3 AI Breakthroughs Revolutionizing Pharma Tech Ops At Roche
By Yiming Peng and Margaux Penwarden, Roche
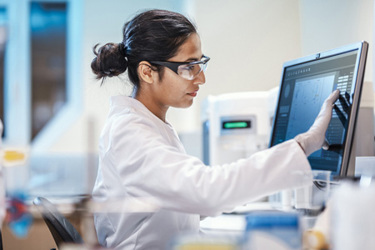
Artificial intelligence and machine learning (AI/ML) are revolutionizing pharmaceutical manufacturing, driving major benefits like higher production yields, reduced process variability, and minimized quality risks.
While most AI proof of concepts, particularly in generative AI (GenAI), get stuck in pilot purgatory, Roche has already scaled AI deployments, delivering clear time and cost savings by turning complex insights into actionable results.
We’ll be diving into these innovations at the 2024 International Society of Pharmaceutical Engineering (ISPE) Annual Meeting & Expo, where we’ll showcase real-world case studies. This preview offers a glimpse into the challenges we tackled, the AI-driven solutions we implemented, and the measurable impact we've achieved.
1. Driving End-to-End Process Performance And Product Health Through Predictive Modeling
Problem statement
Biologics manufacturing is a complex process, with living cells producing therapeutic proteins — where maintaining product quality and optimizing yields are constant hurdles. Variability, often driven by elusive root causes, adds to the challenge. At Roche, we’re harnessing the power of AI to unlock insights from vast, complex data, transforming our ability to enhance product yield and quality and ultimately delivering life-changing therapies to patients more efficiently.
Solution description
Roche’s AI-powered solution introduces predictive apps for titer/yield and critical quality attributes (CQAs), equipping operators to make smarter, faster decisions. This advanced technology:
- Predicts trends and identifies key factors driving variability.
- Optimizes process settings and batch sequencing for maximum efficiency.
- Leverages AI to proactively detect and address potential deviations related to CQAs.
With this solution, we’re redefining production processes and unlocking new levels of efficiency in drug manufacturing.
Overall impact
This solution has driven significant improvements, including:
- 5% to 10% boost in yields and enhanced target product quality in biologics manufacturing.
- Up to 50% reduction in CQA write-offs, significantly minimizing losses.
- Deeper process insights that fuel innovative product development for the future.
These achievements showcase our commitment to excellence in biologics manufacturing.
2. Harnessing AI To Optimize Manufacturing Scheduling And Overcome Capacity Constraints
Problem statement
Automating granular scheduling for manufacturing production plans is a complex endeavor, influenced by various factors like operator shifts, variable process durations, and both planned and unplanned events. The unpredictability of these events requires rapid responses, making automated AI integration essential for enhancing flexibility and responsiveness. At Roche, we’re leveraging AI-driven optimization to transform scheduling efficiency, overcome capacity constraints, and significantly increase production capacity — all while reducing costs. This approach not only streamlines operations but also enhances our manufacturing agility and effectiveness.
Solution description
Roche has developed an AI-driven solution utilizing genetic algorithms to optimize product sequencing and enhance asset utilization during planned downtimes. The application suggests maintenance and testing intervals while adjusting existing schedules in response to unplanned events. It also provides risk assessments, including success probabilities, enabling users to make informed decisions. Implemented across Roche’s global network, this approach has resulted in productivity gains of 5% to 10% on constrained assets.
Overall impact
- Achieved 5% to 10% capacity gains on constrained assets, enabling enhanced operational efficiency.
- Experienced a more agile response to unplanned events, ensuring greater resilience in production processes.
3. Mitigating Deviations Through A Generative AI-Driven Quality Assistant
Problem statement
Deviations — unplanned events that disrupt established protocols, standards, or expectations in pharmaceutical manufacturing — can arise at any moment, posing risks to product quality, safety, and compliance. These disruptions often lead to supply delays and inflated costs.
To tackle these challenges head-on, it is essential to identify trends and recurring deviations, unlocking insights into underlying issues such as problem statements, root causes, and effective remediation strategies. Roche is leveraging state-of-the-art generative AI-powered tools for deviation management, enabling swift and effective responses to current challenges while significantly reducing the likelihood of future occurrences. This proactive approach not only enhances operational resilience but also safeguards our commitment to quality and compliance.
Solution description
Recent advancements in GenAI have transformed Roche Pharma’s approach to manufacturing deviation management through the innovative use of retrieval augmented generation (RAG). RAG combines traditional information retrieval methods with generative AI to enhance the search process, allowing users to quickly access relevant data and context.
This cutting-edge technology empowers users to swiftly analyze historically similar deviations, enabling them to tackle current challenges more effectively. By capitalizing on the power of prompt engineering and RAG, we are not just responding to deviations, we are proactively minimizing their recurrence and driving a culture of continuous improvement in our operations.
Overall impact
- Eliminate labor-intensive manual reviews and investigations, achieving an estimated time savings of 30% to 50%.
- Leverage advanced analytics to enable intelligent deviation management, accelerating the closure of similar issues and driving operational efficiency.
- Shift from reactive to proactive management by identifying potential merging issues early, allowing for timely interventions and continuous improvement.
About The Authors:
Yiming Peng is the global head of statistics and data science for Roche Pharma Technical Operations. He and his nonclinical biostatistics team make extensive contributions to process development and drug manufacturing. His areas of expertise include statistical process control, design of experiments, data analytics, machine learning, AI, statistical consulting and collaboration. He is an active member of ISPE and the American Statistical Association (ASA). Prior to joining Roche/Genentech, he worked at Novartis and specialized in assay development and companion diagnostics. He graduated from Virginia Tech with a Ph.D. in statistics.
Margaux Penwarden is global data analytics senior director at Roche and comes with over 10 years of experience in applied data science, leading 40-plus analytics projects across various industries, with a focus on pharmaceuticals. Her work has helped key pharma sites gain recognition by the World Economic Forum and contributed to a Guinness World Record for Formula E. She focuses on scaling advanced analytics, ensuring that data-driven insights can be applied broadly across organizations, beyond technical teams, to drive impactful change. She is an active member of ISPE, holds an M.Sc. in computer science and applied mathematics from Telecom Paristech, and holds an M.Sc. in statistics from Imperial College London.