Embracing An Economic Model For Agile Process Development
By Irwin Hirsh, Q-Specialists AB
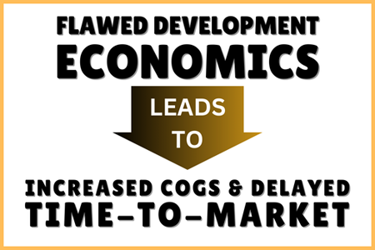
Pharmaceutical process development teams face a growing challenge in prioritizing and managing their tasks effectively as they work to bring innovative drugs to market. The introduction of AI-driven lead discovery could trigger a surge in the volume and quality of new medical entities (NMEs) entering the development pipeline, thus amplifying existing bottlenecks and inefficiencies in process development, highlighting a critical need for change.
Traditionally, the industry has focused its process development efforts primarily on supplying clinical trials, often at the expense of developing a robust, market-ready manufacturing process. As a result, pharmaceutical companies find themselves at risk for or even grappling with delayed process optimization, higher production costs, and regulatory challenges — all of which can delay the market launch of potentially lifesaving drugs. To address these issues, process development requires a new economic model that integrates clinical risk and lifetime product value and enables a proactive, real-time decision-making approach.
Figure 1 How Development Delays Matter
The development of a manufacturing process, if not well designed, documented or communicated, can cause delays in launch by delaying the process validation and/or the ability to manufacture at scale at the final site of production. Cost of Goods Sold (COGS) are also, to a large extent, determined by design choices and the quality of the documentation that gets carried into the on-market process. Therefore, we need to not only consider manufacturing process development as essential to supply clinical trials but as a value stream that has the potential to impact the lifetime value of a product in significant ways. This is why an economic model that includes impact on product lifetime value, as well as a phase-based risk of clinical failure for the entity, will add great value to decision making process.
The Problem: Delays, Bottlenecks, And Knowledge Gaps
While critical to drug manufacturing, process development teams often operate in a reactive mode, waiting for clinical trial outcomes to inform their work. This clinical dependency results in a backlog of projects that require immediate attention upon trial success, creating significant bottlenecks. With the traditional waterfall approach to project management, decision-making is often centralized and delayed, relying on periodic scientific review boards to guide priorities. By the time decisions are made, data can become outdated, leading to suboptimal results and further delays. This rigidity impacts two crucial areas of drug life cycle value: cost of goods sold (COGS) and time to market.
COGS And Manufacturing Efficiency
The cost of goods sold, a critical factor in drug pricing, is highly dependent on early design choices within the manufacturing process. For example, a drug that undergoes multiple unoptimized processes may incur unnecessary costs in raw materials, labor, and equipment, leading to a less competitive product. According to industry data,1 manufacturing costs alone can constitute up to 17% of a biopharmaceutical company’s R&D expenditures per market success. By embedding economic considerations into the design phase, process development teams could identify opportunities to reduce COGS by optimizing efficiency and minimizing waste.
Example calculation
Consider a biologic drug with a projected annual production cost of $200 million, primarily due to process inefficiencies. By optimizing upstream and downstream steps, process development could potentially reduce this cost by 10%-15%, saving $20 million to $30 million annually. Over a typical 10-year product life cycle, these savings could translate into $200 million to $300 million, significantly impacting the product’s profitability and pricing strategy.
Figure 2 Prioritization Considerations for the Model
Decisions must be made in the light of one’s own business realities. A company building its own site must prioritize differently than a company utilizing a CMO or even existing facilities.
If your model is useful, it will also allow you to quickly and with consensus make decisions about trade-offs between:
- COGS and time to market
- in-house and outsourced manufacturing
- India vs. EU
All of these decisions will help the process development department know how and why it must prioritize which projects and experiments and complete a virtuous circle.
Time To Market And Knowledge Management
Poor knowledge management can slow down regulatory approval and delay time to market, reducing both profitability and patient access to new treatments. Weak documentation and communication often hinder critical steps like process validation and technical transfers to manufacturing sites, leading to missed production slots or failed batches. A delayed launch, even by a few months, can mean lost market share and millions of dollars in revenue. In "blockbuster" therapeutic areas, for example, a six-month delay might cost a company over $500 million in lost sales. These losses affect not only the business but also the patients awaiting treatment. For companies without blockbuster drugs, revenue shortfalls from delays can still severely harm financial stability. Even worse, the shortfalls may be compounded if delays enable competitors to come to market first.
