"From Panacea To Precision To Personalized Medicine": The Potential Of Computational Biology In Drug Development
By Life Science Connect Editorial Staff
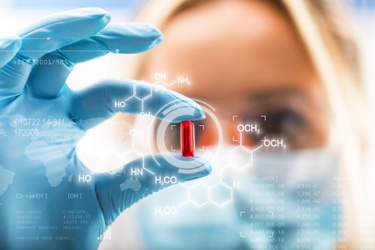
The next era of drug discovery and development will be defined by the power of computational biology. The question is, have we entered said era or are we still on our way? The answer depends on who you ask and where they work. EVQLV’s CEO and cofounder, Andrew Satz, and Recursion’s president and COO, Tina Marriott Larson, are both working at biotech companies that leverage computational biology to help discover new targeted drugs. On the recent Bioprocess Online Live event How Computational Biology Speeds Drug Discovery, Development, host and Chief Editor Matt Pillar caught up with Satz and Marriott Larson to glean greater insight into both EVQLV and Recursion’s ongoing initiatives.
Throughout the conversation, Satz and Marriott Larson touched on the convergence of bio and tech to spark major innovation in the drug discovery space. They also discussed industry anxiety around artificial intelligence (AI) and machine learning (ML), sharing their thoughts on whether AI will inevitably take our jobs. Though they both dismiss that possibility, they are confident that these technologies have the potential to make us all more effective at our jobs. In biopharma, the potential reverberations of AI and ML are profound, creating nearly infinite opportunities for drug discovery to become more tailored, specific, and beneficial for patients.
Why The Convergence Of Bio And Tech Is Vital
Though the rapid evolution and diversification of technology have long been seen as tools to improve the efficiency of how we work and live, the newest wave of AI and ML technologies is only just starting to impact biopharma’s approach to drug discovery. The application of computational data is now being used to identify novel targets and the molecules with the potential to impact them, whereas, historically, the process comprised a specific target and corresponding lab work. The speed and simplification possible with computation stands to improve the efficiency of drug development and increase the odds of getting drugs to patients as quickly as possible.
Marriott Larson describes the current biotech revolution as “industrializing drug discovery.” Through that process, Recursion and other biotechs are leaning on something called the flywheel effect, a tech phenomenon in which each cycle becomes more efficient and higher quality. These tech cycles lead to cost and discovery efficiencies over time. Previously, a scientist would have to dig through literature to identify any data relevant to a drug discovery program. Now, a language learning model (LLM) can quickly parse data for meaningful findings.
Though the potential of computational biology is exciting, the field is still extremely new, making it hard to accurately predict its trajectory. In the startup world, experts refer to two distinct stages: 0 to 1 and 1 to 10. During the 0 to 1 phase, a previously unmet need is met, while the 1 to 10 phase focuses on improvement, growth, and longevity. From Satz’s perspective, ML and AI are in the 1 to 10 phase: “Once we start seeing more AI-generated drugs come to market with costs being driven down, we're going to move from panacea to precision to personalized medicine. My hope is that we're shifting from a repeated process to something more specific and tailored. If we industrialize, we’ll have more medicines on the market. That’s the 0 to 1 switch.”
This isn’t Marriott Larson’s first biotech revolution. She joined Genentech in the mid-’90s and watched it grow from a small biotech working with recombinant DNA technology to develop biologics to a major player in the industry. In reflecting, she considers, “How long does it take a technology platform to change the world? It was about 25 years between the founding of Genentech and its tipping point. The earliest versions of technology are not the best versions. What we must look at is, are we on the right trajectory to keep getting better? By design, it takes a lot of time to get something to the clinic.”
The important thing to remember is that this movement is a collaboration between two industries: tech and biopharma. For it to truly work, all hands must be on deck. Per Satz, “Historically, we’ve taken a very scientific approach to this process and tested one thing [at a time]. Engineering tries to look at things holistically. AI and ML are powerful tools, but just tools. We need to use intuition, CRISPR, organs on a chip, and automation together to solve problems. When it works, we’ll be able to help a lot of people.”
How Recursion And EVQLV Are Using Computational Biology
Marriott Larson joined Recursion in 2018: “For me, it was like going back to the Genentech of the mid-’90s. I'm a biochemical engineer by training; I believe that technology can and will continue to advance medical science.” Recursion is building what’s described as a “Google Maps version of the human body,” and computational scientists are running the show. Recursion scientists begin with a cell context relevant to a disease and run every experiment in that cellular context, knock out every gene, and build a large data set for ML. From there, the data guides the Recursion team to different opportunities to treat the disease. According to Marriott Larson, “In the same way that we would use Google Maps to get from San Francisco to Seattle, we think about how we navigate these massive data sets to arrive at novel targets in biology. Once we find novel targets, how do we navigate to the molecules that impact those targets? These computational technologies have allowed us a different way of thinking about how we enter into the drug discovery process.”
For Satz, the choice to cofound EVQLV centered around two things: “The first was watching my mother suffer from a neurological disorder and feeling helpless for most of my life and not wanting others to experience that suffering. The second is my personal mission of bringing healing and freedom to others. In 2019, I looked at the massive growth of biological data, and it became clear that there is a way to add and improve on existing methods of discovery.” At EVQLV, they’re using cloud-based technology to copy how immune systems form antibodies to create human antibody sequences. The team is generating functional antibodies out of the gate without a need to conduct immunization or to make a recombinant protein to perform that immunization. It allows drug developers to skip over long, costly steps to get to a functional molecule.
When To DIY Vs. When To Outsource
Ultimately, whether you choose to DIY or outsource your computational biology projects depends on the scale of your goals and the questions you’re asking. Satz notes that, “There are four ways you can implement computation: build new models, [improve] existing models, generate data, or find new ways to use the data that you have.” Implementing a large-scale computational infrastructure is a major investment in time, people, and laboratories. At Recursion, they have built an infrastructure that conducts 2 million experiments every week. Their setup requires supercomputers and massive lab offerings to accommodate a primary cell line and infer relationships in silico. Still, they do some outsourcing. Per Marriott Larson, “It takes a village to bring any drug to market; we’re not building this on our own.”
That said, Recursion is taking a specific large-scale approach, which they knew from the start. Other industry players have smaller, more targeted projects that could benefit from outsourcing partnerships. EVQLV brings advanced tools to organizations that don’t have the capacity to build them in-house. By looking at efficient ways to reduce the antibody search space, EVQLV designed a platform that combines evolution, structure, and protein language models to generate target-specific antibody sequences. The platform builds and modifies antibodies while retaining confirmational and functional integrity and provides a shorter path to recombinant antibody expression and functional testing. It comprises a suite of learning modules designed to come up with antibody sequences while interrogating binding, affinity, specificity, and developability. By forming an outsourcing partnership with EVQLV, sponsors are able to leapfrog months of traditional trial and error.
A Marriage Of Two Different Worlds
The integration of AI into everyday life is a major pivot. Satz notes, “There's been a dropoff in skepticism over the past year. People who are not data scientists are able to interact with machine learning and see what's possible with chatGPT and other LLMs.” Both Satz and Marriott Larson note that organizations looking to merge biology and technology will need to focus more on the integration of two different cultures than anything else. This entails educating life science scientists, computational scientists, and all relevant stakeholders on how to understand each other’s languages. Satz encourages the recruitment of translators, or people knowledgeable in both biology and tech who can explain things to those who are unfamiliar with the other industry. By creating a culture of equanimity and collaboration across your biotech, you are well-suited to leverage the rapidly expanding benefits of AI technology to impact patients around the world.
To listen to the full Bioprocess Online Live event, click here.