How Mechanistic Modeling Eases Wet Lab Dependence
By Emmanuel Mensah, Keck Graduate Institute
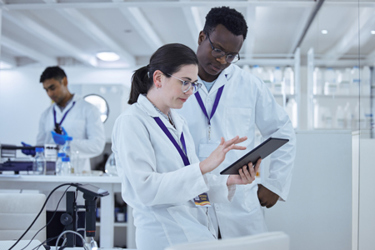
The process of purification is essential in biopharmaceutical manufacturing in order to guarantee product quality. Conventional approaches mostly rely on resource-intensive wet lab experiments. Mechanistic modeling provides a more efficient approach. This software generates a digital twin of chromatography steps, predicting critical outputs like yield and purity.1-4 At present, most mAbs are only purified using two chromatography steps, a Protein A capture step and a polishing step, like anion exchange chromatography, to remove process and product-related impurities.5 These resources are designed to assist in late-stage discussions. This study describes a process for assessing model credibility using a risk-based method for late-stage process development applications.6
The Project Plan
At least two key objectives were taken into consideration when designing the project:
- A risk assessment on using the model to answer questions of interest.
- Applying the model to complete process characterization studies and inform a control strategy.
Mechanistic modeling was leveraged in this project using Cytiva’s GoSilico software. It primarily serves to mimic chromatography steps in a computational environment, thereby substantially reducing the dependence on physical experiments.
What Is GoSilico?
GoSilico is a mechanistic chromatography software, which uses a computer to simulate the steps in separating biomolecules using chromatography. It generates a digital twin of specific chromatography steps capable of predicting critical process outputs such as cycle times, purity, pool volumes, and yield. It can be applied across various types of molecules and also can be used in multiple stages of drug process development.
- Early stage – process development and optimization
- Late stage – process characterization and control strategy definition
Assessing Model Credibility Using A Risk-based Method
Evaluation of the model's credibility in relation to wet lab downstream studies was conducted using a risk-based scoring system to ascertain the regulatory comprehension of the process itself.7 In connection with the process, specific technical issues of relevance were established, and a score was provided for two sections described as “model influence” and “decision consequence.” Model influence describes how much information is based on the model compared to wet-lab data while decision consequence describes the severity of the negative outcome of the decision if an incorrect prediction is made.6 The numerical scores assigned to these sections were considered when determining the risk assessment final score.
Example: Assessing Effects On Purity
Let’s consider a case in which the model was applied to predict how a load challenge would affect the product's purity in the elution pool, which is a crucial quality attribute (CQA). The predictions of the model aid in determining whether the process will satisfy the necessary purity standards without requiring significant physical testing.
Model influence: This section evaluates how much the decision relies on the model's data compared to wet lab data. If the model is used extensively for predictions with minimal wet lab verification, the model influence score would be high.
Decision consequence: This section assesses the potential negative impact of an incorrect model prediction. For a critical quality attribute like purity, an incorrect prediction could lead to significant regulatory and product quality issues, such as product recalls or failed batches. Thus, the decision consequence would be high.
A high score highlights the significant risk involved in using the model to make decisions and underscores the necessity of thorough validation and verification processes to ensure the model's predictions are trustworthy and accurate.
Assessing Model Credibility
A comprehensive information-gathering process and examination of key factors was conducted to assess the model's credibility — in other words, to confirm its reliability and trustworthiness. Credibility should align with the level of risk associated with the model. As such, model risk drives the selection of credibility goals and activities.7 Credibility goals were determined based on desired qualitative or quantitative outcomes, such as pre-specified acceptance criteria, which were established using scientific reasoning. These activities include model fitting, risk assessment, software code verification, and validation through adequacy assessments relevant to the context of use (COU). Verification and validation (V&V) activities were categorized into credibility factors, with the required rigor depending on the COU and model risk. V&V activities and goals were outlined, including acceptable outcomes in a detailed plan, and executed systematically, ensuring the model's credibility was thoroughly assessed and maintained.6
Questions Of Interest And Model Risk
Identifying the question of interest is the first step in using mechanistic modeling and simulation to inform regulatory decisions.7 The choice to be made using data from the computational model along with potentially additional sources of information is the question of interest. It is the particular issue, choice, or query that is being addressed. Neither the computational model nor the topic of the inquiry should be limited to the computational model. What effect, for example, does a low load challenge have on the purity of the product in the elution pool?
The questions of interest were focused on chromatography process parameters and their effect on yield and purity. Questions of interest with higher model influence and decision consequence had the highest risk scores. The model risk was assessed or decided by:
- the weight of the model in the totality of evidence for a given decision, i.e., model influence; and
- the potential consequences of a wrong decision, i.e., decision consequence.6
Model influence and decision consequence are shaped by the COU, thus enabling model risk to be case-specific. It is important to note that if the risk is high, there would be a higher threshold for evidence around the model’s credibility.
Model Validation
To proceed with model validation, four main areas were taken into account:
- Code verification — thoroughly checking the code to ensure it is correct and functional.
- Agreement with other modelsodel calibration results — analyzing results and making adjustments with real data for accuracy.
