits they could bring to the field of cell therapeutics.
Conclusion
The pursuit of innovative, scalable, and efficient production processes for allogeneic ATMPs represents a transformative step forward in modern medicine. By harnessing the power of digital twins and integrating them into the manufacturing landscape, the Fraunhofer Lighthouse Project RNAuto sets a new benchmark for precision, consistency, and cost-effectiveness in cell therapy production.
This paradigm shift not only promises to lower production costs but also ensures the delivery of high-quality therapeutic products to a broader patient population. The successful implementation of digital twin technology, predictive modeling, and advanced process control paves the way for Pharma 4.0 standards, where automation and digitalization become the cornerstone of manufacturing excellence.
However, realizing this vision requires the active engagement and collaboration of all stakeholders in the biomedical field. Researchers, manufacturers, and regulatory bodies must work hand in hand to overcome existing regulatory and technical challenges. By advocating for updated guidelines and frameworks that accommodate these novel technologies, we can accelerate their adoption and bring lifesaving therapies to market more swiftly.
References
- Blache, U., Popp, G., Dünkel, A., Koehl, U., & Fricke, S. (2022). Potential solutions for manufacture of CAR T cells in cancer immunotherapy. Nature communications, 13(1), 5225.
- Udugama, I. A., Lopez, P. C., Gargalo, C. L., Li, X., Bayer, C., & Gernaey, K. V. (2021). Digital Twin in biomanufacturing: Challenges and opportunities towards its implementation. Systems Microbiology and Biomanufacturing, 1, 257-274.
- Forschungsbeirat der Plattform Industrie 4.0/acatech. (2021). Impulsbericht Industrie 4.0–Forschung für die Gestaltung der Zukunft.
- Kleijnen, J. P. (1993). Simulation and optimization in production planning: a case study. Decision Support Systems, 9(3), 269–280.
- Kleinstreuer, C., & Poweigha, T. (2005). Modeling and simulation of bioreactor process dynamics. In Bioprocess Parameter Control (pp. 91-146). Berlin, Heidelberg: Springer Berlin Heidelberg.
- Viet, N. D., & Jang, A. (2021). Development of artificial intelligence-based models for the prediction of filtration performance and membrane fouling in an osmotic membrane bioreactor. Journal of Environmental Chemical Engineering, 9(4), 105337.
About The Authors:
Rene-Pascal Fischer is a scientist and software architect at Fraunhofer IESE focusing on Industry 4.0 and its adoption in the pharmaceutical domain. Having started his Ph.D. on validation and certification of processes using digital twins, he is an advocate of the holistic digitalization and the connected enablement of a digital control flow. He is actively contributing to open-source software and multiple research projects for both Industry 4.0 and Pharma 4.0 as well as the ISPE’s Pharma 4.0 community of practice. Contact him at rene-pascal.fischer@iese.fraunhofer.de or reach him on LinkedIn.
Anna Dünkel is a biologist by training, finished her Ph.D. in the field of antibody engineering, and is now focusing on process development of cell and gene therapy manufacturing as a group leader at the Fraunhofer IZI. Together with her team, she is particularly interested in the process design of allogenic cell and gene therapies, automated manufacturing, and upscaling with a special expertise in allogenic NK cell-based therapeutics. The driving force behind her work in the RNAuto project is linking biological research with core aspects of GMP process development and the exploitation of the consortium's potential to combine technical expertise with life science topics. Contact her at anna.duenkel@izi.fraunhofer.de or reach her on LinkedIn.