Expanding RNA-Based Immune Profiling to Immune State Makes Precision Medicine More Precise
By Jarret Glasscock, Ph.D. CEO, Cofactor Genomics
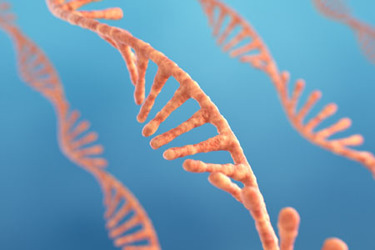
The race to cure cancer has focused on drug discovery; in the last five years, clinical trials increased 500% for new drugs. Genome targeted therapies were an early source of excitement, but only an estimated 5% of patients benefit. More recently, immune checkpoint inhibitors offered a completely new class of therapeutic with breakthrough results, but even these transformational therapies work in less than 25% of patients. Low response rate, high cost of care, and potentially serious toxicity make it essential to separate potential responders from non-respondents.
In this new era of precision medicine, dependency on legacy diagnostics that are built on single-analyte biomarkers fail to give physicians enough information to treat most patients effectively. That’s because the immune response is not a single cell acting in isolation. The immune response to cancer is a combination of several immune cell types: CD4s promote CD8 activity and inhibit Treg, Treg inhibits CD8, and so on. Single DNA mutations, single RNA fusions, and even single immune cell types are neither sufficient nor reliable biomarkers, and ultimately leave us with poor outcomes. In fact, 75% of cancer patients are given drugs that ultimately don’t benefit them, and in some cases, may actually harm them.
In response, the field of diagnostics dramatically expanded over the last ten years, offering tools that can be useful well beyond the initial disease diagnosis. Predictive diagnostics match patients with therapies by employing advanced molecular and computational approaches. Multidimensional biomarkers using RNA sequencing combined with machine learning reveal a much more complete picture of cancer. For years, scientists have been looking at RNA, but only recently gained better technology and a better understanding of how to work with this valuable molecule.
RNA Vs. DNA
There are several advantages of RNA over DNA as a biomolecule. RNA is labile, changing in both sequence and abundance in response to genetic and epigenetic changes. RNA also changes in response to external factors like disease, therapy, exercise, and more. In contrast, DNA is generally static and changes very little, and a detected DNA mutation does not necessarily result in disease. The body compensates, repairs, and overcomes DNA mutation through redundancies. By evaluating RNA, we get closer to understanding the true impact of disease on the body.
This next generation of predictive diagnostics is being built using high-throughput RNA sequencing and computational tools, including machine learning and AI. Multidimensional models of genes measure the dynamic, interconnected expression levels beyond the presence or absence of a single, isolated transcript. The development of models is not that far off from gene expression classifiers and immune profiling. However, early research indicates that making use of machine learning for model generation significantly increases the predictive power of the resulting biomarker. The ever-increasing depth of sequencing we can now achieve, paired with the plummeting costs of it, allow us to finally leverage all the benefits and unique qualities that are encoded and buried deep in the rich data represented by RNA.
When we consider the landscape of predictive diagnostics, the evolution of technologies that have made an impact in clinical practice follows a path that begins with simplicity and adds additional context. In the beginning, diagnostics were focused on making predictions based on detecting mutations - often through a large DNA panel - by homing in on one or two key genes to make a clinical decision. Recognizing that more signals were required to capture the complex biology of cancer, the field of multianalyte oncology diagnostics was born. With the advent of immune-oncology, we can now use a new approach to characterize the tumor microenvironment and better understand the role that the immune system plays in disease progression and therapy response. In other words, multidimensional biomarkers improve our ability to predict a patient’s response to treatment.
Predictive diagnostics provide confidence in therapy selection by enriching for responders. The KEYNOTE-048 study attempted to provide more confidence in therapy selection by enriching for responders to IO monotherapy using a higher threshold (≥20) for the on-label PD-L1 IHC test, a single-analyte biomarker. Yet, the highest PD-L1 score threshold is still only a modest improvement upon response rates one might expect without using the test at all. The power of the multidimensional approach comes from the added consideration of a combination of multiple cell types and signals. It is not driven by one individual cell type. The additional analytes, combined with machine learning, capture the most complete information yet about a patient’s disease and give physicians the best chance to treat cancer with therapies that will actually work.
The industry has been working to characterize the immune component of the tumor microenvironment more fully, and in this characterization, we squeeze out more information and better prediction of which subset of patients will ultimately respond to powerful new immunotherapies. Measuring presence and abundance of various immune cells in the tumor microenvironment has been a particular focus in immune profiling efforts to date but is not enough. Moving beyond PD-L1 measurement to quantify T-cell, B-cell, macrophage, monocyte, and beyond has been the goal. Multi-dimensional RNA approaches are at the center of much of this work.
Immune profiling is now able to move beyond immune cell identification and quantification to include immune cell state, a progressively interesting and important consideration in the context of patient response to immunotherapy treatment. Historically, approaches such as immune cell localization or viral infection status have been used to infer state of immune activity. For example, immune cells held at the periphery of the tumor may not be activated, or at least not be effective, and a viral infection like HPV may provide additional information that influences both prognostic and predictive factors in the context of immunotherapy. RNA expression is now being used to move the field from qualitative to quantitative profiling of immune cell states, initially in single gene form, and more recently, in more robust multi-analyte approaches.
This exciting new concept has been captured in recent work published in Nature Scientific Reviews that investigated how RNA-based techniques to measure T-cell state are powering diagnostic approaches that predict which patients will benefit from immunotherapies such as immune checkpoint inhibitors. The study showed that multianalyte biomarkers based on T cell subtype profiling predicted patient response to anti-PD-1 therapy in three different cancers (lung cancer, melanoma, and head and neck) and outperformed the indicated PD-L1 test and Tumor Mutational Burden. Studies like these illustrate that more granular characterization of immune cells can, in fact, increase the predictive power of biomarkers.
In 21st century precision medicine, there are an increasing number of immunotherapies shown to work in subsets of the population. This new class of immunotherapies has durable and lasting results for certain patients, but currently available diagnostic tests are not sufficient to identify a breadth of patients who will benefit. Using new technologies in predictive diagnostics, physicians can now determine whether a patient’s tumor has an immune profile that will fit a treatment path that includes monotherapy and avoids the toxicities of treatments like chemotherapy. This new chapter of diagnostics, focused on characterizing immune activity, provides greater detail and better information to physicians by not only measuring immune analytes, but also identifying the cell state. This more complete model of the tumor biology is showing promise to become a new standard for predicting patient response and helping more patients benefit from the power of immunotherapy.