Implementing Small-Scale Models For Biopharmaceutical Development
By Joschka Johannes Buyel (Bayer AG), Klaus Kaiser (Bayer AG), Kiran Andra (Alexion), Robert G. Luo (GlaxoSmithKline R&D), Alexis Henry (Biogen), Caroline Leveder (Sanofi R&D), Mark Richards (Eisai Inc.), Trish Connolly (BioPhorum Development Group)
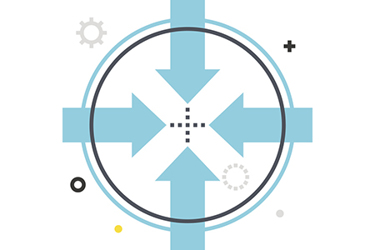
Small-scale models (SSMs) are widely used in the biopharmaceutical industry. These models are used for process development and optimization, scale-up, technology transfer, process characterization, process validation, virus clearance studies, and resolution of deviations encountered during manufacturing throughout a product’s life cycle. SSMs are also referred to as “scale-down models” or “scaled-down models.”
Demonstration that an SSM is representative of the large-scale manufacturing system is called “small-scale model qualification” (SSMQ), which is sometimes also referred to as “assessment,” “evaluation,” or “verification.” The demonstration is an important task that supports process validation and is required by regulatory authorities.1,2,3 However, design, execution, and analysis of SSMQ studies can be challenging due to the lack of clear guidance on current best practices.
In this three-part article series, we will provide options and tools for design, execution, and data analysis of SSMQs together with illustrative case studies. The articles are intended to provide an overview of current practices for the qualification and refinement of SSMs used in the development of biopharmaceutical drug substance manufacturing processes. SSMs are utilized to meet regulatory expectations.4,5,6, It must be experimentally demonstrated that an SSM – running at the appropriate process parameters (inputs) – has comparable performance and quality attributes (outputs) to the manufacturing scale.
The articles summarize the discussions and exchanges of a BioPhorum Development Group workstream on small-scale model justification. Their purpose is to provide reference documents for bioprocess professionals who will carry out small-scale model justification. In order to write the articles, surveys were conducted among BioPhorum workstream team members on the practices of conducting small-scale model justification. The results of the surveys are not presented in these articles but are available in an expanded BioPhorum white paper on the topic.7 The first part discusses the applications of the models, including viral clearance, quality oversight, and design considerations for justification of SSMs.
Applications Of The Model
SSMs are utilized throughout the life cycle of a product, both before and after the model is qualified. Early-phase process development relies heavily on the use of SSMs in studies that enable clinical manufacturing, which are performed well in advance of model qualification. Late-stage process development studies such as characterization utilize SSMs to support the identification of critical process parameters (CPPs) and to define proven acceptable ranges (PARs) for the process parameters of each unit operation in both the upstream and downstream process. During the validation of a process, SSMs are utilized in upstream for the evaluation of media stability, cell line stability, and qualification of raw materials as well as in downstream studies that support impurity clearance validation. SSMs are also used in life cycle management of a product post-approval as they are utilized to support process, raw material, cell bank changes as well as in the resolution of deviations.
The production process of a biological therapeutic derived from human or animal cell culture must include orthogonal methods of removing or inactivating potential viral contaminants. ICH Q5A8 provides industry guidelines for generating the data that appropriately supports the capacity of a production process to clear infectious viruses. Considering viral clearance can only be demonstrated using virus, verification at the manufacturing scale is not possible. Instead, early-phase demonstration and late-stage characterization and validation of viral clearance are performed at the laboratory scale using SSMs. As such, it is critical to justify the validity of the SSMs used in these studies and demonstrate comparability of the model to the manufacturing-scale process. Critical parameters for unit operations in the manufacturing process must be considered in the design of the SSM and either conserved to mimic the manufacturing scale or in some cases, worst-case conditions can be utilized, if such conditions are known. Justification of the validity of the SSM is demonstrated by comparing the process responses such as yield, purity, and chromatograms from the SSM to those responses achieved by the unit operation at the manufacturing scale.
Quality Oversight And Design Considerations
This section describes the role of quality assurance in the SSM implementation and qualification. As the model matures from an early-stage development tool into use for late-stage process validation and continuous process verification, the level of quality oversight increases. While practice varies from company to company, quality assurance tends to be involved in SSM report review and situations where the model is being used to evaluate high-risk steps or changes such as late-stage viral clearance studies or post-approval raw material changes.
A manufacturing process is developed at small scale, then scaled up to large scale. The technical system representing the final commercial manufacturing scale for further studies (e.g., process characterization studies) is referred to as an SSM in the scope of this document.
At some point in the life cycle of a product, the representativeness of the SSM for the manufacturing scale process must be verified. This exercise is referred to as “justification” in the scope of this document, which includes “assessment” and “qualification.” If this exercise is based on data, SSM data is compared to manufacturing-scale data and associated acceptance criteria are usually set using large-scale data.
Small-Scale System Vs. Large-Scale System
The goal of SSM design is to be representative of the large-scale system. In order to achieve this, a number of input parameters have to be maintained constant or scaled appropriately. Aside from the purely scale-related differences (e.g., reactor or column volume, etc.), the systems utilized at small and large scale may differ as well (e.g., manufacturer brand, mode of operation, vessel geometry, etc.). However, differences should be assessed for their risk to the representativeness of the SSM. The representativeness of the SSM is confirmed by direct comparison of the outputs – typically key performance indicators (e.g., titer, VCD, etc.) and product quality attributes (PQAs) obtained in both systems along with evaluations by subject matter experts (SMEs) based on scientific rationale and historical knowledge.