A Vision For A New Economic Model: Informed, Real-Time Decision-Making
To address these issues, I propose we make efforts toward a process development economic model that accounts for clinical risk levels, failure probabilities, and the impact of delayed process knowledge on product lifetime value. This model should be dynamic, live, and accessible to bench scientists, allowing for frequent updates that reflect the company’s evolving economic landscape as well as data from clinical trials.
Key Features Of The Model
- Integrating Product Lifetime Value with Stage-Specific Risks
Currently, late-phase candidates will warrant accelerated development based solely on the improved risk of failure profile by having made it so far in clinical trials. While this is very appropriate, I believe we must also create a better understanding of the consequences of not investing earlier in development of the process. Do we even know if we are increasing the risks of late-stage failure or an unacceptable loss in product lifetime value when we decide to delay work on early-stage entities?
Figure 3 Development Delays Will Compound
- Real-Time Access for Bench Scientists
By making the economic model available as part of the decision-making tool at the bench scientist level, it allows process teams to prioritize experiments based on a real-time understanding of the business. For instance, a bench scientist could immediately prioritize tasks with the highest value impact, perhaps as an embedded feature inside their electronic laboratory notebook.
The Role Of Queuing Theory In Process Development
Moving away from a waterfall approach to decision-making, we can leverage queuing theory principles to optimize task flow and maximize resource utilization. Queuing theory, a mathematical study of waiting lines, allows for prioritizing the “weighted shortest task” — balancing urgency, resource availability, and potential value impact. This model breaks the rigidity of batching tasks in the waterfall model, reducing TAKT time and improving throughput.
Figure 4 Where the Business Suffers Due to Delays
Example application
In a queuing model, smaller, high-value tasks can take precedence, reducing wait times for critical path activities. Let’s say two tasks are waiting to proceed: Task A, requiring 2 hours and having a high-value impact, and Task B, requiring 10 hours with a lower impact. According to queuing theory, prioritizing Task A would maximize output for available time and resources, freeing up capacity for other critical tasks. The result is a streamlined, responsive approach that can bring decision times down from weeks to hours.
Implementing AI Modeling To Address Potential Bottlenecks
As AI-driven lead discovery accelerates the entry of NMEs into the pipeline, traditional process development methods will struggle to keep pace. Here, AI modeling of processes or specific process steps provides a cost-effective solution. By conducting in silico (computer-simulated) experiments, companies can explore numerous variables and outcomes without the resource-intensive requirements of wet chemistry.
Case in point: in silico process simulation
Let’s say a process development team needs to assess 15 different conditions for an NME’s purification step. Conducting these tests physically might take weeks, cost thousands of dollars, and require extensive lab resources. An AI-driven in silico model, however, could simulate these conditions within hours, allowing teams to focus only on the most promising configurations. This approach not only reduces capital expenditures but also enables process development to respond swiftly to the influx of new candidates.
Enhancing Experimentation And Reducing Workload
AI modeling allows process development teams to simulate thousands of experimental conditions rapidly, identifying the most promising configurations for validation. For instance, optimizing cell growth conditions for an NME might typically involve testing various combinations of nutrient concentrations, feed rates, and oxygen transfer levels in the lab, a process that could take weeks. With AI, these experiments can be simulated in silico within hours.
Case in pointA process development team evaluating 120 nutrient configurations for an upstream process identified the top five optimal setups using AI modeling. Laboratory experiments validated these predictions, achieving a 25% improvement in viable cell density and reducing experimental workload by 40%. |
Improving Predictability In Scale-Up
Scaling bioprocesses from bench to production volumes is a common bottleneck, often due to unanticipated changes in nutrient distribution, oxygen transfer, or shear forces. AI-driven digital twins can simulate these conditions across scales, predicting potential risks and guiding adjustments to critical process parameters (CPPs).
Case in pointDuring the scale-up of a 5-L bioreactor process to 200 L, AI simulations flagged nutrient limitations and oxygen transfer inefficiencies. Adjustments to agitation and sparging rates based on these insights enabled the scale-up to succeed in just three runs, compared to the typical six. This saved time and materials and ensured consistent product quality. |
Streamlining Workflow Integration
AI tools can analyze historical bioreactor data to uncover inefficiencies and guide real-time adjustments, enhancing the overall workflow. Additionally, by integrating these tools into platforms like electronic laboratory notebooks (ELNs), teams gain direct access to actionable recommendations.
