Incorporating AI Tools Into Downstream Process Optimization
By Foteini Michalopoulou, Imperial College London
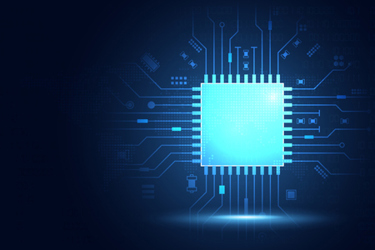
In process industries, from biomanufacturing and pharmaceuticals to petrochemicals and food engineering, mechanistic models have long been the go-to approach for simulating, optimizing, and controlling complex operations. These models, grounded in first principles, offer high-fidelity insights into system behavior. But as digitalization reshapes expectations for process monitoring and control, a key question emerges: how can we maintain rigorous scientific modeling while meeting the demands of real-time responsiveness?
Surrogate models, particularly data-driven and hybrid approaches, offer a compelling answer. These models are not designed to replace mechanistic thinking. Instead, they complement it by reducing computational load, enabling faster decision-making and extending the utility of rigorous models to scenarios where fast turnaround is critical.
The increasing adoption of digital twins, automated optimization routines, and closed-loop control strategies makes this blended modeling approach especially valuable. In the following case study, conducted in collaboration with Dr. Maria Papathanasiou, we explore this concept through the lens of ion exchange chromatography used in the purification of monoclonal antibodies.
When Time Is The Limiting Reagent
Real-time process optimization and control demand more than accuracy, they require speed. In settings where process parameters must be adjusted between process cycles or even within a single operating cycle, delays in computation can translate to missed opportunities, reduced yields, or compromised product quality.
This is especially true in continuous ion exchange chromatography, where the process runs in a series of repeating cycles. At the end of each cycle, there may be a chance to adjust operating conditions based on performance. In more advanced setups, updates may be needed even more frequently, sometimes every minute, requiring fast, reliable insights to guide those changes as the process runs.
In both cases, the goal is the same: improve yield and purity by adjusting key variables like flow rates or modifier concentrations. But the timeline available to do so varies significantly. What does not vary is the need for fast, accurate insights to guide those changes.
Here, traditional mechanistic models may fall short. While they offer deep understanding and predictive strength, they are computationally intensive. For cycle-to-cycle optimization, they are often too slow to explore the full space of potential adjustments within the limited time between runs. And for minute-by-minute decision-making, they are simply not fast enough to simulate a single scenario, let alone multiple alternatives.
This creates a practical roadblock. Even with a validated model in place, if it cannot deliver answers quickly enough, its value in real-time decision support becomes limited. That is where surrogate models enter the picture.
Building A Bridge With Surrogates
To overcome the timing constraints of mechanistic models, we turned to surrogate modeling. These models are trained to approximate system behavior based on existing process knowledge or data but run fast enough to be used in real-time decision support. In this case study, we focused on two types: a hybrid model1 that retains core aspects of the underlying process and a fully data-driven model2 that relies solely on observed input–output relationships.
Each was built using data generated by the mechanistic model of the system and designed to address a specific optimization need (Figure 1). The hybrid model was built for use between process cycles, where the time window, typically around 1 hour, is short but not instantaneous. The data-driven model, on the other hand, was developed for use within a running cycle, supporting updates at 1-minute intervals.
Figure 1: Schematic representation of the applicability of each model for online process optimization.
In both scenarios, the goal was to improve process yield while meeting a minimum threshold for product purity. This constraint was applied throughout the optimization and reflects the real-world demands of chromatographic purification, where quality specifications are nonnegotiable.
To understand whether the surrogate models were truly necessary, we also applied the same optimization routines to the full mechanistic model. This served as a benchmark for evaluating performance under real-time constraints. In the cycle-to-cycle case, the mechanistic model completed fewer iterations within the available window and delivered less favorable results, as shown in Table 1. In the minute-by-minute scenario, it was unable to return any results in time to support decision-making.
Regarding the surrogates, when applied to cycle-to-cycle optimization, the hybrid model stood out. By maintaining a connection to the process physics, it offered more reliable guidance within the available time. It was fast enough to explore hundreds of potential combinations and reliably identified parameter sets that improved yield without compromising purity.
In contrast, the data-driven model was the better fit for real-time use. Its lightweight structure allowed it to run nearly instantaneously, enabling thousands of iterations per hour, while still meeting the purity target. This made it particularly effective for minute-by-minute updates, where responsiveness is critical and even the hybrid model could not keep up.
Optimization Scenario | Model Type | Purity Constraint Met | Yield Achieved |
---|---|---|---|
Cycle-to-cycle | Mechanistic | Yes | 78% |
Hybrid | Yes | 82% | |
Data-Driven | Yes | 79% | |
Minute-by-minute | Mechanistic | No | - |
Hybrid | Yes | 63% | |
Data-Driven | Yes | 79% |
Table 1: Surrogate model outcomes across the two optimization scenarios.
Practical Integration And Flexibility
Both surrogate models were designed with deployment in mind. Because they rely only on variables already measured during standard operation, they are well-suited for integration into process analytical technology (PAT) frameworks, digital twins, that replicate and monitor the physical process in real time, or broader control strategy upgrades. Their speed and adaptability allow them to complement existing measurements, providing real-time predictions that support faster decision-making.
In chromatography, where measurement delays and limited real-time feedback are common, surrogate models can also help bridge information gaps. They can offer insight when measurements are unavailable or act as virtual sensors, offering predictions between sampling points, where direct measurement is delayed or unavailable. As more data becomes available, the models can be updated to improve accuracy over time, strengthening their role as trusted decision-support tools.
Importantly, in every case, the operating conditions identified by these models maintained the required purity constraints while improving yield. This consistency is especially valuable in purification processes and supports their use in quality by design (QbD) strategies, where performance must align with both efficiency and quality expectations.
By accelerating optimization cycles and providing fast, reliable guidance, these models can help reduce development timelines, improve batch consistency, and support a more agile manufacturing environment.
A Collaborative Future: Using The Right Tool At The Right Time
Each modeling strategy has its place. Mechanistic models remain essential for detailed process understanding, regulatory filings, and rigorous scenario planning. Hybrid models offer speed and interpretability, making them ideal for online optimization where decisions must be both quick and still anchored in process understanding. Black-box models, while less transparent, deliver the agility needed for real-time control systems and digital twin integration.
Rather than selecting one model to serve all purposes, a layered approach allows teams to use the right tool at the right time. By combining models within a flexible framework, manufacturers can adapt to different phases of development and operation without losing scientific grounding.
This work demonstrates the role surrogate models can play in making mechanistic insights actionable under time constraints. Far from replacing first-principles thinking, hybrid and data-driven models extend its impact. When used together, they allow for faster, smarter, and more adaptable decision-making. In doing so, they help with aligning process understanding with the pace of modern manufacturing demands.
References
- Michalopoulou, F. & Papathanasiou, M.M. (2025) An approach to hybrid modelling in chromatographic separation processes. Digital Chemical Engineering. 14, 100215.
- Michalopoulou, F. & Papathanasiou, M.M. (2024) Assessment of data-driven modeling approaches for chromatographic separation processes. AIChE Journal. e18600.
About The Author:
Foteini Michalopoulou is a Ph.D. candidate at Imperial College London specializing in machine learning applications for process design, optimization, and control. Her research focuses on predictive modeling and time series forecasting. Connect with her on LinkedIn.