Risk-Based Design For Small-Scale Model Justification
When designing a SSMQ study, the approach taken should be risk-based. There are two types of risks: (1) technical risk and (2) business risk. Prospectively designing the qualification study adds rigor and helps ensure the study design is appropriate for addressing the level of risk. The rigor to prove representative behavior of the model depends on the following aspects: experience with the model, complexity of the unit operation, and progression of the project (i.e., model expectations and intended use).
Assessment And Qualification Of Small-Scale Models
Both “assessment” and “qualification” of an SSM refer to efforts to understand if the SSM can be used to represent the manufacturing process. When an SSM is used, this justification should be carried out. Qualification is more formal and rigorous than assessment. For example, one large-scale run can be considered sufficient for an assessment, while usually more than one large-scale run is needed to conduct a qualification, based on a survey of team members; results are presented in the white paper.7
Justifying the SSM is an effort to demonstrate that the small-scale system performs equivalently to the large-scale system in terms of outputs (e.g., CQAs, step yield, etc.). Samples are taken from both the small-scale population and the large-scale population to demonstrate that the outputs from the small-scale population are equivalent to the outputs from the large-scale population. Based on the purpose of SSMs, some models need to be assessed, while some need to be qualified based on their intended use, as determined using a risk-based approach.
Number Of Runs Needed
When conducting SSMQ, bioprocessing professionals often encounter these questions: “How many large-scale runs do we need to qualify the model?” “How many small-scale runs do we need to carry out in the lab?” A survey was conducted among the team members. Most team members consider a minimum of three large-scale runs are needed in order to conduct statistical analysis of the outputs. At a minimum, the same number of runs as large scale should be performed for small scale. One can conduct more runs at small scale since the studies are much easier to arrange at small scale than at the large scale.
Acceptance Criteria And Evaluation Criteria
In an SSMQ, acceptance criteria are the criteria that the model needs to meet to be qualified. On the other hand, evaluation criteria are criteria that if the model fails to meet them, it does not mean the model automatically failed to qualify; instead, further evaluation is needed. The use of acceptance vs. evaluation criteria may vary across companies. The acceptance criteria or evaluation criteria for the SSMQ are typically based on large-scale data or scientific rationale. This topic will be discussed in more detail in section 7.0 of the forthcoming white paper.1
Use Of Statistical Methods
When performing an SSMQ, most companies employ a statistical evaluation of the behavior of the small scale when compared to the manufacturing scale, according to the survey of team members.
Recent advancements in process intensification, alongside new drug modalities with low dosage requirements (such as antibody-drug conjugates), limit the number of large-scale runs required to supply clinical trials. Usually, these large-scale runs represent the basis for statistical evaluation of the small-scale model. Lacking this data, some companies have sought to establish methods to handle these kinds of situations. One option is to collect data until sufficient batches are available for a statistical analysis. In this case, process characterization is performed at risk. Another option is using scientific evaluation without applying a formal statistical method. This approach requires experience with the small-scale model being used, combined with sufficient platform knowledge.
When a good understanding of the small-scale model limitations is established, data generated at small scale may be leveraged to provide statistical limits (e.g., large-scale variability).
In Part 2, we will discuss the current opinion on how to execute small-scale model qualification for both upstream and downstream SSMs and analytical setup.
Disclaimer: The approaches and methods presented in this paper resulted from surveys and discussions among team members from member companies. These approaches and methods do not in any way restrict member companies from using other approaches and methods they consider appropriate for their company’s studies. The authors have no other relevant affiliations or financial involvement with any organization or entity with a financial interest in or financial conflict with the subject matter or materials discussed in the manuscript apart from those disclosed.
References
- ICH Harmonised Tripartite Guideline Q11: Development and Manufacture of Drug Substances (Chemical Entities and Biotechnological/Biological Entities. International Conference on Harmonisation of Technical Requirements for Registration of Pharmaceuticals for Human Use. 01 May 2012.
- EMA/CHMP/BWP/187338/2014: Guideline on Process Validation for the Manufacture of Biotechnology-Derived Active Substances and Data to be Provided in the Regulatory Submission. European Medicines Agency, Committee for Human Medicinal Products. 28 Apr 2016.
- Food and Drug Administration (FDA). Process Validation: General Principles and Practices. Guidance for Industry. Jan 2011.
- ICH Harmonised Tripartite Guideline Q7: Good Manufacturing Practice Guide for Active Pharmaceutical Ingredients. International Conference on Harmonisation of Technical Requirements for Registration of Pharmaceuticals for Human Use. 10 Nov 2000.
- ICH Harmonised Tripartite Guideline Q9: Quality Risk Management. International Conference on Harmonisation of Technical Requirements for Registration of Pharmaceuticals for Human Use. 09 Nov 2005.
- ICH Harmonised Tripartite Guideline Q10: Pharmaceutical Quality System. International Conference on Harmonisation of Technical Requirements for Registration of Pharmaceuticals for Human Use. 04 Jun 2008.
- Justification of Small-scale Models: An Industry Perspective. BioPhorum Development Group, Qualification of Small-Scale Models Workstream. May 2021.
- ICH Harmonised Tripartite Guideline Q5A(R1): Viral Safety Evaluation of Biotechnology Products Derived from Cell Lines of Human or Animal Origin. International Conference on Harmonisation of Technical Requirements for Registration of Pharmaceuticals for Human Use. 23 Sep 1999.