Case in pointA digitalization team using AI to analyze historical production data discovered that suboptimal temperature control was contributing to glycosylation variability. By adjusting the operating temperature range, they reduced variability by 20%, improving batch-to-batch consistency. |
Supporting Regulatory Preparedness And Knowledge Management
Regulatory submissions often require detailed documentation demonstrating process stability and reproducibility. AI tools can automate the creation of these reports while ensuring alignment with regulatory guidelines such as ICH Q8-Q10.
Case in pointAI-generated reports detailing process stability across multiple CPP ranges were included in a regulatory submission for a Phase 3 candidate. The streamlined and comprehensive nature of these reports reduced the frequency of regulatory queries, enabling faster approvals. |
Enabling Real-Time Scenario Analysis
The ability to model and predict process outcomes in real time allows teams to proactively address potential disruptions. For example, changes in raw material specifications, new facility constraints, or unexpected parameter shifts can all be evaluated quickly through AI modeling.
Case in pointWhen a raw material supplier modified the specifications of a key input, AI models simulated the impact on the process and recommended adjusted feed rates. These changes were implemented seamlessly, avoiding costly delays or production inconsistencies. |
Accelerating Technology Transfers And Validation
AI modeling simplifies the tech transfer process by simulating how processes will perform in new facilities with different equipment or configurations. These simulations provide actionable insights to adjust for variables like impeller design or vessel geometry before physical validation begins.
Case in pointDuring a tech transfer to a CMO, AI simulations predicted differences in shear forces due to a change in impeller design. By preemptively adjusting agitation parameters, the process was successfully validated on the first attempt, reducing the tech transfer timeline by 20%. |
By incorporating AI modeling into process development, biopharmaceutical companies can address bottlenecks, reduce time to market, and optimize resource utilization. These innovations not only improve operational efficiency but also ensure that new therapies can reach patients faster while maintaining the highest standards of quality.
Building Long-Term Value By Optimizing COGS, Speed, And Product Quality
A robust economic model that integrates real-time data, AI-driven insights, and queuing theory principles can serve as a competitive differentiator in the biopharmaceutical industry. By optimizing COGS, reducing time to market, and enhancing product quality, companies can maximize the lifetime value of each NME.
For instance, a real-time economic model can help identify a 15% improvement in process efficiency, translating to a 10% reduction in COGS. In combination with AI and in silico modeling, these optimizations allow teams to accelerate process validation, tech transfers, and regulatory compliance, supporting a faster, more reliable path to market.
Example: impact on product lifecycle value
Consider a high-value NME with projected annual sales of $1 billion. By reducing COGS by 10% and accelerating time to market by six months, process development could add an estimated $150 million in early revenue, plus ongoing annual savings. Such improvements not only enhance profitability but also strengthen a drug’s competitive positioning, particularly in markets with narrow therapeutic windows.
A Call For Change
The biopharmaceutical industry stands at a critical juncture. The traditional focus on clinical trial supply has hindered process development’s ability to contribute effectively to final manufacturing and lifetime value. By adopting a live, real-time economic model and applying principles from queuing theory, pharmaceutical companies can transform their process development strategies.
The integration of in silico modeling and on-demand, data-driven decision-making enables companies to handle the increasing volume of AI-discovered NMEs more efficiently. This shift from waterfall to agile process management can accelerate market readiness, improve product quality, and reduce costs, ultimately benefiting both patients and the industry as a whole.
In this evolving landscape, a proactive, economically informed approach to process development will ensure that NMEs not only reach the market faster but also achieve lasting value and accessibility.
Reference:
- https://doi.org/10.1080/19420862.2020.1754999 S Farid et. al (2020) Benchmarking biopharmaceutical process development and manufacturing cost contributions to R&D. mAbs12(1).
About The Author:
Irwin Hirsh has nearly 30 years of pharma experience with a background in CMC encompassing discovery, development, manufacturing, quality systems, QRM, and process validation. In 2008, Irwin joined Novo Nordisk, focusing on quality roles and spearheading initiatives related to QRM and life cycle approaches to validation. Subsequently, he transitioned to the Merck (DE) Healthcare division, where he held director roles within the biosimilars and biopharma business units. In 2018, he became a consultant concentrating on enhancing business efficiency and effectiveness. His primary focus involves building process-oriented systems within CMC and quality departments along with implementing digital tools for knowledge management and sharing