The 2 Emerging Fields Propelling The Use Of AI In Precision Medicine
By Apeksha Damania and Bill Woywod, Guidehouse
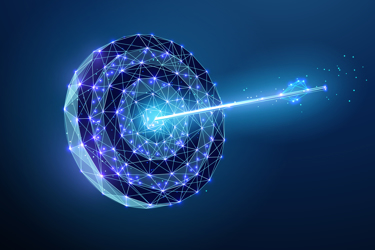
As Part 1 of a two-part series, this article examines how recent advances in oncology diagnosis and treatment preview the full potential of precision medicine. Part 2 will share insights on the challenges the industry must overcome before individualized healthcare can be fully realized.
Long searching for a way to customize drug therapies to meet the unique needs of patients, the pharmaceutical industry is getting closer to being able to take full advantage of precision medicine, thanks to recent breakthroughs in medical technology. The availability of multidimensional data coupled with fast-evolving computational hardware and programming techniques is enabling the use of artificial intelligence (AI) to usher in a new era in which treatments can be tailored to an individual’s genetic, environmental, and behavioral context.
While this innovative medical technology has yet to be fully realized, AI and machine learning (ML) are positioned to help clinicians understand and identify the conditions that may put patients at risk for complications.
Advances in AI-based analysis paradigms have created an unprecedented momentum in computational medical imaging applications, giving rise to new areas of precision medicine research. This article examines how recent medical imaging advances in oncology are bringing about new areas of precision medicine and previews its full potential.
Radiogenomics
In the relatively new field of image recognition, radiogenomics focuses on establishing associations between radiological features in cancer images and genomic or molecular expressions. These associations are used to inform early diagnosis and management of patients, as well as to help identify patients that are at risk of developing toxicity following radiotherapy. The radiogenomics methodology includes a predefined data acquisition protocol followed by a feature extraction (radiomics, deep learning, and traditional image processing techniques) and selection process.1 The association and modeling of these highly discriminative biomarkers can help identify changes at the tissue level, thereby increasing the efficacy of diagnosis and minimizing the risk of toxicity or recurrence of cancer.
Advances in radiogenomics and medical image biomarkers are proving to be increasingly effective in improving patient care. Recently, a team of researchers created a convergent AI/ML model to combine clinical data with image features to provide a more objective risk assessment of liver transplantation for hepatocellular carcinoma (HCC) patients.2 This model has the potential to identify risk factors for HCC recurrence beyond traditional factors like tumor size and biomarker analysis. The clinical implications of such a predictive model include the potential to better predict survival outcomes and effectively inform the transplantation allocation system.
Nanomedicine
Another emerging field, nanomedicine (i.e., the use of nanoscale healthcare technology in pharmaceutical applications to make medically useful products), also promises to be instrumental for achieving precision medicine. Properties of nanomaterials make them ideal for improving early diagnosis and treatment of neurodegenerative diseases and cancer.
Because nanomaterials have properties like high specific surface area, transformable shape and size, and tunable chemical reactivity that allows them to adapt to virtually any microenvironment, they are ideally suited for diagnosis imaging agents as well as drug-load carriers. From a precision medicine perspective, nanotechnology can be applied as a platform technology to address a wide range of unmet needs, such as for a subset population or as a tailored technology to address a patient-specific problem.
For example, early diagnosis of brain cancer can be made possible using a diagnostic method that combines magnetic nanoparticles with nuclear magnetic resonance (NMR) technology. The magnetic nanoparticles attach to macrovesicles secreted by brain cancer cells, and then NMR is used to detect these macrovesicle/nanoparticle clusters. In another scenario, a team of researchers is exploring carbon nanotubes and gold nanoparticles as sensors to detect proteins indicative of oral cancer.3
As the nanomedicine field continues to evolve, multifunctional approaches are being explored to simultaneously integrate therapeutic and diagnostic agents onto a single nanoparticle or deliver multiple nanomedicine functionalized therapies in unison. Like the conventional combination therapies, these strategies aim to improve the therapeutic outcome through targeted, multi-agent delivery that preserves drug synergy.
However, a persistent challenge in all forms of drug administration is that drug synergy is time-dependent, dose-dependent, and patient-specific at any given point of treatment.4 High intratumor and interpatient heterogeneities make it difficult to analyze the outputs from various diagnostic and therapeutic platforms. Establishing a system that screens for optimal nanoparticle preparation will enable more efficient drug delivery and its implementation as a personalized medicine tool.
Using pattern analysis and classification algorithms, AI/ML techniques can play a critical role in improving the diagnostic and therapeutic accuracy for nanomedicine. AI techniques can help optimize material properties according to predicted interactions with the target drug, biological fluids, immune system, vasculature, and the cellular membranes, all of which affect therapeutic efficacy.
