How To Navigate The Patenting Challenges Of AI-Assisted Drug Discovery
By David McCombs, Vincent Shier, Eugene Goryunov, Dina Blikshteyn, and Brooke Cohen; Haynes Boone
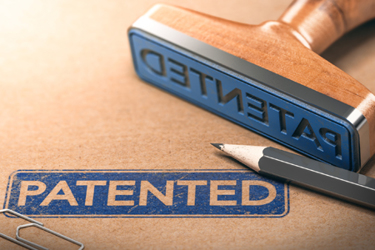
The pharmaceutical industry faces multiple challenges when introducing new drugs to the market. Not only does the process sometimes take over a decade, over 90% of drugs fail before FDA approval.1 In addition, the average cost to bring a single drug to market is a staggering $3 billion.2 With “low hanging fruit” drugs already in the market, companies are now looking at complicated or rare diseases and finding it more difficult to discover the next blockbuster treatment.3
Faced with these challenges, artificial intelligence (AI) has become a new arrow in the drug discovery quiver and has effectively transformed the pharmaceutical industry. AI, specifically machine learning and neural networks, has significantly decreased the cost and time for drug development.
What Are The Current Uses Of AI?
Pharmaceutical companies have incorporated AI into various stages of drug development with resounding results. For example, at the initial stages, a company is faced with what appears to be an insurmountable challenge of identifying a target as well as designing possible molecules to test with the hope of bring about a therapeutic benefit without being cytotoxic. With the power of AI, companies can reduce this overwhelming challenge of searching among millions of candidate compounds for even a single target to a more manageable and testable set of candidate compounds numbering in the hundreds. Even if this set does not turn up a “winner,” AI can help evaluate the results for these molecules to fine-tune the next generation of candidate compounds.
In so doing, building blocks for different types of drugs and gene therapies can be identified.4 Ultimately, the goal of this AI-driven drug discovery process is to efficiently narrow the field of potential drug targets to find the needle in the proverbial haystack that can be taken to clinical trials and in so doing reduce the overall cost and time spent. AI is also being turned to for assistance in establishing suitable protocols for clinical trials, to identify patients to enroll in clinical trials, and to improve patient monitoring during the trials.5
These shiny new tools have allowed scientists to analyze millions of relevant data points using advanced and complex mathematical and statistical concepts to predict potential drug candidates. Such data points may include information about a relevant disease, target protein, toxicity, or data on known protein-molecule interactions or drug molecules. By analyzing a huge volume of information quickly, AI can accurately identify potential drug targets without scientists having to perform physical testing at each step. In other words, AI can perform what is a manual and arduous task much faster.
AI has indeed delivered. To date, there are several AI-discovered drugs by Exscientia, Recursion, and Berg Health that are undergoing clinical trials for FDA approval.6
Companies are also employing AI for antibody discovery for use in therapies. Because the structure of an antibody is complex, creating antibodies that are highly effective against a particular antigen can be difficult. For this reason, many companies are now developing AI programs that can analyze data points, antibody sequences, and structures to design a full or partial sequence for a potential antibody target. These programs can also analyze data from initial trials to optimize the antibody structure to improve affinity. Although there are no AI-developed antibodies in clinical trials yet, there is a lot of excitement and investment into companies and research in this area. In one example, Nabla Bio, a Harvard University spinoff, raised $11 million in seed funding to develop its AI-based platform for therapeutic antibody discovery.7
What Are The Patenting Challenges When Using AI To Develop Drugs?
While AI is having a tremendous impact on drug development, a lack of clarity in patent law for AI-driven innovations means that companies may find it difficult to patent their inventions. For example, patent applications that use AI may face patent eligibility challenges as being directed to unpatentable subject matter including abstract ideas or laws of nature. It is also unclear how much disclosure in a patent application is necessary to satisfy the written description and enablement requirements, especially when drafting claims directed to antibodies (a complicated and unclear area on its own). These hurdles will each be discussed in subsequent articles.
AI-based inventions also raise considerable issues for inventorship. For a patent to be valid, every inventor listed on the application must have participated in conception of the invention and every inventor must be listed on the patent application.8 “Conception is the touchstone of inventorship”9 and requires the inventor to have formed a sufficiently definite idea of the “complete and operative invention.”10 Reducing the invention to a physical manifestation of the idea (i.e., reduction to practice) is not necessary; however, the person must at least perform the mental task of creating the entirety of invention.
In some countries, like South Africa and Australia, AI can be an inventor. However, most countries have laws that stipulate that inventors must be human. In those countries, AI cannot be an inventor.11 In U.S., the Federal Circuit recently heard arguments regarding whether the term inventor in the Patent Act is broad enough to include AI. A recent ruling in Germany held that AI cannot be an inventor, but states that the owners of AI can name themselves as the inventors on the patent application.12 The court declined to say whether the owners would properly be considered the inventors or whether this was just a way to prevent the application from immediate rejection.13 However, this ruling presents an interesting thought for how the law may evolve in the future.
At present, few AI programs are sophisticated enough to “invent.” For the most part, humans still may be considered to conceive the invention because they start with the identification of the drug target, develop the AI input parameters, control/monitor the AI output around select inclusion criteria, and assess the ultimate validity of the candidate pool based on external parameters that the scientist establishes. In this process, AI is merely a tool that the scientist uses for drug discovery in a similar manner that a “dumb” computer may have been used to help map out a combinatorial library based on predictive binding parameters in silico. As such, a strong argument can be made that drugs developed with the assistance of AI are patentable despite the uncertainty in the inventorship law.
In countries where AI cannot be an inventor, several important questions exist, including: When does conception occur when AI is involved? How much do humans need to be involved in conception? If AI is designed to mimic human intelligence, what happens if AI conceives of a drug or an antibody? If there is no human conception, is the innovation unpatentable?
