Hard Lessons In Parenteral Automatic Visual Inspection
By Jai A. Pathak, Ph.D., AstraZeneca
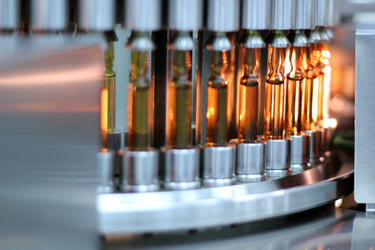
For all its maturity, automatic visual inspection (AVI) technology struggles with low fill volumes and often mistakes vial imperfections for contaminants when there’s more unfilled space inside.
Jai Pathak, AstraZeneca’s director of drug product technology, transfer, and validation, was part of the company’s effort to reduce the number of false rejects for vials containing a particular mAb product. The high-tech problem pulled in multiple contractors to investigate and concluded with a satisfyingly simple solution that cut the false rejection rates roughly in half.
Pathak presented the case study for the International Society for Pharmaceutical Engineering’s 2025 ISPE Aseptic Conference in March. We followed up with some questions about the inquest. Here’s what he told us.
Can you provide brief summaries of the case studies you talked about?
My talk focused on the case of two low-viscosity mAb biologic drug products (DPs) filled at low (1.5 mL) fill volume in 10R ISO vials. 2R ISO vials weren’t chosen because of supply chain constraints. Because of the low fill volume in a large vial, the static detectors (SDs) on the automatic inspection machine (AIM) were unable to detect particulates, as they are designed to.
Therefore, particulate detection was switched from SDs to vision cameras (VCs). Forcing highly sensitive VCs to detect particles was unavoidable but counterproductive, because they picked up flaws/defects in the glass vials in addition to particulates, and this led to increased vial false rejection rates.
A two-step inspection process, automatic followed by manual inspection of vials rejected during AVI, was followed. True defect rates on the order of ~1% were observed in these two mAb DPs.
How do different fill volumes and container formats affect false reject rates in AVI? What other specific challenges do low-fill-volume products present?
I discuss the interplay effects of fill volume in 10R vials and low viscosity later. Fill volume by itself is not as important as when viewed in perspective of the vial size. Recipe development for AVI should be done while carefully accounting for fill volume, product viscosity (vortex depth), and vial size. A low fill volume for a low viscosity fluid will yield a deeper vortex, and the deeper vortex will be more prominent and will approach the vial bottom, driving spinning fluid up the vial wall. Moreover, we discovered product defect kit vials and actual product vials to be inspected should be filled in vials from the same vial source site: nominally identical 10R vials manufactured at different sites can have slight differences in curvature in the neck region and also in thickness in the heel region.
While these small differences can’t be discerned by manual visual inspectors, sensitive machine VCs can detect them easily, and this detection leads to higher-than-acceptable false rejects due to the extreme sensitivity of VCs.
How did you identify whether the false rejects were due to AVI limitations, product characteristics, or container-related issues?
We used a combination of diagnostic visualization of the vials being inspected by SD detectors. We also inspected the incoming vials for scratches and monitored for the formation of scratches due to glass-to-glass contact on the fill line.
The visualization of the spinning vials in front of the SD showed exactly why they were ineffective in picking up particulates: the combination of the low fill volume and low viscosity led to the formation of a deep vortex of liquid in the vial center, and the liquid climbed up the wall of this vial, thus disappearing out of the field of view of the SD!
Incoming vials showed scratches due to suboptimal tertiary packaging of the filled vial cartons on the shipping pallet. Inserting a foam block on the pallets to arrest sliding of the cartons during shipment led to reduced scratches on vials. Inspection of vials showed low incidence of scratch formation on the fill line. Thus, ineffective packing was identified as the main determinant of scratch formation on incoming vials.
How do you see artificial intelligence improving issues like false rejects?
Artificial intelligence is a valuable computational resource/tool to train an AIM to recognize defects and to spot them when they occur. After using vials and syringes with legitimate defects to create image libraries, AI can be harnessed to compare tested product containers against the library. Images that correspond to true defects from within these libraries will lead to rejection of the product, thus minimizing false rejects and improving yields in the AVI process.
What best practices emerged from these case studies that you would recommend to peers?
For a successor mAb product, we proactively prepared for successful AVI. We initiated a four-way collaboration between us, the CMO’s DP/AVI teams, the AIM vendor, and the defect kit vendor.
We carved out a dedicated AVI workstream within the technology transfer team and created a cross-disciplinary team that was charged with the goal of successful AVI process development. The AIM vendor helped us de-risk inspection of this new product in 2R vials with bench-scale studies done at their site. The defect kit vendor developed representative product-specific defect kits, and they also brought a specialist engineer to the CMO site to tune and optimize the AIM and recipe.
The effect of this effort was a significant improvement of the AVI process of this product over the two previous ones: the true defect rate in the successor product was ~ 0.05%, much lower than the 1% seen with the precursor products.
Editor’s Note: Viewpoints expressed here are those of the expert, and do not represent the views of his employer.
About The Expert:
Jai A. Pathak, Ph.D., is a director at AstraZeneca and an active member of ISPE. He has spent much of his career in drug product process development. He received his B.S. in chemical engineering from the University of Rochester and his M.S. and Ph.D. in chemical engineering from Penn State. He completed postdoctoral research first at the National Institute of Standards and Technology and at the U.S. Naval Research Laboratory, and then started his bio-pharma career at MedImmune in Dec. 2008.