In a recent study, Reker et al. demonstrated the application of ML integrated with high-throughput experimentation for the rapid and large-scale identification of effective nano particle formulations. (This development was previously limited by the inability to predict which mix of ingredients would have high loading capacities.5) In the study, ML was used to identify 100 self-assembling drug nanoparticles from 2.1 million pairings of 788 therapeutic small molecules and 2,686 approved excipients. In addition to providing a platform for the development of safer and more efficacious nanomedicine with high drug-loading capacities, AI/ML techniques offer an opportunity to personalize the nano delivery systems, considering features like drug absorption, genetics, allergies, etc., to help mitigate the side effects of the nanoparticle formulations.
Optimizing drug combinations can facilitate drug synergism to enhance clinical efficacy of cancer treatment. AI and its related technologies can be used to select the right nanomedicine combinations as well as to maintain optimum drug levels in the blood or at the target site. For example, the artificial intelligence platform CURATE.AI was used to prospectively guide the dosing of a bromodomain inhibitor (ZEN‐3694) and enzalutamide administered in combination to a patient with metastatic castration‐resistant prostate cancer to reduce serum prostate‐specific antigen (PSA) levels.6 The platform successfully identified dose adjustments for each drug, increasing the treatment efficacy and tolerance. This enabled the patient to continue with the combination regimen, resulting in a durable response and no disease progression.
Looking Ahead
The use of AI-driven medical imaging and AI-integrated nanotechnology offers new opportunities for the development of novel strategies in terms of prevention, diagnosis, and treatment of various diseases. However, before this AI-fueled precision medicine can be realized to the benefit of all involved, several critical challenges must first be overcome. Part 2 of this article series provides insights on the primary barriers to creating a new precision medicine standard of care.
About The Authors:
Apeksha Damania, Ph.D., is a consultant within the Life Science practice at Guidehouse. She has over seven years’ research experience in translational medicine, with technical expertise in regenerative medicine, biomaterials, tissue engineering, and drug delivery approaches. Her areas of functional expertise include market access, evidence-based research and analysis, competitive assessment, and publication and communication strategy.
Bill Woywod is an associate director in Health at Guidehouse. He has over 10 years’ experience leading advanced analytics and data science initiatives across the healthcare industry, including biopharma, med tech, and public and commercial payer. His areas of technical expertise include supervised and unsupervised machine learning, claims data analytics, rate setting, natural language processing, and econometrics. He founded and leads Guidehouse's COBRA expert community, a collective of experts at the intersection of life sciences and data science focused on thought leadership and continuous innovation.
- Trivizakis E, Papadakis GZ, Souglakos I, Papanikolaou N, Koumakis L, Spandidos DA, Tsatsakis A, Karantanas AH, Marias K “Artificial intelligence radiogenomics for advancing precision and effectiveness in oncologic care (Review)” Int J Oncol 57; 43-53 (2020) https://www.spandidos-publications.com/10.3892/ijo.2020.5063
- He T, Fong JN, Moore LW, Ezeana CF, Victor D, Divatia M, Vasquez M, Ghobrial RM, Wong STC “An imageomics and multi-network based deep learning model for risk assessment of liver transplantation for hepatocellular cancer” Comput Med Imaging Graph 11; 89: 101894 (2021) https://doi.org/10.1016/j.compmedimag.2021.101894
- Malhotra R, Patel V, Vaque JP, Gutkind JS, Rusling JF “Ultrasensitive Electrochemical Immunosensor for Oral Cancer Biomarker IL-6 Using Carbon Nanotube Forest Electrodes and Multilabel Amplification” Anal Chem 82(8); 3118–3123 (2010) https://pubs.acs.org/doi/10.1021/ac902802b
- Ho D, Wang P, Kee T “Artificial intelligence in nanomedicine” Nanoscale Horiz 4(2); 365-377 (2019) 10.1039/c8nh00233a
- Reker D, Rybakova Y, Kirtane AR, Cao R, Yang JW, Navamajiti N, Gardner A, Zhang RM, Esfandiary T, L’Heureux J, et al. “Computationally guided high-throughput design of self-assembling drug nanoparticles” Nat Nanotechnol (2021) https://doi.org/10.1038/s41565-021-00870-y
- Pantuck AJ, Lee DK, Kee T, Wang P, Lakhotia S, Silverman MH, Mathis C, Drakaki A, Belldegrun AS, Ho CM, Ho D “Modulating BET Bromodomain Inhibitor ZEN‐3694 and Enzalutamide Combination Dosing in a Metastatic Prostate Cancer Patient Using CURATE.AI, an Artificial Intelligence Platform” Adv Therap 1(6); 1800104 (2018) https://doi.org/10.1002/adtp.20180010