AI will likely soon approach and even surpass human intelligence for certain purposes. When that happens, it may be possible that the AI program will conceive of the drugs and scientists may only be reducing the drugs to practice. In this case, the drug candidate that the AI program invented would likely be unpatentable under current law. So, if companies invest in AI, how will they recoup their investment?
How Can AI-Developed Drugs Be Protected?
There are a few ways that an AI-based patent application can preempt inventorship issues. First, the application can explain how humans developed the AI and include code or pseudo-code that a human wrote in creating the AI. For example, humans decide what mathematical or statistical methods AI should use to analyze the data and determine how AI “thinks” through the problems presented to it. Since AI is trained on data, humans can also conceive the data sets used to train the AI. Depending on the type of training data used, AI may be designed to perform a certain task or focus on a particular aspect of the input. These tasks can include identifying small molecules, antibodies, or genes that have particular characteristics.
Second, the patent application can explain a human contribution for analyzing an AI-produced output and why this step is part of the conception. For example, when AI outputs candidate molecules, antibodies, or genes, these structures are nothing more than an in silico designed library of candidate compounds, albeit of a much smaller size. Much inventive work is still required to develop synthetic means of producing the candidates, envisioning their utility, and devising methods of testing.
Another way to minimize inventorship issues is to minimize the role of AI. For instance, a patent application can explain that AI is but one of many methods for performing complex data analysis that identifies potential drug targets. In other words, although AI may have been used by the inventors it is distinctly possible that other less efficient means may have also been used. Describing these alternative means may mitigate the risk of AI being deemed an “inventor.”
Even if a drug is ultimately held to be “invented” by AI, prudent drafting practice would encourage practitioners to draft applications that include embodiments that are clearly conceived by humans. These embodiments include methods of making the drug, methods of using the drug, pharmaceutical formulations, possible modifications or precursors, etc. Another approach is to describe the elements of a class of molecules that work for a certain therapeutic purpose.
Because AI may output a small group of molecules, a practitioner may want to avoid writing a claim that too narrowly captures these molecules that are the direct result of the AI output. Instead, practitioners may want to consider broadening the claims to include a class that humans have contributed to defining. For example, if the AI outputs molecule A as a potential drug target, scientists may use this molecule to discover that molecule A belongs to Class I, which includes molecules B, C, D, and E. Thus, practitioners could direct claims to the entire Class I rather than just molecule A.
Should Companies Continue To Invest Resources In And Patent AI?
The patent act’s primary focus is to promote progress in the useful arts, which unquestionably includes providing incentives and rewards for discovery of new therapies and new therapeutics. As anyone in the patent community is familiar with, laws change, whether by act of Congress or through judicial interpretation. Thus, any present questions about the use of AI in drug discovery should not stop or prevent the pharmaceutical industry from investing in AI nor should it stop companies from pursuing patent protection for their discoveries. AI’s potential to significantly reduce the cost and time of drug development is too valuable for companies to slow their progress. Further, despite potential issues with inventorship, strategies exist that may mitigate the risk that AI-developed drug patents may be challenged on inventorship grounds.
This article reflects only the present personal considerations, opinions, and/or views of the authors, which should not be attributed to any of the authors' current or prior law firm(s) or former or present clients.
- Suzi Ring, How AI Is Aiming at the Bad Math of Drug Development, Bloomberg (Nov. 29, 2021, 4:47 AM), https://www.bloomberg.com/news/articles/2021-11-29/how-ai-is-aiming-at-the-bad-math-of-drug-development-quicktake
- Id.
- David H. Freeman, Hunting for New Drugs with AI, Nature (Dec. 18, 2019), https://www.nature.com/articles/d41586-019-03846-0
- Ring, supra note 1.
- Debleena Paul et al., Artificial intelligence in drug discovery and development, 26(1) Drug Discovery Today 80, 87 (January 2021).
- Exscientia, https://www.exscientia.ai/pipeline (last visited Feb. 28, 2022); Recursion Pharmaceuticals, https://www.recursion.com/pipeline (last visited Feb. 28, 2022); Berg Health, https://www.berghealth.com/research/healthcare-professionals/pipeline/bpm31510/ (last visited Feb. 28, 2022); Berg Health, https://www.berghealth.com/research/healthcare-professionals/pipeline/bpm31543/ (last visited Feb. 28, 2022).
- Andrea Park, Harvard spinout Nabla Bio nabs $11M for AI-driven antibody design, Fierce Biotech (Dec 7, 2021 08:34 a.m.), https://www.fiercebiotech.com/medtech/ai-driven-antibody-designer-nabla-bio-raises-11m-seed-funding
- 35 U.S.C. § 115-116.
- Burroughs Wellcome Co. v. Barr Laboratories, Inc., 40 F.3d 1223, 1227-28 (Fed. Cir. 1994).
- Hybritech, Inc. v. Monoclonal Antibodies, Inc., 802 F.2d 1367, 1376 (Fed. Cir. 1986).
- Thaler v. Hirshfeld, 1:20-cv-903, U.S. Dist. LEXIS 167393, at *25 (E.D. Vir. Sept. 2, 2021); Matthew Bultman, Can a Robot Invent? The Fight Around AI and Patents Explained, Bloomberg Law (Sept. 9, 2021, 4:01 a.m.), https://news.bloomberglaw.com/ip-law/can-a-robot-invent-the-fight-around-ai-and-patents-explained
- Kingsley Egbuonu, The latest news on the DABUS patent case, IP Stars from Managing IP (April 4, 2022), https://www.ipstars.com/NewsAndAnalysis/the-latest-news-on-the-dabus-patent-case/Index/7366
- Id.