The Symphony Of Simulation: Mechanistic Modeling And Digital Twins In Biologics
By Life Science Connect Editorial Staff
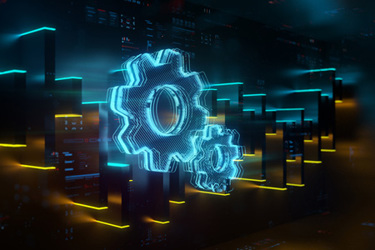
Developing and manufacturing biologics demands a concerted convergence of expertise from chemical and biological sciences, data analytics, and engineering. At the heart of this collaborative effort lies the growing adoption of mechanistic modeling and digital twins, technologies poised to streamline bioprocessing.
In the context of bioprocess development and manufacturing, a digital twin can be defined as a dynamic, virtual representation of a physical bioprocess system. These models, which range in complexity, aim to capture the multifaceted phenomena occurring within a physical system, encompassing biological, chemical, and physical interactions. Ideally, a fully realized digital twin possesses the ability to interact bidirectionally with its physical counterpart, enabling both monitoring and real-time control. This "ultimate dream" drives ongoing efforts to create sophisticated digital twins capable of replicating and influencing real-world bioprocesses.
In bioprocessing, mechanistic models — mathematical representations of natural phenomenon grounded in fundamental scientific principles such as physics, chemistry, and biology — play a supporting role to digital twins. These models elucidate the underlying mechanisms driving bioprocess behavior, providing a predictive framework based on established scientific laws.
Establishing a relationship between digital twins and mechanistic models can offer compounding benefits in terms of technical insights for bioprocessing applications. By serving as core components within a digital twin's architecture, mechanistic models can provide foundational understanding of a bioprocess's inherent dynamics. Digital twins, meanwhile, transcend simple mechanistic representations by enabling highly realistic simulations that leverage real-time data and analytical models alongside artificial intelligence (AI) and machine learning to reap transformative insights. This allows the digital twin to "personalize" its representation, adapting to specific use cases and mirroring the unique characteristics of a particular physical system.
Crucially, the power of a digital twin lies in its ability to remain current, reflecting the evolving state of its physical counterpart through real-time data integration. This dynamic adaptation enables the digital twin to not only simulate but also control the bioprocess, automating adjustments and minimizing human intervention. The balance between mechanistic and data-driven models is use-case dependent, requiring innovative approaches to optimize their integration. Ultimately, the goal is to create digital twins that can mimic the decision-making of experienced scientists, automating process control and optimization for greater efficiency and consistency.
The Many Uses For Digital Twins
From process optimization and development acceleration to seamless tech transfer and scale-up, digital twins are finding applications across the entire biopharma value chain. The industry is witnessing a growing recognition of their potential, with AI and digital twins becoming central to strategic initiatives across organizations.
The core driver behind this push is the fundamental need to expedite drug development and reduce costs, all while maintaining the highest standards of safety and efficacy: by enabling faster time-to-market, digital twins directly contribute to improved patient access to essential medications. In the development space, digital twins offer targeted solutions for optimizing upstream and downstream processes. These virtual replicas can significantly reduce the workload associated with process development, minimize raw material consumption, and accelerate overall timelines. Whether focusing on specific unit operations like bioreactors or addressing broader process challenges, the key is to align digital twin development with organizational strategic goals and patient needs.
For example, within bioreactor applications, digital twins can model cell culture behavior and mixing dynamics, facilitating seamless scale-up by replicating optimal conditions from smaller scales. The focus remains on addressing relevant problem statements that contribute to operational efficiency and, ultimately, better patient outcomes. Implementing automated control systems, even with simplistic devices, is a crucial first step toward realizing the full potential of digital twins. These control systems can be further enhanced by integrating mechanistic models, providing a robust framework for process optimization.
