How To Add Efficiency From Development To Validation With Design Of Experiments, Part 1
By Peter Alexander and Thomas Harrington
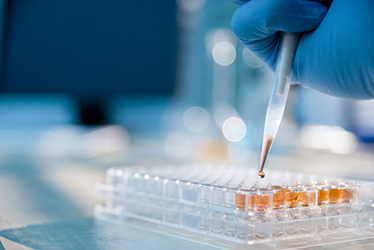
This is the first in a two-part series on using design of experiments in biologics development and manufacturing.
Among many challenges in the biopharmaceutical product development cycle is the development and validation of high-yielding, reproducible processes for manufacturing products that are suitable for use in humans. Unless a platform process is available for a “plug-and-play” approach to process development, a broad variety of potential process input parameters must be evaluated against a similarly large set of output parameters. In some cases, even platform processes need to be modified to account for the biochemical differences between similar biological products.
Having tools to efficiently screen process parameters for their criticality in product yield and purity, and to identify the proven acceptable ranges for those parameters, shortens process development timelines and reduces process development and validation costs. One such tool involves the application of a statistically-based design-of-experiments (DoE) approach to process development and validation.
DoE Basics
The efficiency of using DoE comes from its ability to reduce the total number of experiments required to evaluate a given parameter set while adding statistical robustness to the analysis of the output data. Consider the following example for developing a cell culture process.
Process parameters in cell culture include but are not limited to nutrient concentration, temperature, pH, and oxygen transfer rate. Each of these “lumped parameters” involves multiple component parameters, but assume for a moment that these four parameters are the only ones to consider.
To evaluate four parameters at only two levels (i.e., high and low values) would require 24 (2n) or 16 experiments to test each parameter in a pairwise study. Now suppose that four different nutrients need to be tested at two levels, and oxygen transfer rate must be assessed by the combination of three parameters (air flow rate, agitator speed, and pure oxygen flow rate). The number of pairwise experiments is now 28 or 256. That process performance should be assessed by multiple process outputs (e.g., product concentration, product purity) makes the analysis even more complex and expensive.
By applying a DoE parameter screening design, eight process parameters can be evaluated at two levels in as few as seven (n-1) experiments using a Plackett-Burman design. Basic Plackett-Burman studies that accommodate up to 21 variables can only identify the main effects of the parameters under study and interactions between the parameters cannot be resolved. However, all main effects can be determined, and some parameters can generally be eliminated from further experiments. Table 1 illustrates a typical Plackett-Burman study.
Fractional factorial designs are often used as a starting point for screening up to 21 variables to identify those that are critical to the process. By randomly assigning the combinations of high and low values for each parameter, a statistically robust experimental study (p<0.05) can be achieved for determining the main effects of the input parameters in the design. Center points can be added to fractional factorial designs.
Two-factor interactions can be identified by modifying the design to include additional experiments in a fractional factorial design. These designs may be quarter-, half-, or full-factorials by increasing the number of experiments to perform and thereby increasing the statistical resolution of the study.
Once a given design is established with the required number of input parameters and the cognate number of experiments to perform, any number of output parameters (e.g., cell density, cell viability, product titer) can be added to the design and analyzed concurrently. After executing the experiments described by the input parameters, output data are entered into the software to complete the statistical analysis (Table 1, Cell Yield column).
Table 1. Basic Plackett-Burman Design Example. Seven parameters are evaluated at two levels in eight experiments, where “-“ and “+” represent the low and high parameter values to be tested. This is a Resolution 3 design, which can only resolve the variables, if any, that influence the output parameters (e.g., cell yield).
Having identified the parameters that have the main effects on the process (possible critical process parameters), other DoE designs can be applied to optimize those parameters. Commonly, full-factorials or response surface designs (RSDs) like the central composite design are used to study three or four parameters at multiple levels to find their optima. A central composite design for three parameters at five levels is composed of 20 experiments including six center points and will determine the optimal level for each of those inputs for multiple output parameters (Table 2). One advantage central composite design has over other designs is that data from a properly designed fractional-factorial design can be used, thus reducing the total number of experiments required to complete the central composite design matrix.