- Validation agreement — testing the model against controlled experiments to confirm it can predict outcomes correctly.4
Adequacy Assessment
It was determined that the model can be used for late-stage process development activities based on the evidence gathered through adequacy assessment. The evidence assessed fell into three categories, each with varying degrees of risk and credibility. Code verification and quality checks were performed to ensure that the simulation ran without any errors. Model calibration was conducted to examine how well the simulation aligns with experimental data, using metrics like peak shape agreement. Furthermore, model performance against untrained data was performed to check if the model can accurately predict outcomes even if it was not explicitly trained on it. Moreover, evidence such as peak shape agreement and retention time showed that the model can accurately replicate key features of the experimental data.4
The model was applied to perform risk assessments and support late-stage process development activities. The methods utilized were cause and effects (C&E) analysis and failure modes and effects analysis (FMEA).6
Cause And Effects Analysis
C&E is a method of scoring that determines the relationship between critical process parameters (CPPs) and critical quality attributes (CQAs). CPPs are considered crucial as they impact CQAs. These qualities significantly affect the quality and safety of a product, making it essential to analyze the parameters that affect them.
In the context of chromatography steps, parameters such as load challenge and elution length were analyzed by creating questions of interest to be scored. For the scoring criteria, three different scores were used: 1, 5, and 10.
- A score of 1 is assigned when a CPP has no effect on a CQA and a score of 5 is assigned when a CPP has a small effect that is not considered significant on a CQA
- A score of 10 is assigned when a CPP has a large and significant effect on a CQA. Three factors in each question were analyzed and the numerical scores of each factor were multiplied together to create a final score for the question.
Failure Modes And Effects Analysis
FMEA is a systematic methodology employed to identify failure modes for the CQAs and potential causes. Failure modes, in this context, describe deviations from the expected downstream process and the consequences that result as occurrences of these unexpected changes. FMEA allows for a comprehensive consideration and observation of failure modes that affect CQAs. Engaging in this type of analysis will help ensure that a quality product will be made each time by giving insight to create a consistently strong and effective control strategy. A question of interest was asked and given three different scores, with each answer being placed in one of three categories: severity, occurrence, and detection. Each one is sorted by five different potential numerical scores: 1, 3, 5, 7, and 9. All the scores have their own unique qualitative descriptions in each category.
- A severity score of 1 describes an instance where a failure mode has no effect on the parameter output. An occurrence score of 1 describes a failure mode event that is never observed. It also refers to an instance where a failure mode can be detected and corrected before the process is affected.
- A score of 3 describes when the process performance is somewhat affected but not a CQA. A score of 3 describes a rare event and scenario where the failure mode can be detected and fixed before the process is affected in most instances.
- A score of 5 is given when there is some effect to the CQA. A score of 5 describes a failure mode instance where it can be detected and fixed after it affects the process. It denotes an event that occurs occasionally.
- A score of 7 is given when there is potential serious risk of the CQA being compromised. It is usually assigned to frequent events and instances that are difficult to detect even after the process.
- A score of 9 is assigned when the failure mode compromises the CQA and the whole batch must be discarded. It refers to very frequent events and failure modes that can’t be noticed at all.
The three FMEA scores for severity, occurrence, and detection are multiplied together to create a final score.
Conclusion
Biopharmaceutical process development is greatly improved by leveraging mechanistic chromatographic modeling through process characterization studies. By executing a high-resolution HIC mechanistic model using GoSilico, accurate predictions and optimizations of chromatographic separations were achieved, improving efficiency and product consistency. It was noted that developing a robust framework for assessing the credibility of these models ensures their scientific reliability and regulatory compliance by establishing rigorous criteria and validation procedures.
Also, detailed risk assessments, including C&E analysis and FMEA, were performed to identify and mitigate potential risks in late-stage development, ensuring robustness and reliability. Integrating these cutting-edge modeling techniques through characterization and risk assessment was highlighted as leading to a deeper understanding and control of chromatographic processes, ultimately contributing to the development of safe and effective biopharmaceutical products.
References:
- Cytiva (n.d.) Mechanistic vs statistical models. Mechanistic vs statistical models | Cytiva (cytivalifesciences.com)
- Cytiva (2022) Hydrophobic interaction and reversed phase chromatography. Hydrophobic Interaction and Reversed Phase Chromatography, Principles and Methods (cytivalifesciences.com)
- Cytiva (n.d.) Chromatography mechanistic modeling. Chromatography mechanistic modeling | Cytiva (cytivalifesciences.com)
- Garcia, C. D., & Lee, M. R. (2021). Enhancing modeling skills through GoSilico mechanistic modeling. Modeling Techniques in Science, 7(2), 112-129.
- Mollerup, J. M. (2008). A Review of the Thermodynamics of Protein Association to Ligands, Protein Adsorption, and Adsorption Isotherms. Chem. Eng. Technol. 31, No. 6, 864-874. https://doi.org/10.1002/ceat.200800082
- Wilson, S. M., et al. (2016). Mitigating risks in modeling studies: Strategies and implementations. Risk Management Review, 3(2), 55-70
- U.S. FDA (2023) Assessing the Credibility of Computational Modeling and Simulation in Medical Device Submissions. https://www.fda.gov/media/154985/download
About The Author:
Emmanuel Mensah is a Master of Engineering candidate at the Keck Graduate Institute of Applied Life Sciences, Claremont, California, specializing in biopharmaceutical processing. He holds a B.S. degree in chemistry from the Kwame Nkrumah University of Science and Technology, Ghana. He has worked on collaborative projects with companies, including Pfizer, on late-stage modeling.