Implementing Digital Twins For Better Bioprocessing
The journey toward creating fully autonomous digital twins capable of controlling complex unit operations, such as bioreactors, requires a structured, phased approach. Starting with more accessible unit operations — and then gradually increasing complexity — is essential for building confidence and ensuring each component functions correctly.
A computational fluid dynamics (CFD) model serves as an excellent example of a proven technology that can be leveraged in this phased approach. CFD, used to simulate fluid and air behavior, is particularly valuable for understanding mixing dynamics within bioreactors. By accurately modeling these dynamics, developers can ensure consistent scale-up from smaller to larger bioreactors. Once this foundational understanding is established, further layers of complexity, such as cell culture kinetics, can be added to enhance the digital twin's capabilities.
This gradual development fosters confidence and facilitates broader adoption within an organization. Using digital twins to demonstrate tangible benefits for discrete unit operations, such as reduced at-scale testing and time savings, is crucial for gaining buy-in from upper management. The ability to anticipate and mitigate potential issues early in the process further solidifies the value of digital twins.
The role of AI in enhancing digital twin accuracy is highly context-dependent: when mechanistic understanding and models are sufficient, AI may contribute marginally. However, in scenarios where mechanistic knowledge is limited, AI and machine learning can fill critical gaps. The key lies in having access to relevant data for training and validating AI models that can adequately support a dearth of mechanistic knowledge.
While mechanistic models provide a strong foundation, they may not always capture the full complexity of specific bioprocesses. In such cases, integrating data-driven and statistical models becomes essential. Moreover, advancements in AI, such as physics-informed machine learning, are enabling the development of models that require less training data. AI can also augment simulation methodologies, improving the volume of data available for training and validating machine learning models. This data augmentation is particularly valuable when financial constraints limit the scope of design of experiments (DoE).
Improving Digital Twins To Improve Drug Development
The ability to personalize digital twins to specific operations hinges on data. Ready-to-use, contextualized data from diverse sources, such as manufacturing execution systems (MES) and process historians, is foundational for developing and deploying effective digital twins in manufacturing settings. This necessitates a robust IT infrastructure capable of collecting, standardizing, storing, and extracting data. Beyond simulation, digital twins can be used for feedback control, which requires seamless data connectivity, allowing the digital twin's output to influence process parameters in real-time. Implementing these control systems demands a holistic approach, one that incorporates data accessibility, model orchestration, and integration with control layers.
Organizations face various challenges in adopting digital twins, including data infrastructure limitations, convincing scientists and shop floor personnel of their value, and justifying investments to leadership teams. A phased approach, starting with impactful applications that demonstrate cost and time savings, is crucial for gaining buy-in. Quantifying the ROI of digital twins can be challenging, particularly when models provide new insights. However, focusing on KPIs such as cost reduction, FTE reduction, and time reduction can help demonstrate value.
The FDA has recognized the potential of digital twins and has released guidelines addressing key challenges, including data variability, model explainability, performance drift, and life cycle management. Early engagement with regulatory agencies is crucial for establishing a clear path to GMP implementation. Successful implementation of digital twins requires a multidisciplinary team, including experts in modeling, automation, IT, and data management. Upskilling operators and engineers is also essential for maximizing the benefits of this technology. Moreover, like any technology, digital twins have limitations, particularly around data and infrastructure constraints, making personnel support and continuous improvement strategies both crucial to their success.
Conclusion
The convergence of mechanistic modeling and digital twin technology heralds a new era for biologics development and manufacturing. By seamlessly integrating fundamental scientific principles with real-time data and advanced analytics, these tools empower organizations to optimize processes, accelerate timelines, and enhance product quality. While challenges remain in data management, regulatory navigation, and organizational adoption, the potential benefits are undeniable. As the biopharma industry continues to embrace digital transformation, the strategic deployment of digital twins, built upon robust mechanistic foundations and fueled by AI-driven insights, will be pivotal in driving innovation and ensuring the timely delivery of life-saving therapies to patients worldwide.