Table 2. Central Composite Design Example. In this example, three input parameters are evaluated at five levels with Level 0 being the normal set point (center points), “-“ and “+” being the low and high parameter values based on previous screening studies, and “- alpha” and “+alpha” being the “low-low” and “high-high” parameter values set by the DoE software. Product yield is another example output parameter. This type of design may be suitable for complex operations such as fermentations, refolding operations, and chromatography.
Strategy For Product Life Cycle Toward Process Validation
As described above, a sequence of fewer than a dozen experiments in a screening design, followed by two dozen experiments in a response surface design can be used to optimize a typical biopharmaceutical production process and provide a statistically significant justification for those setpoints. To obtain these same rigorous results from a series of “one-off” experiments would require hundreds of experiments to be executed and analyzed manually.
Two examples of design strategies are presented here as commercial case study examples and will be described in greater detail in part 2 of this series. For ease of illustration, the first design strategy uses a four-factor full-factorial design as a central composite design. The example shows a progression from a half-factorial design (Table 3), which is a Resolution 3 screening design, to a full-factorial design (Resolution 4 central composite design) simply by “folding it over” (Table 4). This allows for all main parameters to be verified as significant before committing to the full design with sufficient resolution to identify interactions between input parameters. For this example, four parameters are investigated in 16 experiments using four center points to determine main effects and interactions in the fermentation unit operation.
The second design shows how a screening design can be implemented directly as a Resolution 4 design by beginning with a “folded-over Plackett-Burman Design.” For this example, 12 parameters are investigated in 24 experiments to determine main effects and interactions in the upstream process.
Table 3. Half-Factorial Design with Two Center Points. In this example, four input parameters are evaluated at five levels with Level 0 being the normal set point (center points) and “-“ and “+” being the low and high parameter values based on previous screening studies.
Table 4. Full-Factorial Design with Four Center Points. Experiments 1-10 (red) and 11-20 (blue) are each half-factorial designs with two center points [Resolution 3]. Table 3 represents the first 10 experiments (red), which comprise a half-factorial. The augmented data set of 20 experiments becomes a Resolution 4 design allowing all main effects and interactions to be identified.
DoE In Process Validation
As a new biopharmaceutical product moves through its development cycle toward Phase 3 clinical trials and eventual market launch, regulatory authorities require that manufacturers establish the reproducibility and robustness of their production process through process characterization and process validation. These studies involve large numbers of experiments to determine the normal operating range (NOR), proven acceptable range (PAR), and in some cases, the limit of failure (LOF) for each of the critical process parameters (CPPs) that the process development program has established. Frequently, how CPPs in individual unit operations interact to affect the critical quality attributes (CQAs) of the final product become important parameters for the characterization program.
The DoE tools described above for process design can greatly aid the characterization and validation of late-stage production processes. Part 2 of this series will describe two case studies in which DoE strategies were used to characterize a biopharmaceutical process leading into process validation for a Phase III program.
About the Authors:
Peter Alexander has over 35 years of industrial biotechnology experience in process development for therapeutic and vaccine products in numerous technology platforms, including conjugate polysaccharides, recombinant proteins, DNA vaccines, adenovectors, and virus-like particles. He is an independent consultant with a focus on CMC, technology transfer, and scale-up. He has held management and senior director positions for Aeras, IDT Biologika Corp., Lonza, Cambrex, and Baxter among other companies.
Thomas Harrington is an independent consultant with over 20 years of experience in biopharmaceutical and pharmaceutical process development and manufacturing. Throughout his career, he has led process and operational strategy initiatives for Abbott Laboratories, Altus Pharmaceuticals, Lonza Biologics, and Genzyme Corp., which is now Sanofi’s specialty therapeutics division. He has expertise in process validation of biopharmaceutical manufacturing processes. He has extensive practice in using design of experiments to perform process characterization studies to determine critical process parameters for upstream and downstream unit operations.