FDA Seeks Input On AI Adoption In Drug Development And Manufacture
By John Giantsidis, president, and Maria Papademetris, regulatory policy analyst, CyberActa, Inc.
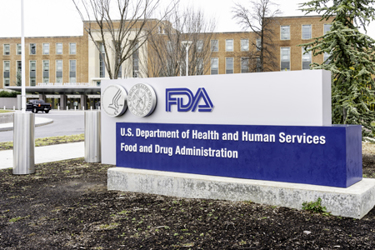
Artificial intelligence (AI) is the bedrock of our current digital revolution, just like the steam engine or electricity in the past. AI-based medical systems can be purely software-based, acting in the virtual world (e.g., image analysis software) or AI can be embedded in hardware devices (e.g., advanced surgical robotics or Internet of Medical Things applications). When combined with the utilization of data, these technologies enable, among other things, real-time value chains, new digital products and services, and new customer-centric business models. The U.S. FDA and other regulatory bodies have understood the incredible value that AI systems can offer in making predictions, recommendations, or decisions influencing real or virtual environments. Each AI system life cycle phase involves: i) design, data, and models, which is a context-dependent sequence encompassing planning and design, data collection, and processing, as well as model building; ii) verification and validation; iii) deployment; and iv) operation and monitoring.
The FDA, to begin an initial communication with stakeholders released two discussion papers for consideration: Using Artificial Intelligence and Machine Learning in the Development of Drug and Biological Products1 and Artificial Intelligence in Drug Manufacturing.2
Drug Development Discussion Paper Highlights
The FDA paper identified several current and potential areas for AI adoption:
1. Drug Discovery
- Drug target identification, selection, and prioritization
- Compound screening and design
2. Nonclinical Research
3. Clinical Research
- Recruitment
- Selection and stratification of trial participants
- Dose/dosing regimen optimization
- Adherence
- Retention
- Site selection
- Clinical trial data collection, management, and analysis
- Data collection
- Data management
- Data analysis
- Clinical endpoint assessment
4. Post-marketing Safety Surveillance
- Case processing
- Case evaluation
- Case submission
5. Advanced Pharmaceutical Manufacturing
- Optimization of process design
- Advanced process control
- Smart monitoring and maintenance
- Trend monitoring
Finally, the FDA acknowledges that AI has the potential to accelerate the current drug development process and make clinical trials safer and more efficient. The agency identified the key AI questions in drug development that would require further assistance from industry, academia, and other stakeholders.
Human-Led Governance, Accountability, and Transparency
- In what specific use cases or applications of AI/ML in drug development are there the greatest need for additional regulatory clarity?
- What are the main barriers and facilitators of transparency with AI/ML used during the drug development process (and in what context)?
- How are pre-specification activities managed, and changes captured and monitored, to ensure the safe and effective use of AI/ML in drug development?
Quality, Reliability, and Representativeness of Data
- What additional data considerations exist for AI/ML in the drug development process?
- What are some of the key practices utilized by stakeholders to help ensure data privacy and security?
- What processes are developers using for bias identification and management?
Model Development, Performance, Monitoring, and Validation
- What practices and documentation are being used to inform and record data source selection and inclusion or exclusion criteria?
- In what context of use are stakeholders addressing explainability, and how have you balanced considerations of performance and explainability?
- What are some examples of current tools, processes, approaches, and best practices being used by stakeholders for: selecting model types and algorithms for a given use, determining when to use specific approaches for validating models and measuring performance in a given context, evaluating transparency and explainability, and increasing model transparency, etc.?
Artificial Intelligence In Drug Manufacturing Discussion Paper Highlights
FDA’s risk-based regulatory framework would require adjustment to address the use of AI technologies in drug manufacturing considering the potential ambiguity of how to apply existing current good manufacturing practice (cGMP) regulations for NDAs, ANDAs, and biologics license applications (BLAs).
The discussion paper on drug manufacturing really showcases that the FDA is growing its own experience and expertise with AI technologies and FDA truly understands the use of AI technologies and how it should best be governed. An area requiring considerable regulatory oversight is cloud applications because they may affect oversight of pharmaceutical manufacturing data and records. The ongoing interactions between cloud applications and process controls could complicate the ability to establish data traceability, create potential cybersecurity vulnerabilities, and require evaluation of the procedures in place to monitor data integrity vulnerabilities during an inspection, and the FDA provided several “potentially associated requirements”3,4 to meet the following:
- How are data integrity and data quality ensured in these environments?
- What oversight is needed by the manufacturer based on existing quality agreements between the manufacturer and a third party (e.g., for cloud data management) with respect to managing the risks of AI in the context of manufacturing monitoring and control?
- During inspections, how is the manufacturer ensuring that the third party creates and updates AI software with appropriate safeguards for data safety and security?
The FDA is formally asking for input from industry and all other stakeholders on the following questions:
- What types of AI applications do you envision being used in pharmaceutical manufacturing?
- Are there additional aspects of the current regulatory framework that may affect the implementation of AI in drug manufacturing and should be considered by FDA?
- Would guidance in the area of AI in drug manufacturing be beneficial? If so, what aspects of AI technology should be considered?
- What are the necessary elements for a manufacturer to implement AI-based models in a cGMP environment?
- What are common practices for validating and maintaining self-learning AI models and what steps need to be considered to establish best practices?
- What are the necessary mechanisms for managing the data used to generate AI models in pharmaceutical manufacturing?
- Are there other aspects of implementing models (including AI-based models) for pharmaceutical manufacturing where further guidance would be helpful?
- Are there aspects of the application of AI in pharmaceutical manufacturing not covered in this document that FDA should consider?
Conclusion
These discussion papers foretell FDA’s approach to regulating AI/ML in drug development, including the development of medical devices for use with drugs. The FDA has shown incredible commitment to the adoption of AI/ML and now is soliciting feedback on the associated opportunities and challenges. Interested stakeholders can submit comments for FDA’s consideration on the discussion paper on drug development until Aug. 9, 2023, to Docket No. FDA-2023-N-0743, available at https://www.regulations.gov/docket/FDA-2023-N-0743. Interested stakeholders wishing to present ideas and concerns for consideration and potential policy development on the application of the existing regulatory framework to use of AI in pharmaceutical manufacturing can submit comments to Docket No. FDA-2023-N-0487 (deadline is unclear but we are guessing it might have the same deadline of Aug. 9, 2023).
References
- https://www.fda.gov/media/167973/download
- https://www.fda.gov/media/165743/download
- 21 CFR 11, 211.180, 211.184, 211.194, 600.12
- ICH Q7 Good Manufacturing Practice Guidance for Active Pharmaceutical Ingredients (September 2016)
About The Authors:
John Giantsidis is the president of CyberActa, Inc. and partners with innovators to develop and execute regulatory commercialization, privacy, cybersecurity, and data risk solutions that help them compete in global markets. He can be reached at john.giantsidis@cyberacta.com.
Maria Papademetris is a regulatory policy analyst with CyberActa, Inc., a boutique consultancy empowering medical device, digital health, and pharmaceutical companies in their cybersecurity, privacy, data integrity, risk, AI/ML regulatory compliance, and commercialization endeavors. She can be reached at maria.papademetris@cyberacta.com.